A transfer learning approach for reaction discovery in small data situations using generative model
ISCIENCE(2022)
摘要
Sustainable practices in chemical sciences can be better realized by adopting interdisciplinary approaches that combine the advantages of machine learning (ML) on the initially acquired small data in reaction discovery. Developing new re-actions generally remains heuristic and even time and resource intensive. For instance, synthesis of fluorine-containing compounds, which constitute -20% of the marketed drugs, relies on deoxyfluorination of abundantly available alco-hols. Herein, we demonstrate the use of a recurrent neural network-based deep generative model built on a library of just 37 alcohols for effective learning and exploration of the chemical space. The proof-of-concept ML model is able to generate good quality, synthetically accessible, higher-yielding novel alcohol molecules. This protocol would have superior utility for deployment into a prac-tical reaction discovery pipeline.
更多查看译文
关键词
Artificial intelligence,Computational chemistry,Functional group chemistry,Modeling chemical reactivity
AI 理解论文
溯源树
样例
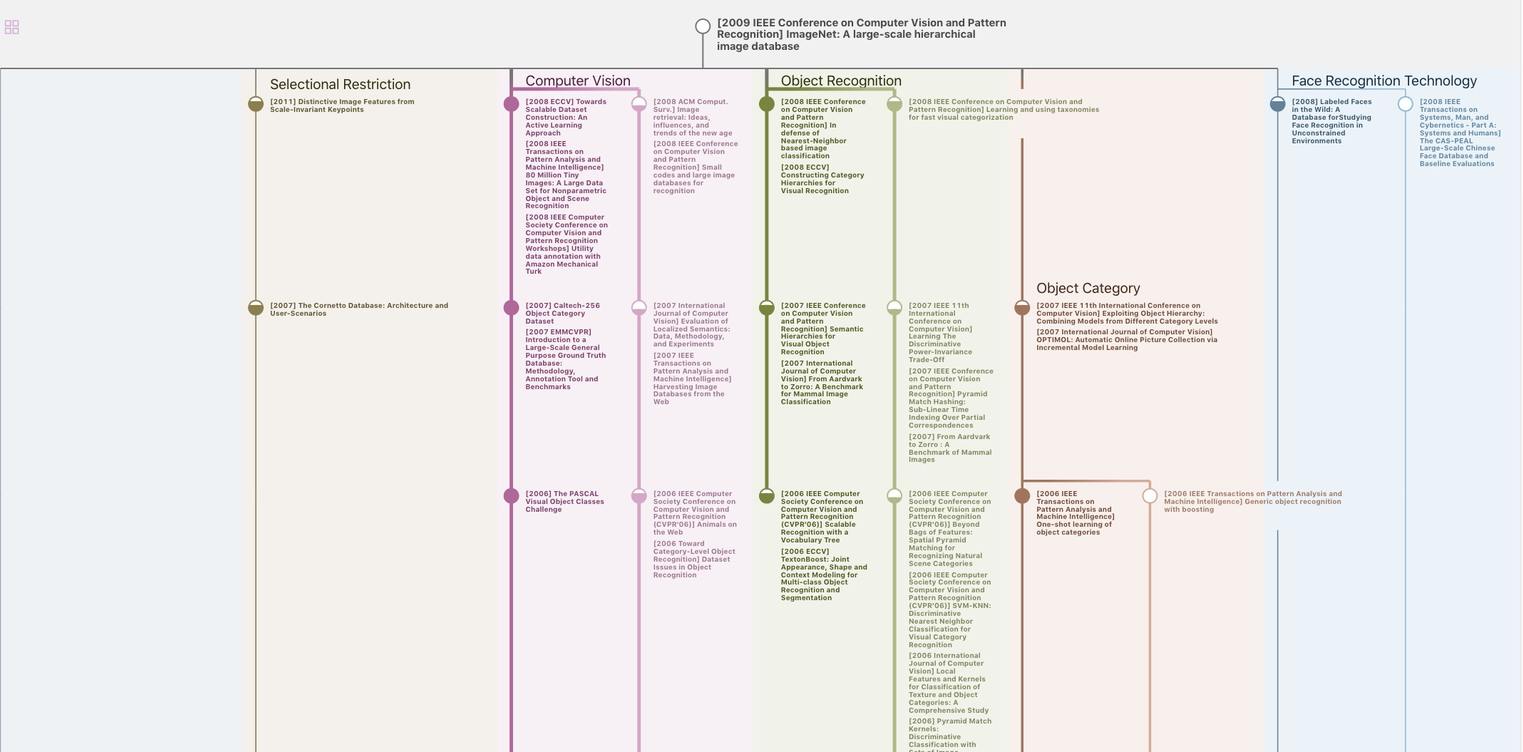
生成溯源树,研究论文发展脉络
Chat Paper
正在生成论文摘要