Delving into Sequential Patches for Deepfake Detection
NeurIPS 2022(2022)
摘要
Recent advances in face forgery techniques produce nearly visually untraceable deepfake videos, which could be leveraged with malicious intentions. As a result, researchers have been devoted to deepfake detection. Previous studies have identified the importance of local low-level cues and temporal information in pursuit to generalize well across deepfake methods, however, they still suffer from robustness problem against post-processings. In this work, we propose the Local- & Temporal-aware Transformer-based Deepfake Detection (LTTD) framework, which adopts a local-to-global learning protocol with a particular focus on the valuable temporal information within local sequences. Specifically, we propose a Local Sequence Transformer (LST), which models the temporal consistency on sequences of restricted spatial regions, where low-level information is hierarchically enhanced with shallow layers of learned 3D filters. Based on the local temporal embeddings, we then achieve the final classification in a global contrastive way. Extensive experiments on popular datasets validate that our approach effectively spots local forgery cues and achieves state-of-the-art performance.
更多查看译文
关键词
Deepfake Detection,Digital Forensics
AI 理解论文
溯源树
样例
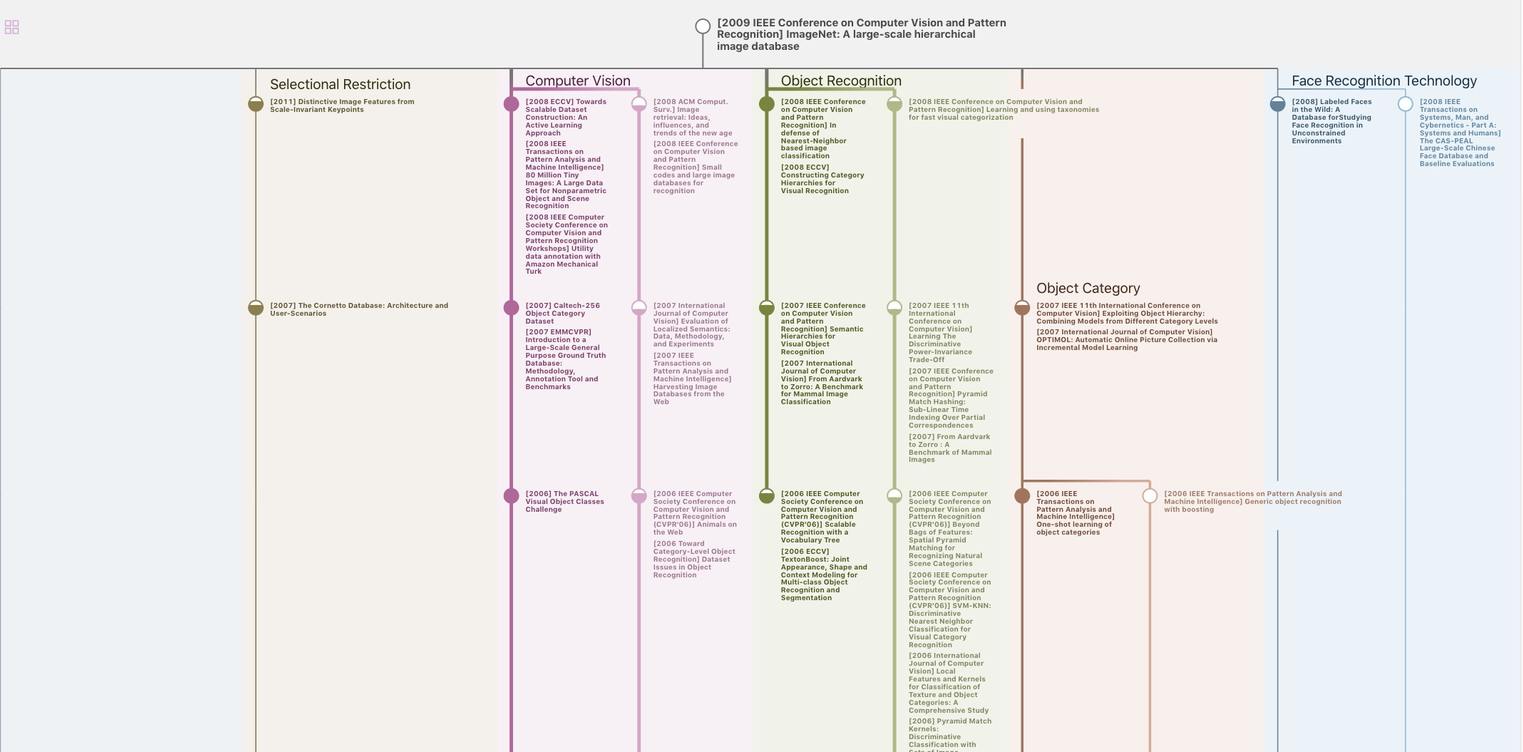
生成溯源树,研究论文发展脉络
Chat Paper
正在生成论文摘要