Pos0514 prediction of response to methotrexate in patients with rheumatoid arthritis: a machine learning approach using clinical trial data
Annals of the Rheumatic Diseases(2022)
摘要
BackgroundMethotrexate (MTX) is the preferred initial disease-modifying drug (DMARD) for rheumatoid arthritis (RA). However, up to 50% of patients respond inadequately to MTX (1). Clinically useful predictors that effectively identify patients with RA who are likely to respond to MTX are lacking. Whether machine learning (ML) can provide robust and clinically useful prediction of response to MTX monotherapy in the first months of treatment in patients with early RA using uniformly collected baseline demographics and clinical data has not been investigated in large patient populations.ObjectivesWe aimed to identify clinical predictors of response to MTX as the first DMARD among patients with RA using ML methods.MethodsRandomized clinical trials (RCT) of patients with RA who were DMARD-naïve and randomized to placebo plus MTX were identified and accessed through the Clinical Study Data Request Consortium and Vivli Center for Global Clinical Research Data. Studies with available Disease Activity Score with 28-joint count and erythrocyte sedimentation rate (DAS28-ESR) at baseline, 12 and 24 weeks were included. Latent class modeling of MTX response was performed. Least absolute shrinkage and selection operator (LASSO) and random forest were used to identify predictors of response.ResultsA total of 775 patients from 4 RCTs were included (mean age 50 years, 80% female). Two distinct classes of patients were identified based on DAS28-ESR change over 24 weeks: “good responders” and “poor responders” to MTX treatment (Figure 1). Baseline DAS28-ESR, anti-citrullinated protein antibody (ACPA) and health assessment questionnaire (HAQ) score were the top predictors of good response to MTX using LASSO (Area Under the Curve [AUC] 0.79) and Random Forest models (AUC 0.68) in the external validation set. DAS28-ESR≤7.4, ACPA positive and HAQ≤2 provided the highest likelihood of response (Table 1). Among patients with 12-week DAS28-ESR>3.2, at least 1 point improvement in DAS28-ESR baseline-to-12-week was predictive of achieving DAS28-ESR≤3.2 at 24 weeks.Table 1.Matrix prediction model: Probability of achieving a good response to methotrexate at 24 weeksDAS28ESR≤7.480.1 (76.4, 83.8)77.3 (70.6, 84)PositiveACPA Status77.1 (68.6, 85.6)74.1 (63.3, 84.9)Negative>7.440.3 (32.1, 48.5)36.5 (29.3, 43.6)Positive36.2 (23.3, 49.1)32.5 (20.9, 44.1)Negative≤2>2HAQFootnote: The number in each cell represents the percentage and 95% CI of achieving the outcome, based on the combination of predictors at baseline. DAS28-ESR: Disease Activity Score with 28-joint count with erythrocyte sedimentation rate; HAQ: Health assessment questionnaire score; ACPA: Anti-citrullinated protein antibody.Figure 1.Two patient class trajectories identified with latent class modeling of DAS28-ESR (N=775)ConclusionWe have developed and externally validated a prediction model for response to MTX within 24 weeks in DMARD-naïve patients with RA, providing variably weighted clinical features and defined cut-offs for clinical decision-making. Trajectory of DAS28-ESR change over 24 weeks in patients with moderate-to-high RA disease activity at baseline who are starting MTX can be predicted by baseline DAS28-ESR, ACPA status and HAQ-score. Patients with at least 1 unit decline in DAS28-ESR within the first 12 weeks of treatment who have not achieved low disease activity by week 12, may be more likely to achieve low disease activity at 24 weeks. These parameters should be considered as part of the clinical decision-making process when initiating MTX in DMARD-naïve patients with RA.References[1]Aletaha D, Smolen JS. Effectiveness profiles and dose dependent retention of traditional disease modifying antirheumatic drugs for rheumatoid arthritis. An observational study. J Rheumatol. 2002;29(8):1631-8.AcknowledgementsThis abstract is based on research using data from data contributors UCB and Roche that has been made available through Vivli, Inc. Vivli has not contributed to or approved, and is not in any way responsible for, the contents of this publication.Disclosure of InterestsStephanie Duong: None declared, Cynthia S. Crowson: None declared, Arjun Athreya: None declared, Elizabeth Atkinson: None declared, John M Davis III Grant/research support from: Pfizer, Kenneth J Warrington Speakers bureau: Chemocentryx, Consultant of: Roche/Genentech, Eric Matteson: None declared, Richard Weinshilboum Shareholder of: OneOme, Liewei Wang Shareholder of: OneOme, Elena Myasoedova: None declared.
