Dynamics of market making algorithms in dealer markets: Learning and tacit collusion
MATHEMATICAL FINANCE(2024)
摘要
The widespread use of market-making algorithms in electronic over-the-counter markets may give rise to unexpected effects resulting from the autonomous learning dynamics of these algorithms. In particular the possibility of "tacit collusion" among market makers has increasingly received regulatory scrutiny. We model the interaction of market makers in a dealer market as a stochastic differential game of intensity control with partial information and study the resulting dynamics of bid-ask spreads. Competition among dealers is modeled as a Nash equilibrium, while collusion is described in terms of Pareto optima. Using a decentralized multi-agent deep reinforcement learning algorithm to model how competing market makers learn to adjust their quotes, we show that the interaction of market making algorithms via market prices, without any sharing of information, may give rise to tacit collusion, with spread levels strictly above the competitive equilibrium level.
更多查看译文
关键词
differential games,decentralized learning,intensity control,learning,Market microstructure,market making,multi-agent actor-critic algorithm,Nash equilibrium,reinforcement tacit collusion
AI 理解论文
溯源树
样例
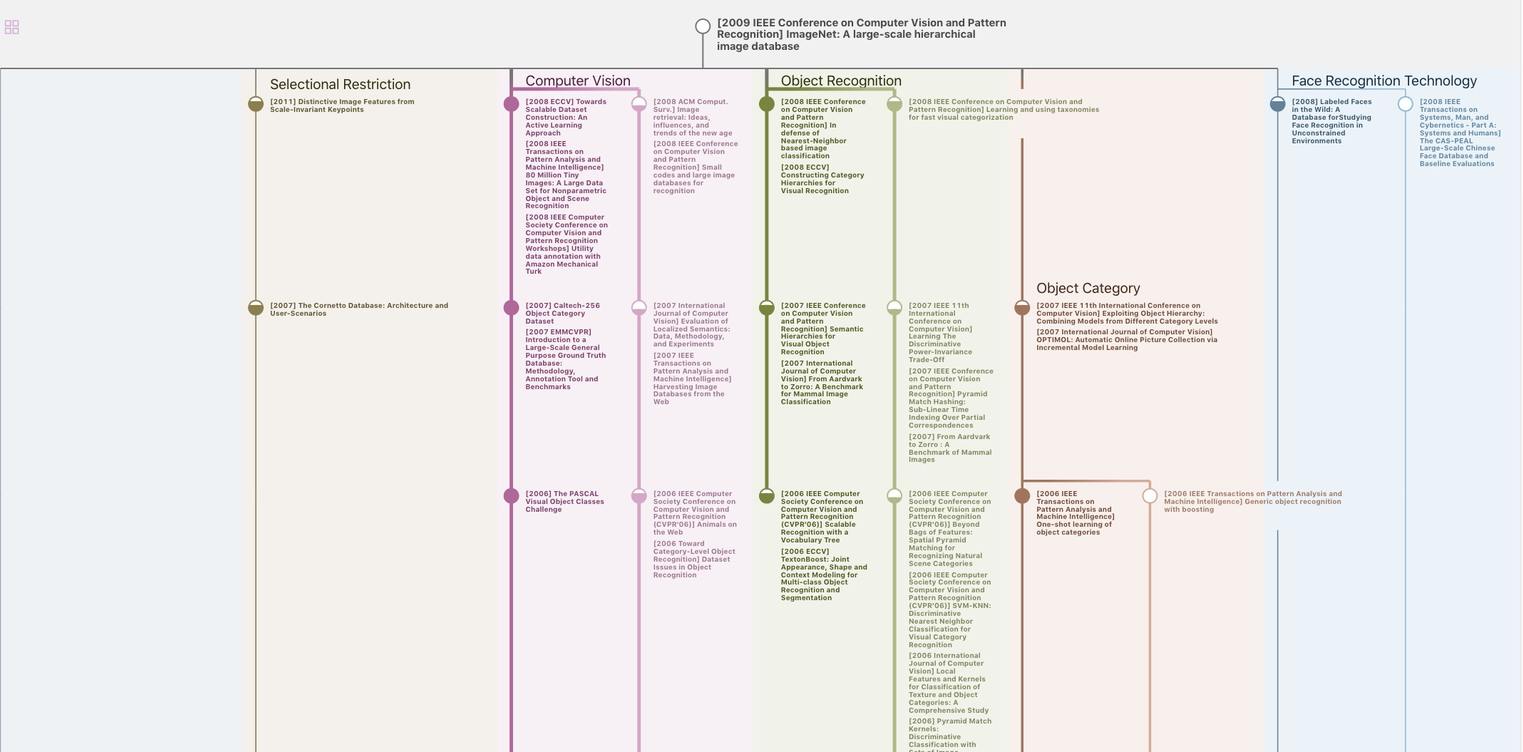
生成溯源树,研究论文发展脉络
Chat Paper
正在生成论文摘要