Deep sparse autoencoder integrated with three-stage framework for glaucoma diagnosis
INTERNATIONAL JOURNAL OF INTELLIGENT SYSTEMS(2022)
摘要
Recently, end-to-end deep neural networks-based glaucoma diagnosis approaches have been gaining much attention. However, the feature extractor and classier in these approaches are trained together, which is known as coadaptation. Therefore, the feature distribution in them should adapt to particular decision boundaries. To learn generic data representations and improve the generalization ability of the model, this paper designs a three-stage framework for glaucoma diagnosis. In the first stage, preprocessing is utilized to extract the Region of Interesting around the Optic Disc to reduce the computational cost and nonobjective interference. In the second stage, Deep Sparse Autoencoder is designed to learn hybrid features between the deep features and the original features, which could improve the effectiveness of final high-level feature expression. Meanwhile, L1 regularization is introduced and applied on the hybrid features to obtain deep features with high complementarity under small sample problem. In the third stage, the obtained generic feature representations are fed into different classifiers, in which Support Vector Machine classifier achieves the best diagnosis performance. The proposed approach is evaluated on two publicly available databases. Extensive experimental results indicate that our approach outperforms the state-of-the-art approaches with the accuracy of 96.00%, 97.00% and Area Under Curve of 96.94%, 98.28% for REFUGE and Drishti-GS1 databases, respectively.
更多查看译文
关键词
deep sparse autoencoder, glaucoma diagnosis, hybrid features, SVM
AI 理解论文
溯源树
样例
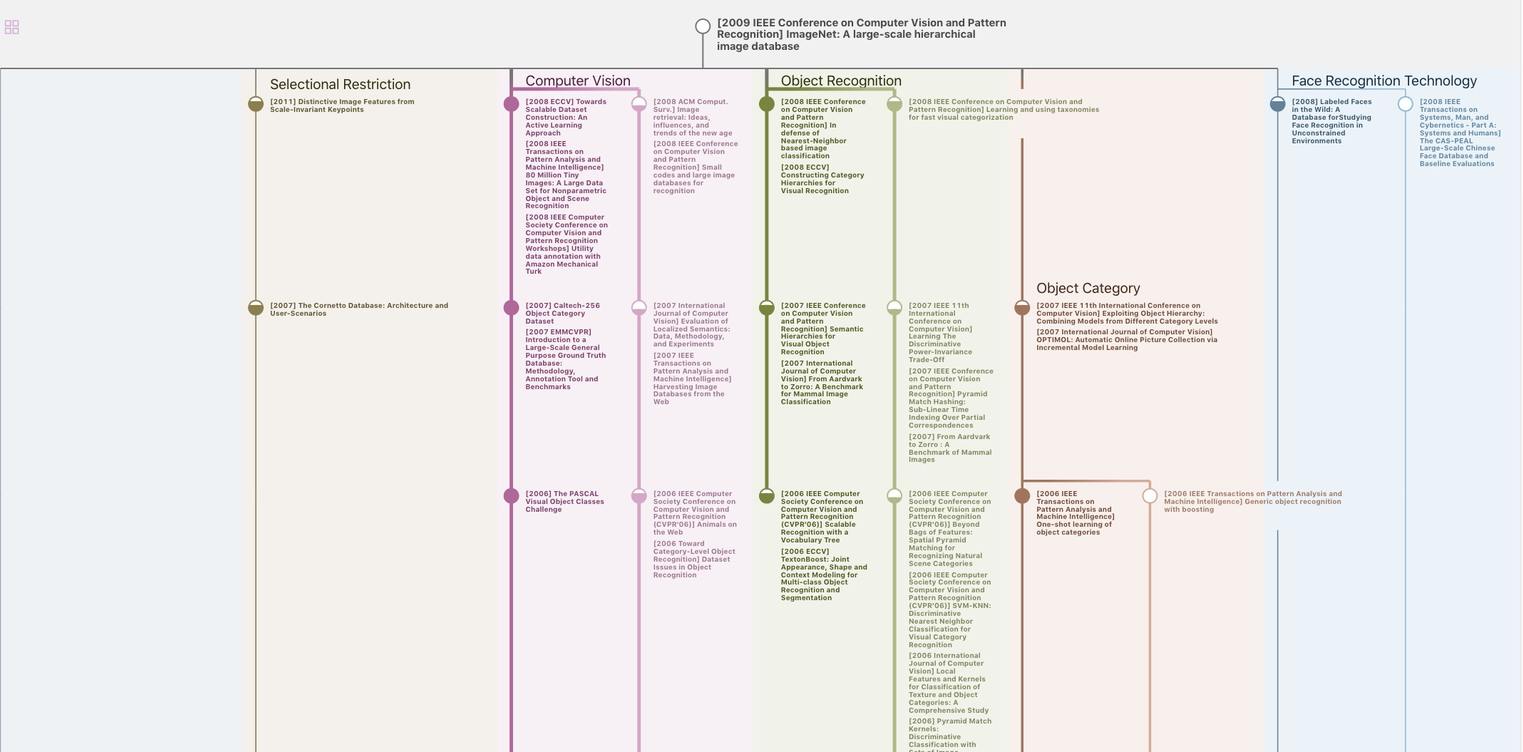
生成溯源树,研究论文发展脉络
Chat Paper
正在生成论文摘要