A migration learning-based transient stability and impact assessment method for hybrid AC-DC power system faults
2022 7th Asia Conference on Power and Electrical Engineering (ACPEE)(2022)
摘要
With increasing penetration of intermittent renewable generation, the mode and characteristics of power system instability have become more complex and difficult to predict. Traditional machine learning prediction models, with different operation modes and topologies of the power grid system, suffers from poor prediction accuracy. This paper proposes a migration learning-based transient stability and quantitative evaluation of fault impact assessment method for hybrid AC-DC power system. Firstly, the characteristics of AC-DC hybrid power grid transient stability feature extraction and pointer are analyzed, then migration learning model and ResNet and their advantages in grid transient prediction are discussed, and a prediction model based on migration learning is built using ResNet18. Finally, the method is applied to the IEEE-39 node test system. Results show that the proposed method can carry out fast stability identification with small number of training samples by using positive sequence voltage data. It has better generalization capability and robustness than traditional machine learning methods.
更多查看译文
关键词
transient stability,migration learning,residual neural networks,fault range assessment,power systems
AI 理解论文
溯源树
样例
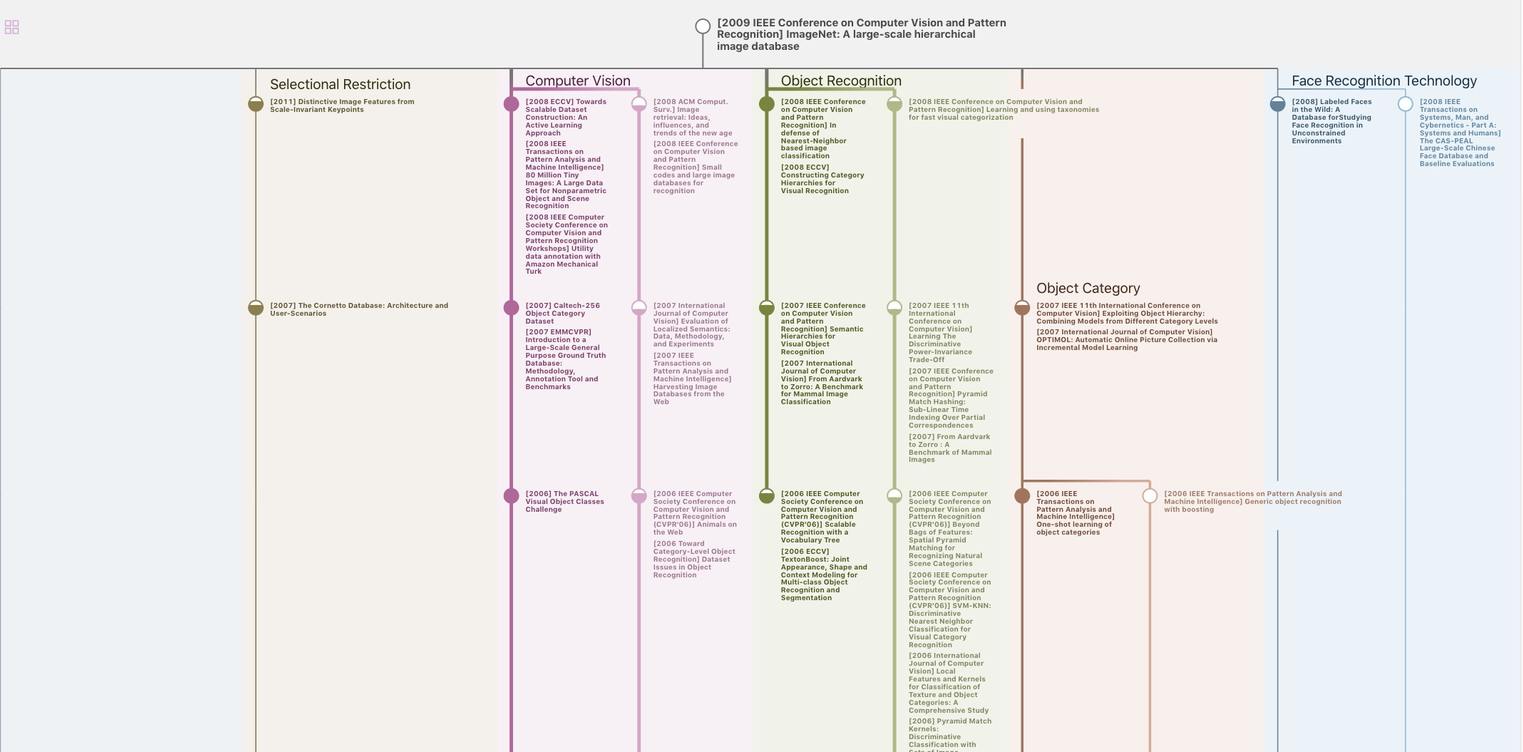
生成溯源树,研究论文发展脉络
Chat Paper
正在生成论文摘要