Unsupervised Domain Adaptation Based on Progressive Transfer for Ship Detection: From Optical to SAR Images
IEEE TRANSACTIONS ON GEOSCIENCE AND REMOTE SENSING(2022)
摘要
In recent years, synthetic aperture radar (SAR) ship detection methods based on convolutional neural networks (CNNs) have attracted wide attention in remote sensing fields. However, these methods require a large number of labeled SAR images to train the network, where labeling for SAR images is more expensive and time-consuming than that for optical images. To address the problem of lacking labeled SAR images, in this article, we proposed an unsupervised domain adaptation (UDA) framework based on progressive transfer for SAR ship detection by transferring knowledge from the optical domain to the SAR domain. Due to the prominent difference between the optical and SAR images, our approach progressively transfers knowledge from three levels: pixel level, feature level, and prediction level. At the pixel level, considering the difference in imaging mechanism, we propose a special data augmentation method for ship targets and build the generator with skip connection based on generative adversarial networks (GANs) to generate transition domain, which can reduce the appearance discrepancy between the optical and SAR images. At the feature level, the detector is trained to learn the domain-invariant features with adversarial alignment. At the prediction level, we further use the predicted pseudo-labels obtained by the feature-aligned detector to learn more discriminative features of the SAR images directly and propose the robust self-training (RST) method to reduce the influence of noisy pseudo-labels on detector training. Specially, RST is formulated as a loss minimization problem for object detection. The experimental results based on the domain adaptation from optical dataset to SAR dataset demonstrate that our approach achieves superior SAR ship detection performance with unlabeled SAR images.
更多查看译文
关键词
Optical imaging,Marine vehicles,Synthetic aperture radar,Radar polarimetry,Adaptive optics,Detectors,Optical detectors,Generative adversarial networks (GANs),optical to synthetic aperture radar (SAR),robust self-training (RST),SAR,ship detection,unsupervised domain adaptation (UDA)
AI 理解论文
溯源树
样例
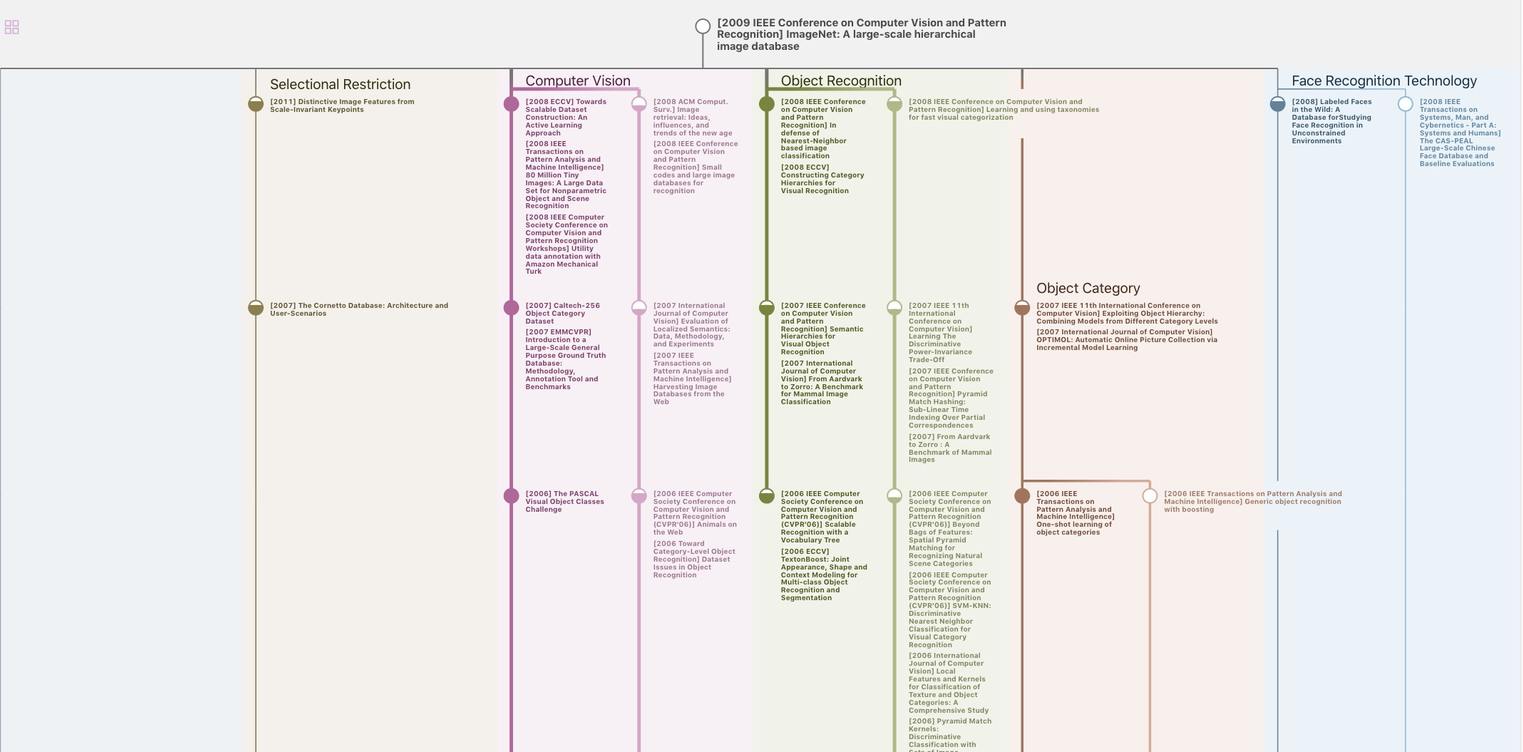
生成溯源树,研究论文发展脉络
Chat Paper
正在生成论文摘要