LiteCON: An All-photonic Neuromorphic Accelerator for Energy-efficient Deep Learning
ACM TRANSACTIONS ON ARCHITECTURE AND CODE OPTIMIZATION(2022)
摘要
Deep learning is highly pervasive in today's data-intensive era. In particular, convolutional neural networks (CNNs) are being widely adopted in a variety of fields for superior accuracy. However, computing deep CNNs on traditional CPUs and GPUs brings several performance and energy pitfalls. Several novel approaches based on ASIC, FPGA, and resistive-memory devices have been recently demonstrated with promising results. Most of them target only the inference (testing) phase of deep learning. There have been very limited attempts to design a full-fledged deep learning accelerator capable of both training and inference. It is due to the highly compute- and memory-intensive nature of the training phase. In this article, we propose LiteCON, a novel analog photonics CNN accelerator. LiteCON uses silicon microdisk-based convolution, memristor-based memory, and dense-wavelength-division-multiplexing for energy-efficient and ultrafast deep learning. We evaluate LiteCON using a commercial CAD framework (IPKISS) on deep learning benchmark models including LeNet and VGG-Net. Compared to the state of the art, LiteCON improves the CNN throughput, energy efficiency, and computational efficiency by up to 32x, 37x, and 5x, respectively, with trivial accuracy degradation.
更多查看译文
关键词
Deep learning,on-chip photonics,memristor
AI 理解论文
溯源树
样例
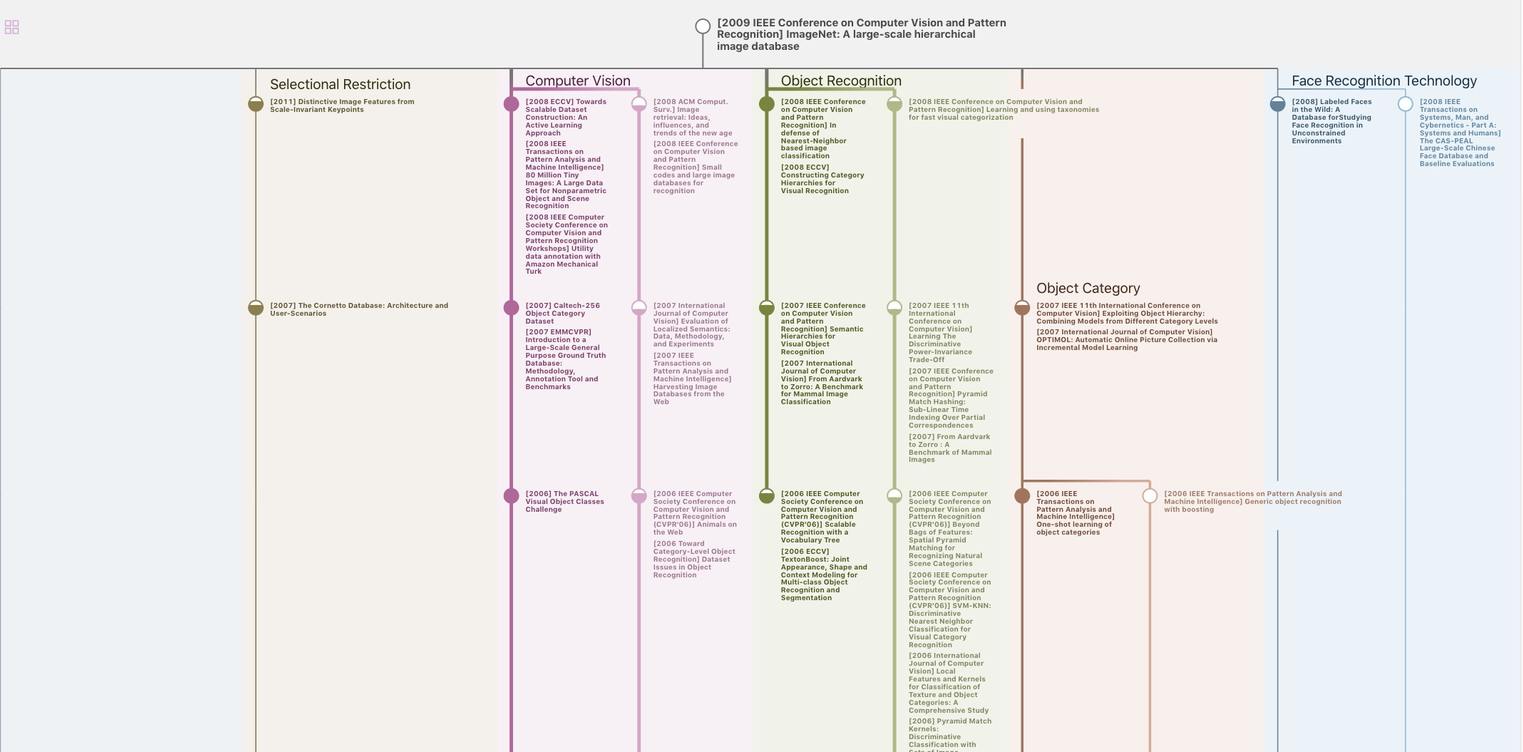
生成溯源树,研究论文发展脉络
Chat Paper
正在生成论文摘要