A Local Correspondence-Aware Hybrid CNN-GCN Model for Single-Image Human Body Reconstruction
IEEE Transactions on Multimedia(2023)
摘要
Reconstructing a 3D human body mesh from a monocular image is a challenging inverse problem because of occlusion and complicated human articulations. Recent deep learning-based methods have made significant progress in single-image human reconstruction. Most of these works are either model-based methods or model-free methods. However, model-based methods always suffer detail losses due to the limited parameter space, and model-free methods are hard to directly recover satisfactory results from images due to the use of a shared global feature for all vertices and the domain gap between 2D regular images and 3D irregular meshes. To resolve these issues, we propose a hybrid model, which combines the advantages of both model based approach and model-free approach to estimate a 3D human mesh in a coarse-to fine manner. Initially, we utilize a convolutional neural network (CNN) to estimate the parameters of a Skinned Multi-Person Linear Model (SMPL), which allows us to generate a coarse human mesh. After that, the vertex coordinates of the coarse human mesh are further refined by a graph convolutional neural network (GCN). Unlike previous GCN-based methods, whose vertex coordinates are recovered from a shared global feature, we propose a LOcal CorRespondence-Aware (LOCRA) module to extract local special features for each vertex. To make the local features related to the human pose, we also add a keypoint-related loss to supervise the training process of the LOCRA module. Experiments demonstrate that our hybrid model with the LOCRA module outperforms existing methods on multiple public benchmarks.
更多查看译文
关键词
reconstruction,correspondence-aware,cnn-gcn,single-image
AI 理解论文
溯源树
样例
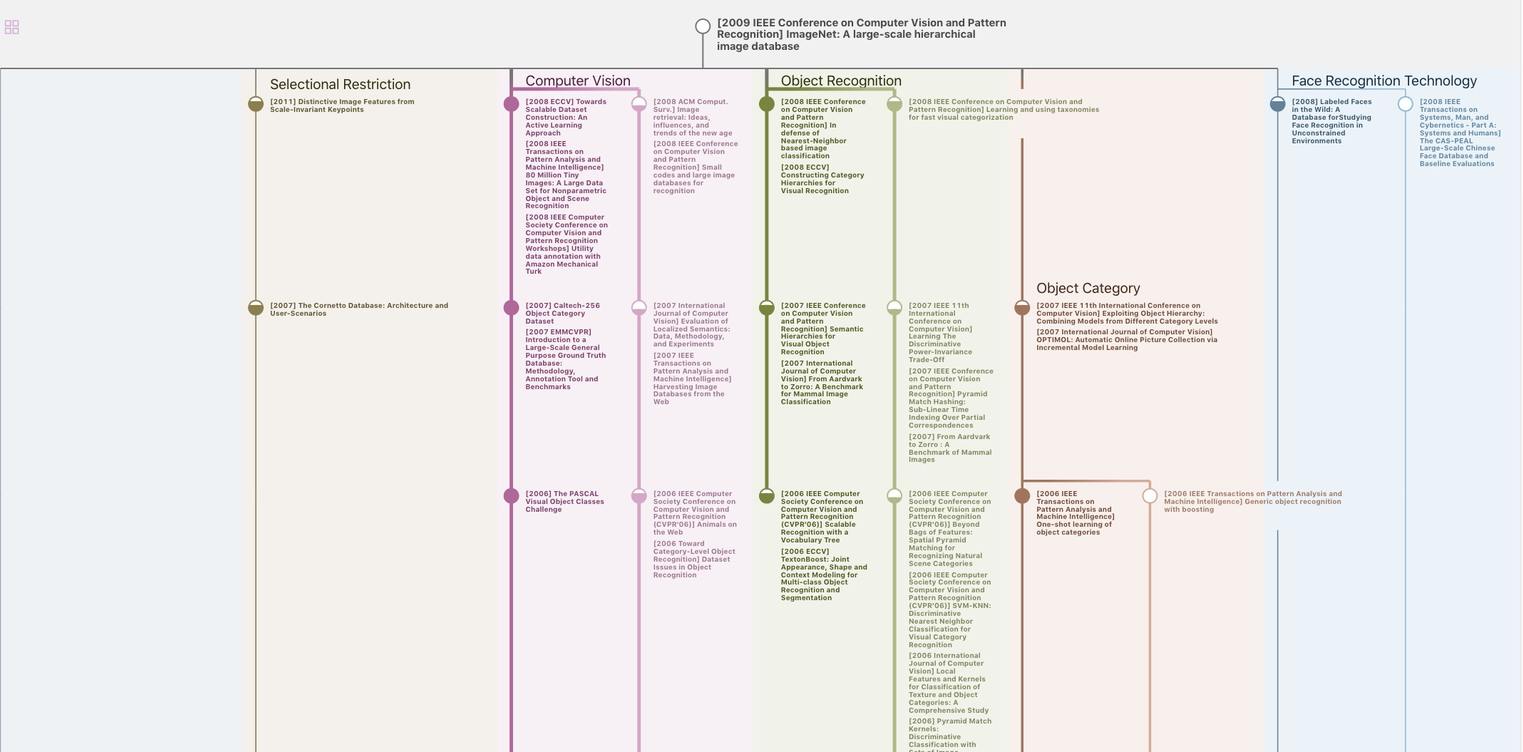
生成溯源树,研究论文发展脉络
Chat Paper
正在生成论文摘要