GID: Global information distillation for medical semantic segmentation
Neurocomputing(2022)
摘要
In this work, we consider transferring global information from Transformer to Convolutional Neural Network (CNN) for medical semantic segmentation tasks. Previous network models for medical semantic segmentation tasks often suffer from difficulties in modeling global information or oversized model parameters. Here, to design a compact network with global and local information, we extract the global information modeling capability of Transformer into the CNN network and successfully apply it to the medical semantic segmentation tasks, called Global Information Distillation. In addition, the following two contributions are proposed to improve the effectiveness of distillation: i) We present an Information Transfer Module, which is based on a convolutional layer to prevent over-regularization and a Transformer layer to transfer global information; ii) For purpose of better transferring the teacher’s soft targets, a Shrinking Result-Pixel distillation method is proposed in this paper. The effectiveness of our knowledge distillation approach is demonstrated by the experiments on multi-organ and cardiac segmentation tasks.
更多查看译文
关键词
Knowledge distillation,Medical semantic segmentation,Convolutional neural network,Transformer,Global information
AI 理解论文
溯源树
样例
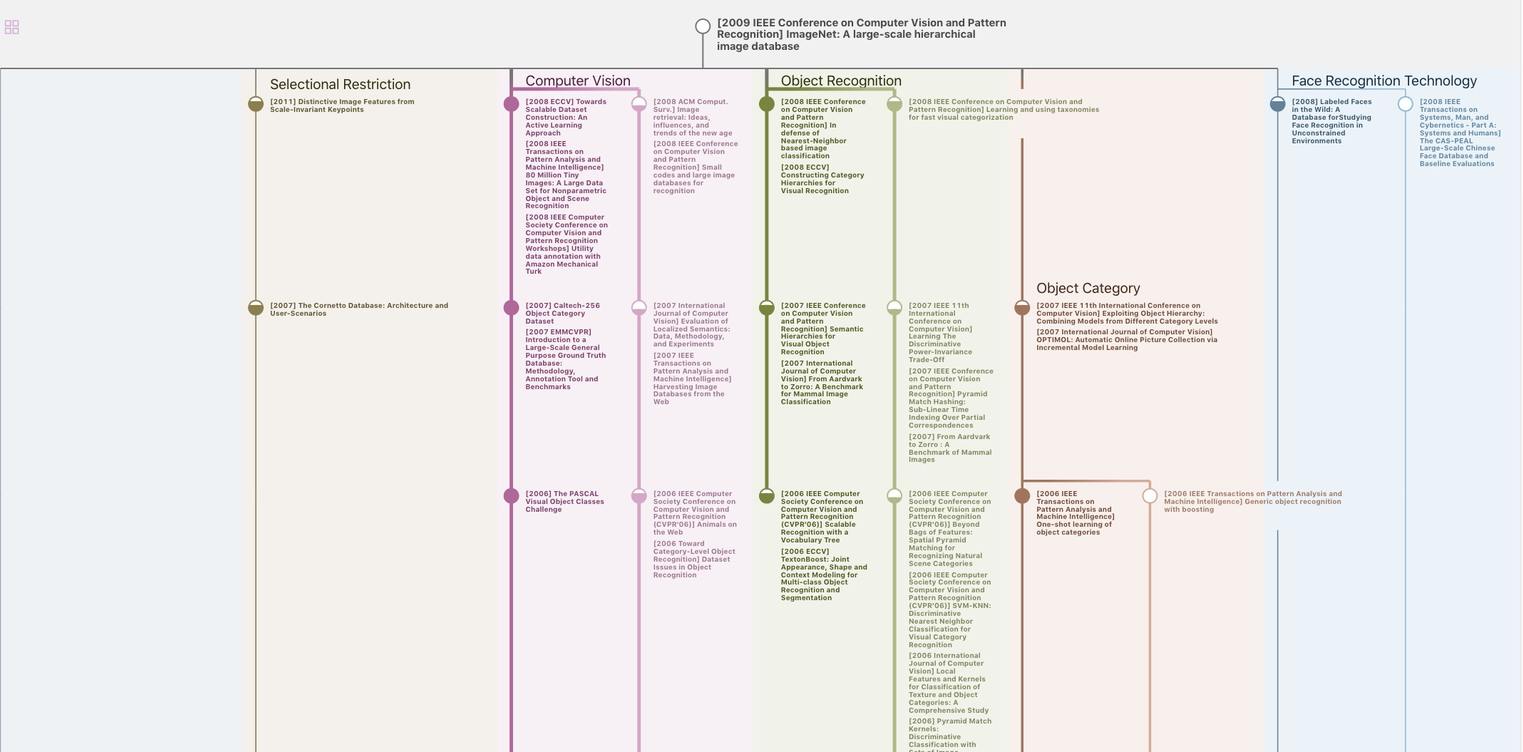
生成溯源树,研究论文发展脉络
Chat Paper
正在生成论文摘要