Numerical Bifurcation Analysis of PDEs From Lattice Boltzmann Model Simulations: a Parsimonious Machine Learning Approach
Journal of Scientific Computing(2022)
摘要
We address a three-tier data-driven approach for the numerical solution of the inverse problem in Partial Differential Equations (PDEs) and for their numerical bifurcation analysis from spatio-temporal data produced by Lattice Boltzmann model simulations using machine learning. In the first step, we exploit manifold learning and in particular parsimonious Diffusion Maps using leave-one-out cross-validation (LOOCV) to both identify the intrinsic dimension of the manifold where the emergent dynamics evolve and for feature selection over the parameter space. In the second step, based on the selected features, we learn the right-hand-side of the effective PDEs using two machine learning schemes, namely shallow Feedforward Neural Networks (FNNs) with two hidden layers and single-layer Random Projection Networks (RPNNs), which basis functions are constructed using an appropriate random sampling approach. Finally, based on the learned black-box PDE model, we construct the corresponding bifurcation diagram, thus exploiting the numerical bifurcation analysis toolkit. For our illustrations, we implemented the proposed method to perform numerical bifurcation analysis of the 1D FitzHugh-Nagumo PDEs from data generated by D 1 Q 3 Lattice Boltzmann simulations. The proposed method was quite effective in terms of numerical accuracy regarding the construction of the coarse-scale bifurcation diagram. Furthermore, the proposed RPNN scheme was ∼ 20 to 30 times less costly regarding the training phase than the traditional shallow FNNs, thus arising as a promising alternative to deep learning for the data-driven numerical solution of the inverse problem for high-dimensional PDEs.
更多查看译文
关键词
Machine learning,Lattice Boltzmann modelling,Diffusion maps,Partial differential equations,Inverse problem,Random projection Neural networks,Numerical bifurcation analysis
AI 理解论文
溯源树
样例
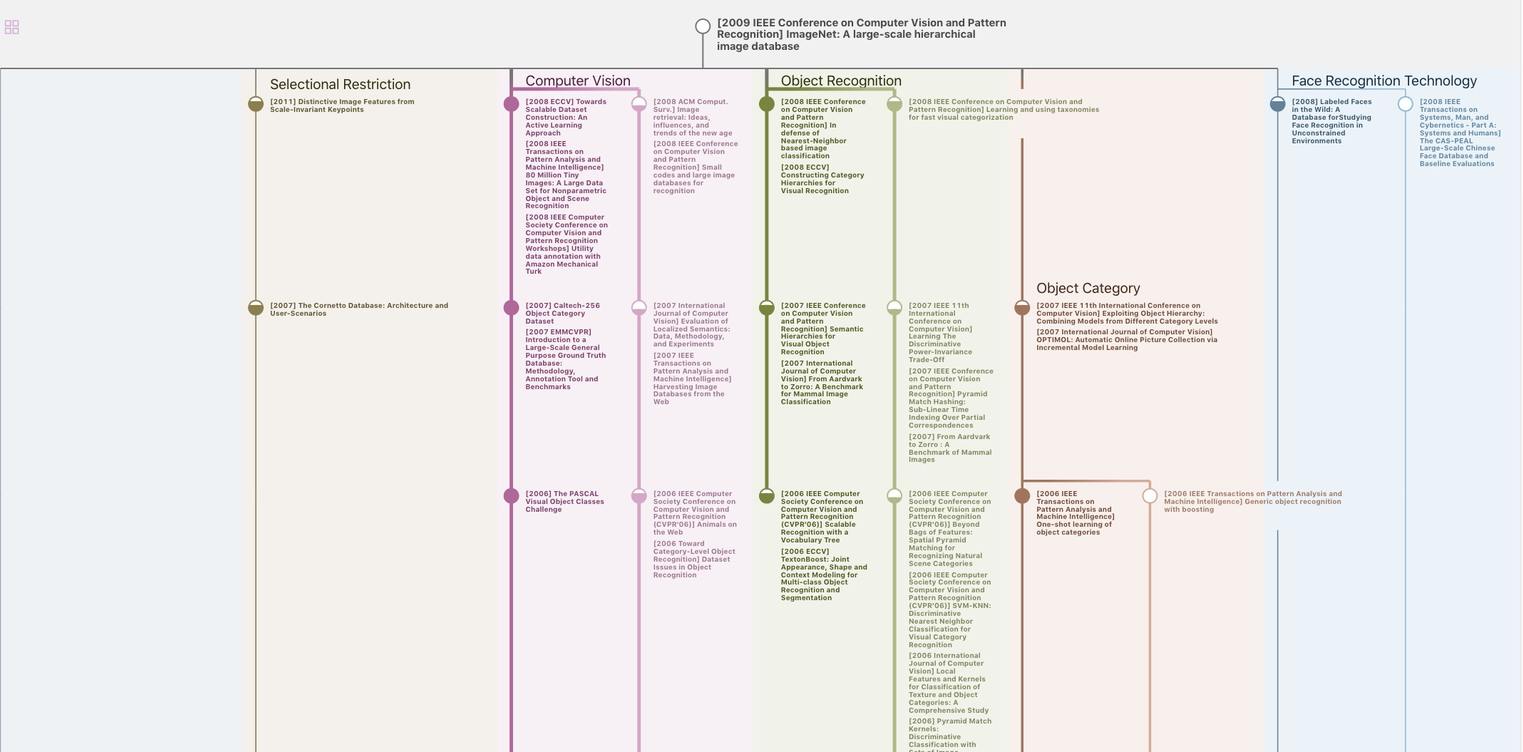
生成溯源树,研究论文发展脉络
Chat Paper
正在生成论文摘要