Adversarial training for deep learning-based cyberattack detection in IoT-based smart city applications
Computers & Security(2022)
摘要
Intrusion Detection Systems (IDS) based on deep learning models can identify and mitigate cyberattacks in IoT applications in a resilient and systematic manner. These models, which support the IDS’s decision, could be vulnerable to a cyberattack known as adversarial attack. In this type of attack, attackers create adversarial samples by introducing small perturbations to attack samples to trick a trained model into misclassifying them as benign applications. These attacks can cause substantial damage to IoT-based smart city models in terms of device malfunction, data leakage, operational outage and financial loss. To our knowledge, the impact of and defence against adversarial attacks on IDS models in relation to smart city applications have not been investigated yet. To address this research gap, in this work, we explore the effect of adversarial attacks on the deep learning and shallow machine learning models by using a recent IoT dataset and propose a method using adversarial retraining that can significantly improve IDS performance when confronting adversarial attacks. Simulation results demonstrate that the presence of adversarial samples deteriorates the detection accuracy significantly by above 70% while our proposed model can deliver detection accuracy above 99% against all types of attacks including adversarial attacks. This makes an IDS robust in protecting IoT-based smart city services.
更多查看译文
关键词
Smart city,Internet of things,Cyberattacks,Deep learning,Machine learning,Retraining
AI 理解论文
溯源树
样例
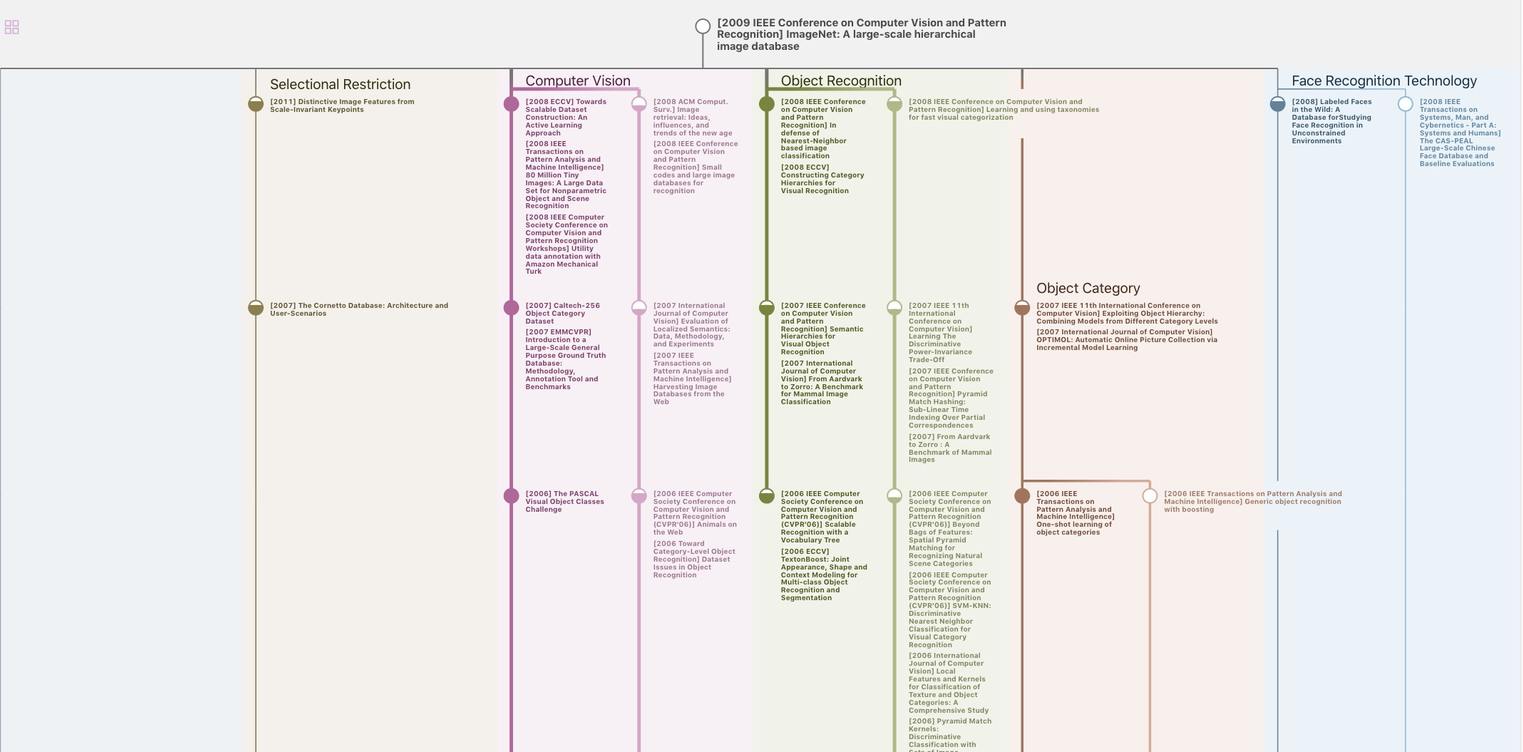
生成溯源树,研究论文发展脉络
Chat Paper
正在生成论文摘要