更多查看译文
关键词
methotrexate,rheumatoid arthritis,machine learning approach,machine learning,prediction
AI 理解论文
溯源树
样例
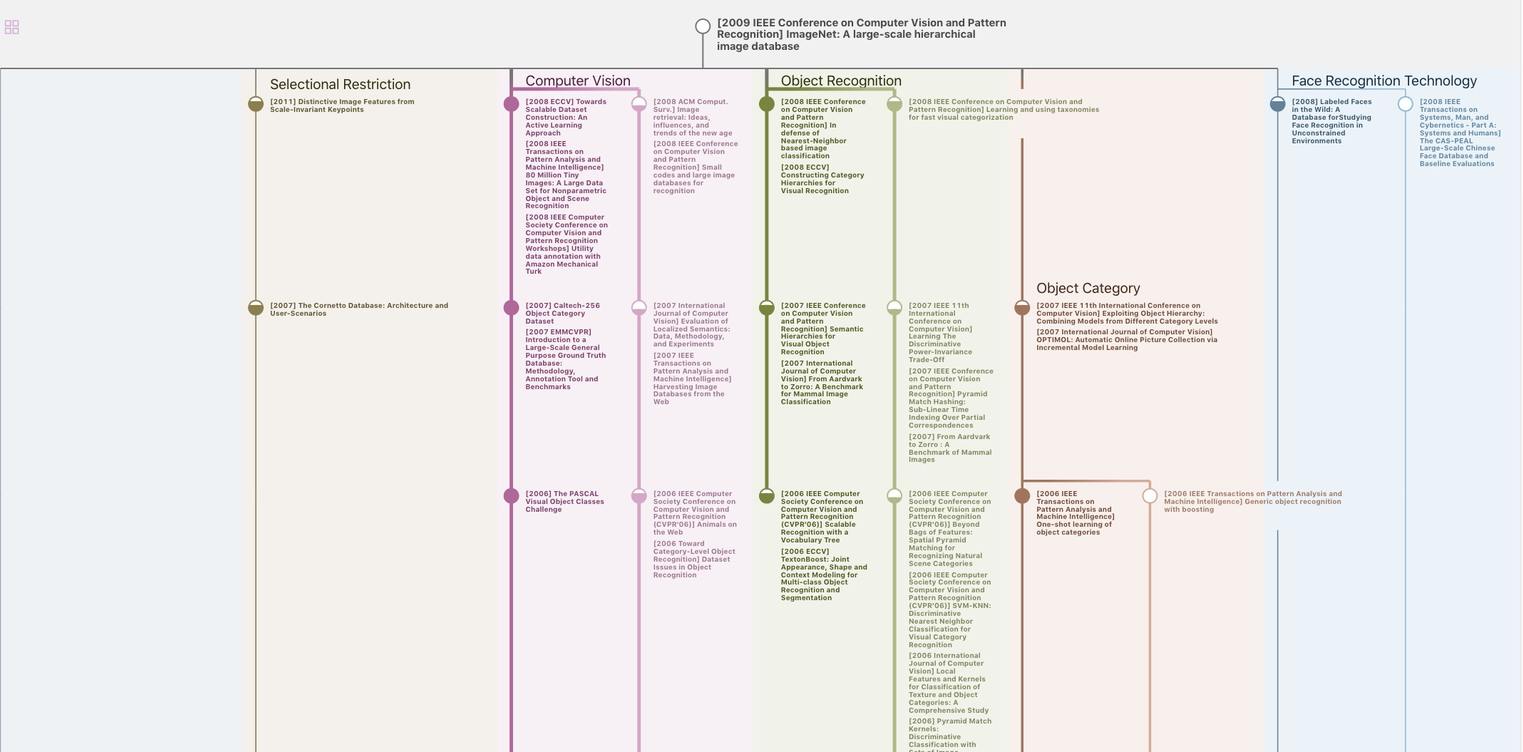
生成溯源树,研究论文发展脉络
Chat Paper
正在生成论文摘要