To make yourself invisible with Adversarial Semantic Contours
Computer Vision and Image Understanding(2023)
摘要
Modern object detectors are vulnerable to adversarial examples, which may bring risks to real-world applications. The sparse attack is an important task which, compared with the popular adversarial perturbation on the whole image, needs to select the potential pixels that is generally regularized by an ℓ0-norm constraint, and simultaneously optimize the corresponding texture. The non-differentiability of ℓ0 norm brings challenges and many works on attacking object detection adopted manually-designed patterns to address them, which are meaningless and independent of objects, and therefore lead to relatively poor attack performance. In this paper, we propose Adversarial Semantic Contour (ASC), an MAP estimate of a Bayesian formulation of sparse attack with a deceived prior of object contour. The object contour prior effectively reduces the search space of pixel selection and improves the attack by introducing more semantic bias. Extensive experiments demonstrate that ASC can corrupt the prediction of 9 modern detectors with different architectures (e.g., one-stage, two-stage and Transformer) by modifying fewer than 5% of the pixels of the object area in COCO in white-box scenario and around 10% of those in black-box scenario. We further extend the attack to datasets for autonomous driving systems to verify the effectiveness. We conclude with cautions about contour being the common weakness of object detectors with various architecture and the care needed in applying them in safety-sensitive scenarios.
更多查看译文
关键词
Adversarial examples,Sparse attacks,Object detection,Detection transformer
AI 理解论文
溯源树
样例
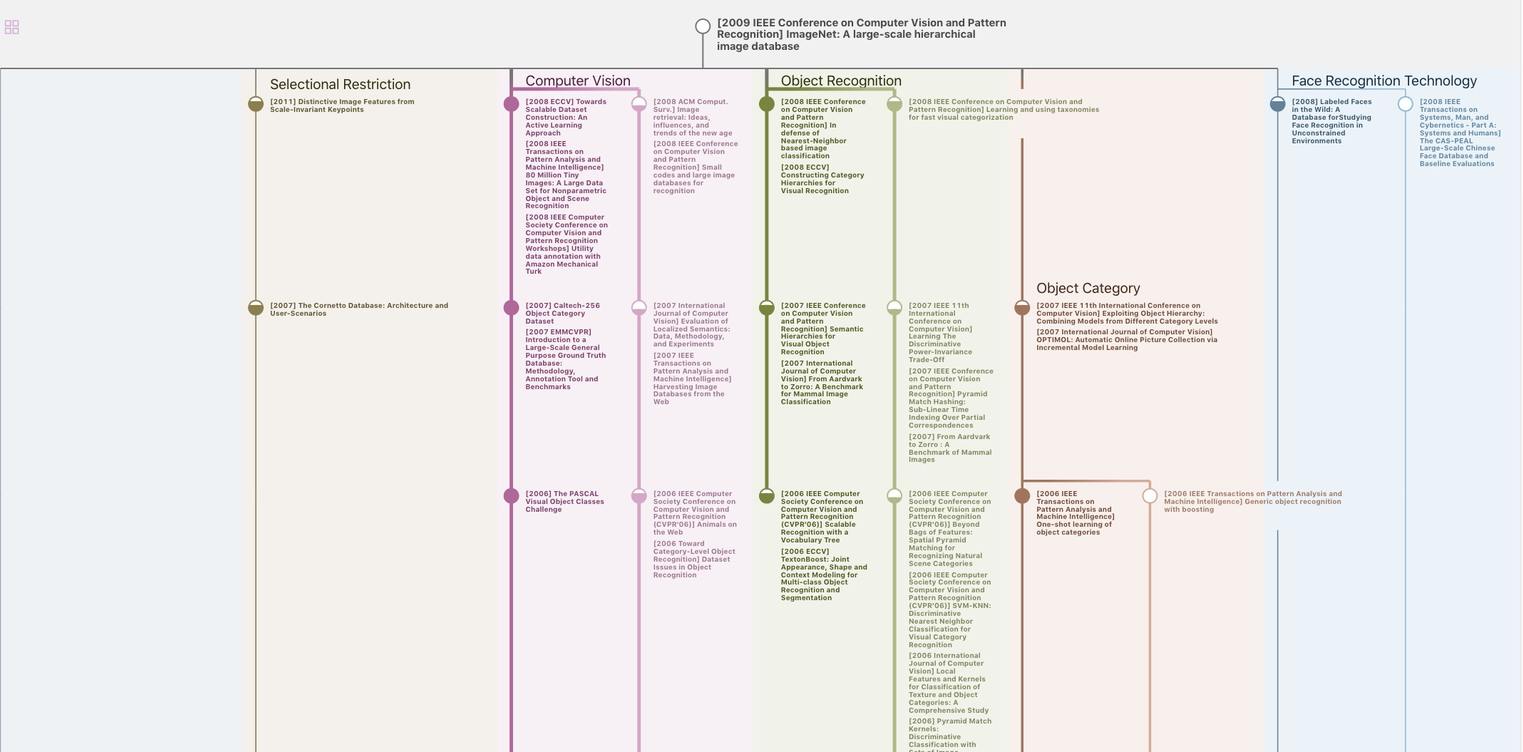
生成溯源树,研究论文发展脉络
Chat Paper
正在生成论文摘要