Quantitative Assessment of DESIS Hyperspectral Data for Plant Biodiversity Estimation in Australia.
IEEE International Geoscience and Remote Sensing Symposium (IGARSS)(2022)
摘要
Diversity of terrestrial plants plays a key role in maintaining a stable, healthy, and productive ecosystem. Though remote sensing has been seen as a promising and cost-effective proxy for estimating plant diversity, there is a lack of quantitative studies on how confidently plant diversity can be inferred from spaceborne hyperspectral data. In this study, we assessed the ability of hyperspectral data captured by the DLR Earth Sensing Imaging Spectrometer (DESIS) for estimating plant species richness in the Southern Tablelands and Snowy Mountains regions in southeast Australia. Spectral features were firstly extracted from DESIS spectra with principal component analysis, canonical correlation analysis, and partial least squares analysis. Then regression was conducted between the extracted features and plant species richness with ordinary least squares regression, kernel ridge regression, and Gaussian process regression. Results were assessed with the coefficient of correlation ($r$) and Root-Mean-Square Error (RMSE), based on a two-fold cross validation scheme. With the best performing model, $r$ is 0.71 and RMSE is 5.99 for the Southern Tablelands region, while $r$ is 0.62 and RMSE is 6.20 for the Snowy Mountains region. The assessment results reported in this study provide supports for future studies on understanding the relationship between spaceborne hyperspectral measurements and terrestrial plant biodiversity.
更多查看译文
关键词
desis hyperspectral data,biodiversity estimation,plant
AI 理解论文
溯源树
样例
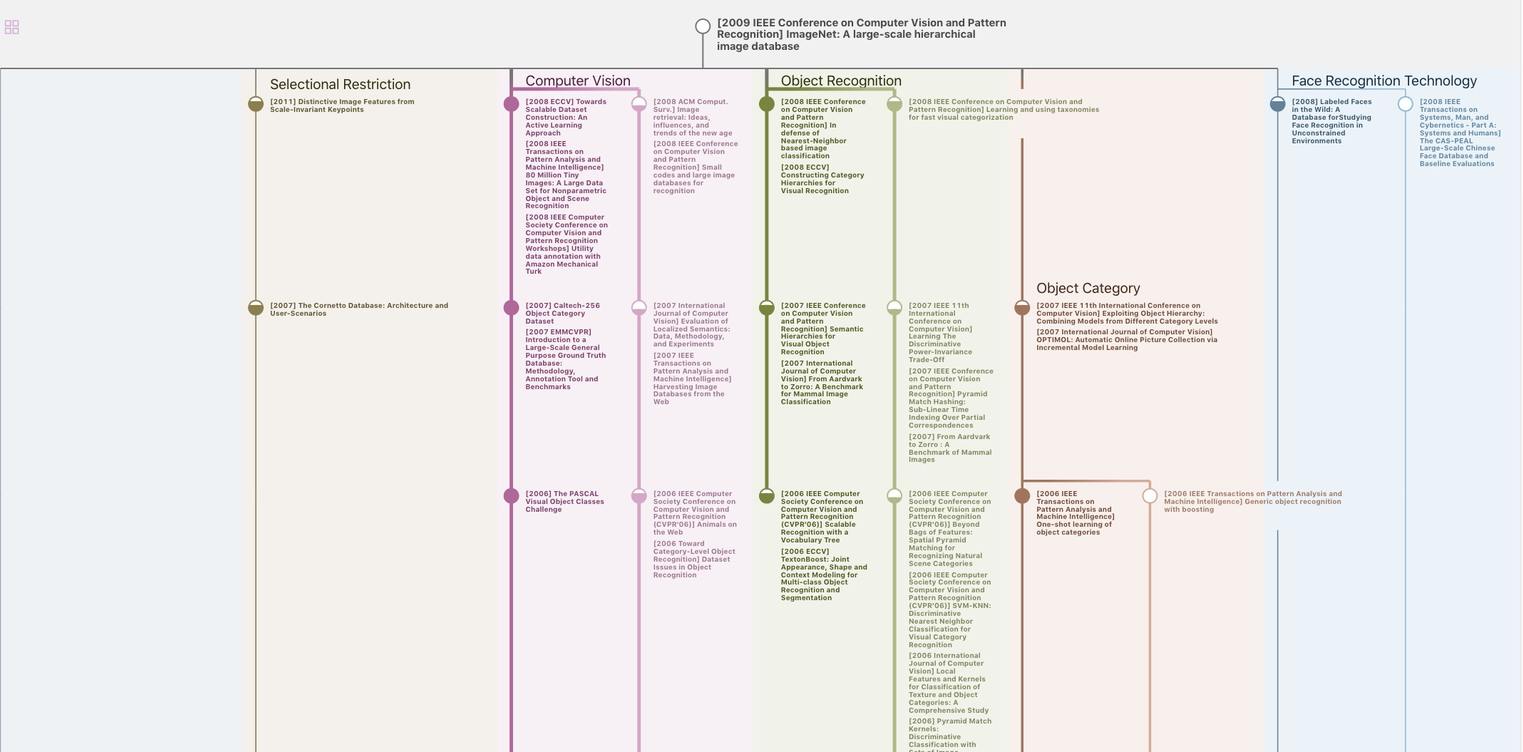
生成溯源树,研究论文发展脉络
Chat Paper
正在生成论文摘要