Optimal Balance of Privacy and Utility with Differential Privacy Deep Learning Frameworks
2021 International Conference on Computational Science and Computational Intelligence (CSCI)(2021)
摘要
As the number of online services has increased, the amount of sensitive data being recorded is rising. Simultaneously, the decision-making process has improved by using the vast amounts of data, where machine learning has transformed entire industries. This paper addresses the development of optimal private deep neural networks and discusses the challenges associated with this task. We focus on differential privacy implementations and finding the optimal balance between accuracy and privacy, benefits and limitations of existing libraries, and challenges of applying private machine learning models in practical applications. Our analysis shows that learning rate, and privacy budget are the key factors that impact the results, and we discuss options for these settings.
更多查看译文
关键词
privacy,personal data,differential privacy,deep neural network
AI 理解论文
溯源树
样例
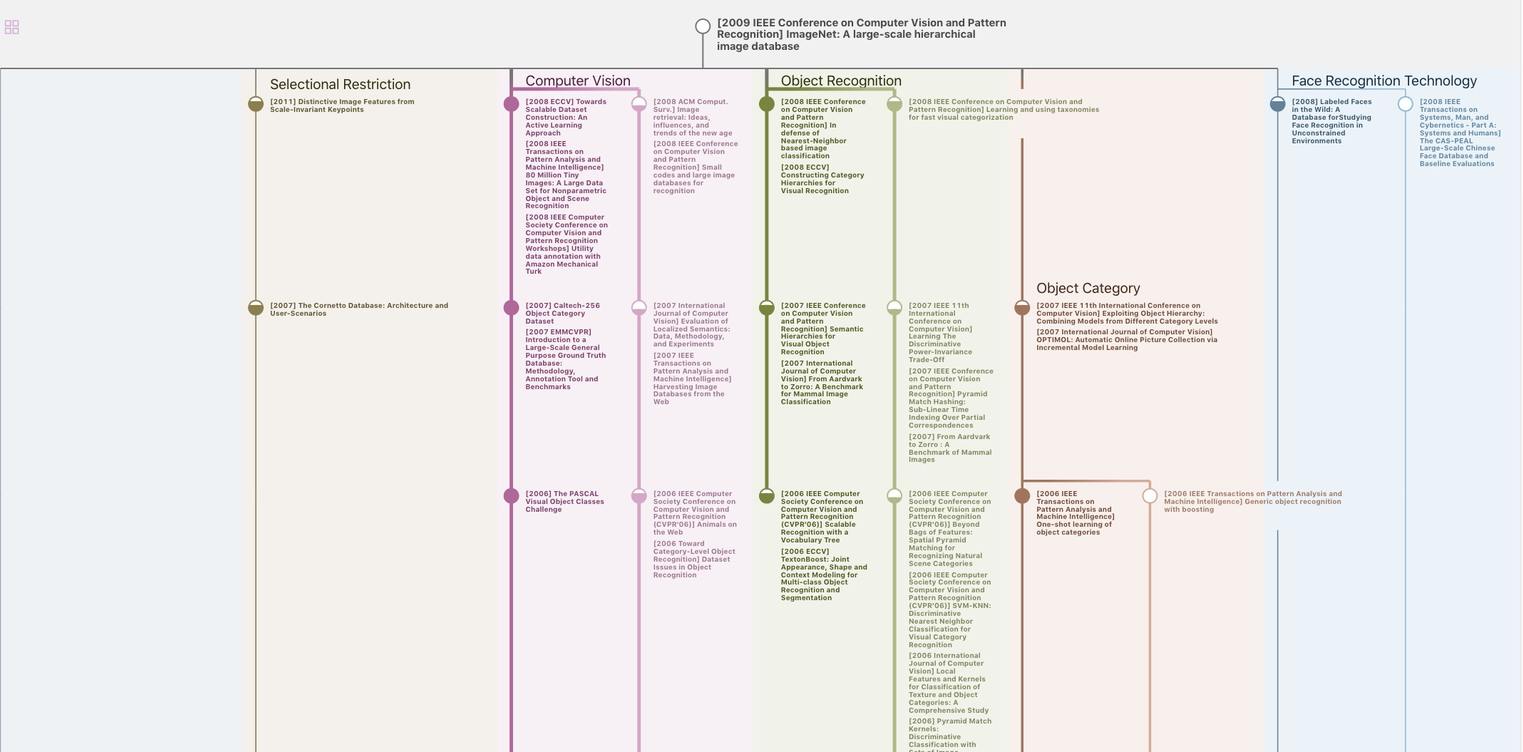
生成溯源树,研究论文发展脉络
Chat Paper
正在生成论文摘要