A Machine Learning Solution for Video Delivery to Mitigate Co-Tier Interference in 5G HetNets
IEEE Transactions on Multimedia(2023)
摘要
The exponential demand for multimedia services is one reason behind the substantial growth of mobile data traffic. Video traffic patterns have significantly changed in the past two years due to the coronavirus disease (COVID-19). The worldwide pandemic has caused many individuals to work from home and use various online video platforms (
e.g.,
Zoom, Google Meet, and Microsoft Teams). As a result, overloaded macrocells are unable to ensure high Quality of Experience (QoE) to all users. Heterogeneous Networks (HetNets) consisting of small cells (femtocells) and macrocells are a promising solution to mitigate this problem. A critical challenge with the deployment of femtocells in HetNets is the interference management between Macro Base Stations (MBSs), Femto Base Stations (FBSs), and between FBS and FBS. Indeed, the dynamic deployment of femtocells can lead to co-tier interference. With the rolling out of the 5G mobile network, it becomes imperative for mobile operators to maintain network capacity and manage different types of interference. Machine Learning (ML) is considered a promising solution to many challenges in 5G HetNets. In this paper, we propose a Machine Learning Interference Classification and Offloading Scheme (MLICOS) to address the problem of co-tier interference between femtocells for video delivery. Two versions of MLICOS, namely, MLICOS1 and MLICOS2, are proposed. The former uses conventional ML classifiers while the latter employs advanced ML algorithms. Both versions of MLICOS are compared with the classic Proportional Fair (PF) scheduling algorithm, Variable Radius and Proportional Fair scheduling (VR + PF) algorithm, and a Cognitive Approach (CA). The ML models are assessed based on the prediction accuracy, precision, recall and F-measure. Simulation results show that MLICOS outperforms the other schemes by providing the highest throughput and the lowest delay and packet loss ratio. A statistical analysis was also carried out to depict the degree of interference faced by users when different schemes are employed.
更多查看译文
关键词
HetNets,COVID-19,interference,machine learning,QoS,statistical visualization
AI 理解论文
溯源树
样例
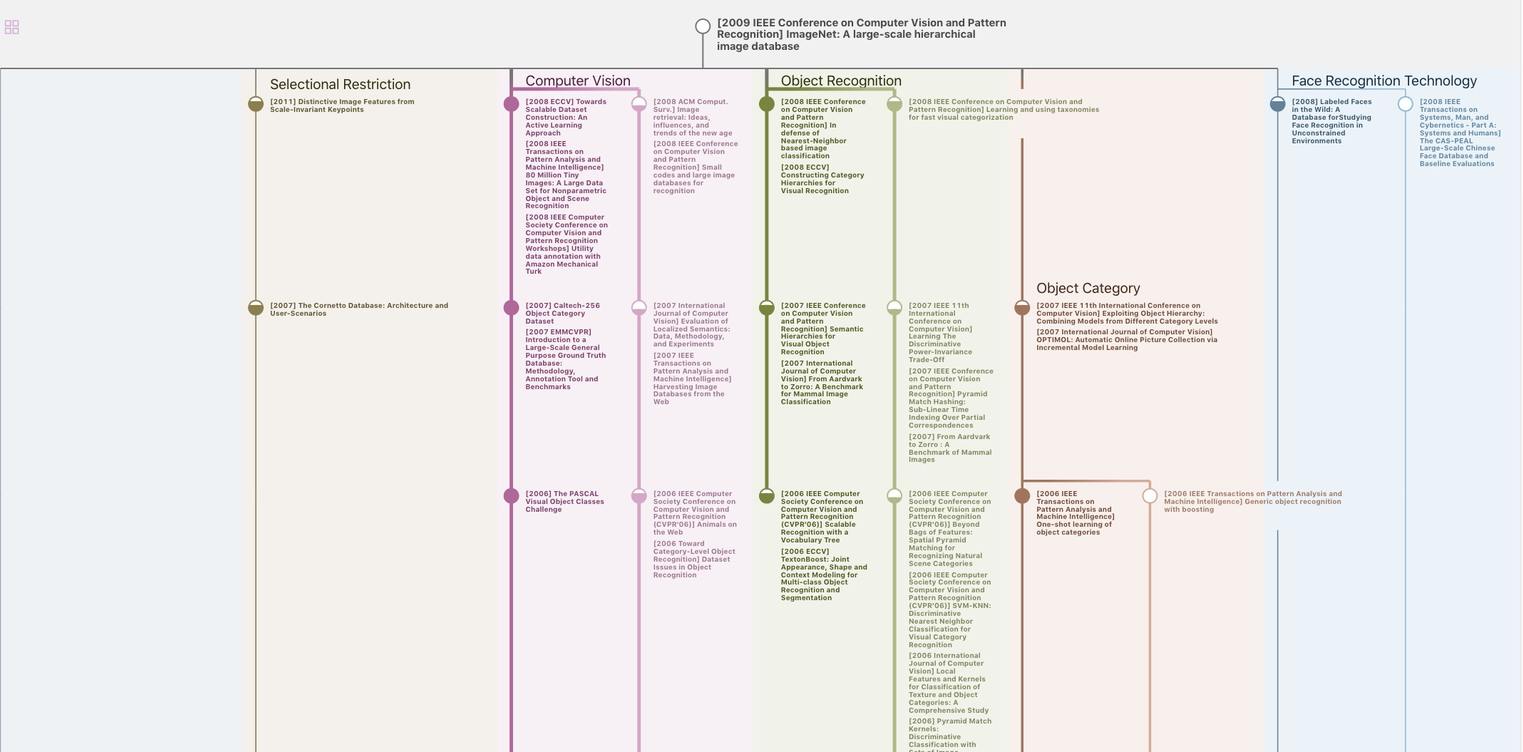
生成溯源树,研究论文发展脉络
Chat Paper
正在生成论文摘要