Strong Heuristics for Named Entity Linking
NAACL 2022: THE 2022 CONFERENCE OF THE NORTH AMERICAN CHAPTER OF THE ASSOCIATION FOR COMPUTATIONAL LINGUISTICS: HUMAN LANGUAGE TECHNOLOGIES: PROCEEDINGS OF THE STUDENT RESEARCH WORKSHOP(2022)
摘要
Named entity linking (NEL) in news is a challenging endeavour due to the frequency of unseen and emerging entities, which necessitates the use of unsupervised or zero-shot methods. However, such methods tend to come with caveats, such as no integration of suitable knowledge bases (like Wikidata) for emerging entities, a lack of scalability, and poor interpretability. Here, we consider person disambiguation in QUOTEBANK, a massive corpus of speaker-attributed quotations from the news, and investigate the suitability of intuitive, lightweight, and scalable heuristics for NEL in web-scale corpora. Our best performing heuristic disambiguates 94% and 63% of the mentions on QUOTEBANK and the AIDA-CoNLL benchmark, respectively. Additionally, the proposed heuristics compare favourably to the state-of-the-art unsupervised and zero-shot methods, EIGENTHEMES and mGENRE, respectively, thereby serving as strong baselines for unsupervised and zero-shot entity linking.
更多查看译文
AI 理解论文
溯源树
样例
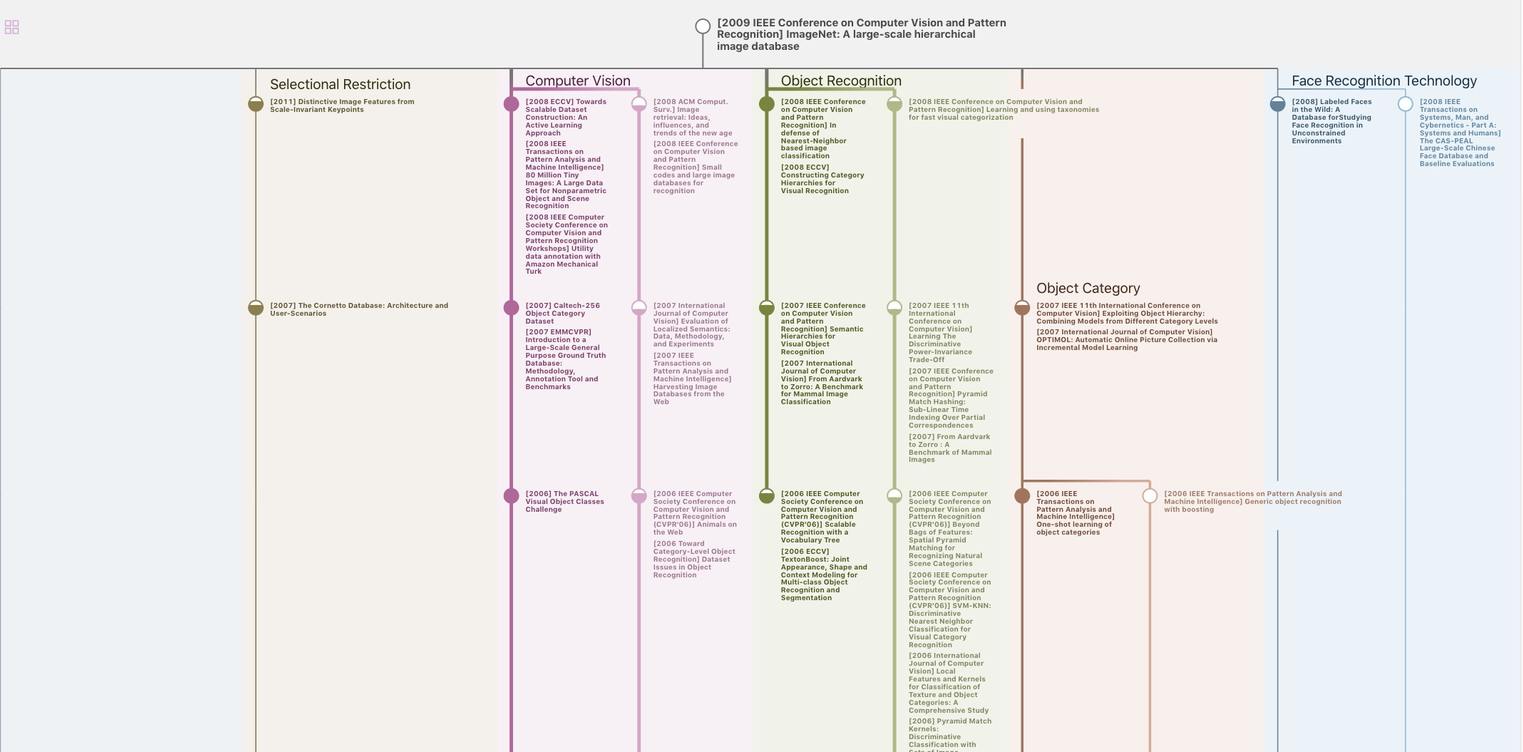
生成溯源树,研究论文发展脉络
Chat Paper
正在生成论文摘要