Inconsistent Ranking Assumptions in Medical Search and Their Downstream Consequences
PROCEEDINGS OF THE 45TH INTERNATIONAL ACM SIGIR CONFERENCE ON RESEARCH AND DEVELOPMENT IN INFORMATION RETRIEVAL (SIGIR '22)(2022)
摘要
Given a query, neural retrieval models predict point estimates of relevance for each document; however, a significant drawback of relying solely on point estimates is that they contain no indication of the model's confidence in its predictions. Despite this lack of information, downstream methods such as reranking, cutoff prediction, and none-of-the-above classification are still able to learn effective functions to accomplish their respective tasks. Unfortunately, these downstream methods can suffer poor performance when the initial ranking model loses confidence in its score predictions. This becomes increasingly important in high-stakes settings, such as medical searches that can influence health decision making. Recent work has resolved this lack of information by introducing Bayesian uncertainty to capture the possible distribution of a document score. This paper presents the use of this uncertainty information as an indicator of how well downstream methods will function over a ranklist. We highlight a significant bias against certain disease-related queries within the posterior distribution of a neural model, and show that this bias in a model's predictive distribution propagates to downstream methods. Finally, we introduce a multi-distribution uncertainty metric, confidence decay, as a valid way of partially identifying these failure cases in an offline setting without the need of any user feedback.
更多查看译文
关键词
information retrieval, uncertainty, bias, fairness, medical search, bayesian
AI 理解论文
溯源树
样例
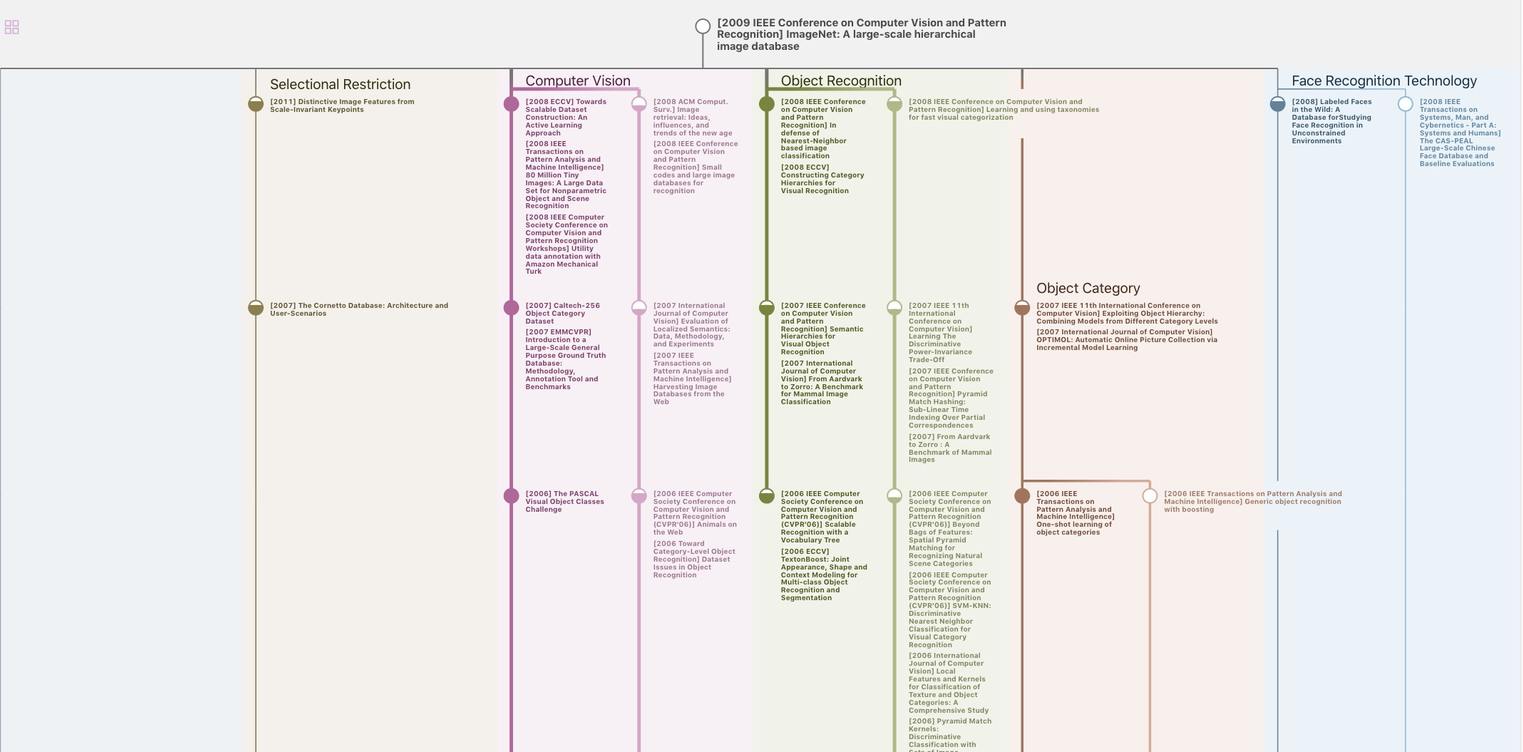
生成溯源树,研究论文发展脉络
Chat Paper
正在生成论文摘要