Automated vehicle inspection model using a deep learning approach
Journal of Ambient Intelligence and Humanized Computing(2022)
摘要
Image-based inspection is a growing area with a large scope of automation. The automatic classification of vehicle damages would make the insurance claim much faster and more efficient. This can effectively reduce the claiming cost. This paper presents, an image classification model using an adapted version of pre-trained convolutional neural networks. The pre-trained neural networks were, the VGG-19 and DenseNet-169. The proposed model is a pipeline that established with fully connected layers for additional damage classification. The final proposed model improves the feature extraction process. The dataset had a class imbalance problem, so a weighted loss function had been used to solve such problem. The model employed binary cross-entropy as a loss function, and sigmoid activation was applied to the output layers as independent layers. Finally, the model presents a multi-label classifier, where one image may be assigned to many labels. The model classifies vehicle damage through five classes: broken glass, broken headlights, broken taillights, scratches, and dents. A four-layer neural network was employed for the classification, along with several regularization approaches to handle overfitting problem. The final results showed that the DenseNet-169 had a better accuracy of 81%, whereas VGG-19 had a 78%. Another approach had been proposed where it had a mix of transfer and ensemble learning approaches. This final approach had an accuracy of 85.5% and F1-scores of 0.855.
更多查看译文
关键词
Deep learning,Computer vision,Deep convolutional neural networks,Transfer learning
AI 理解论文
溯源树
样例
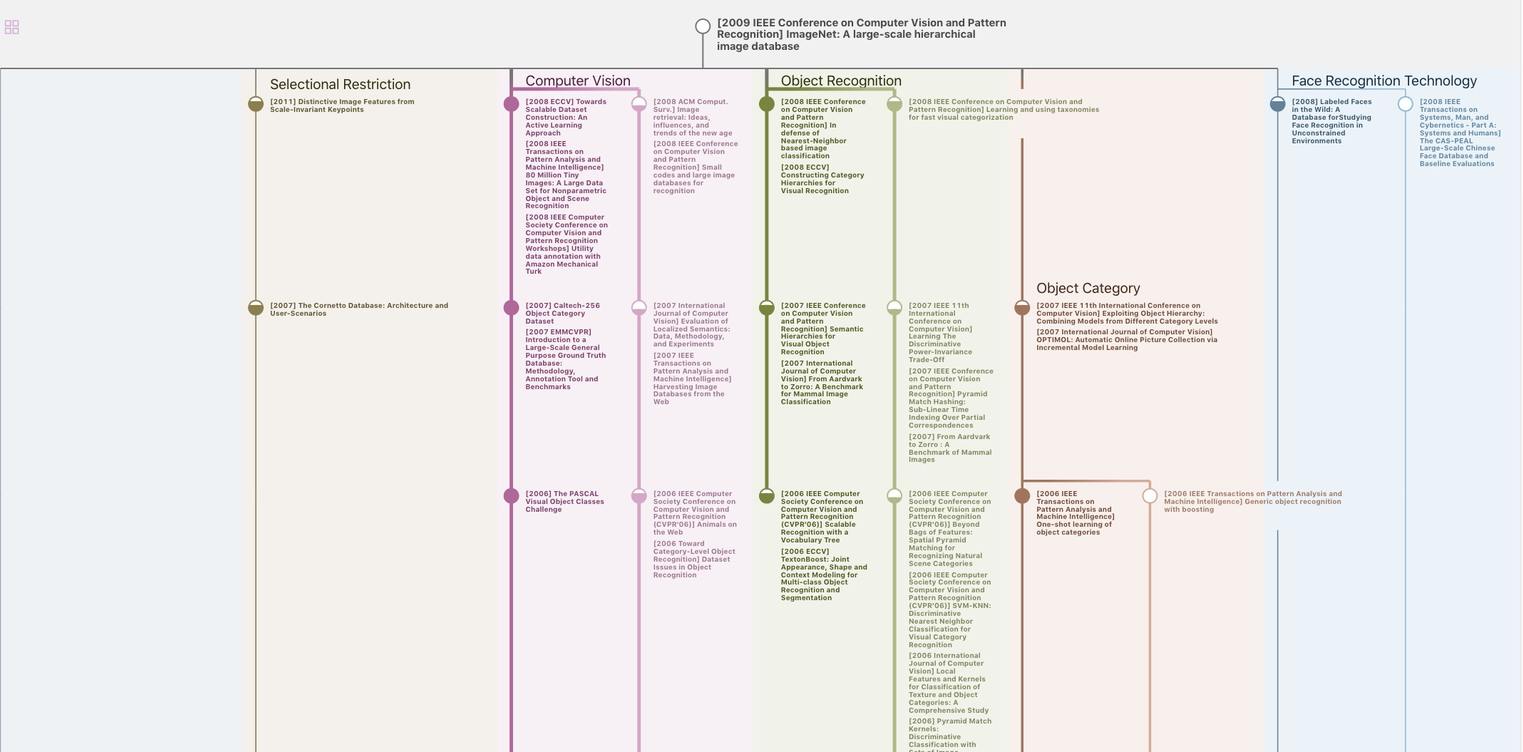
生成溯源树,研究论文发展脉络
Chat Paper
正在生成论文摘要