Toward Online Power System Model Identification: A Deep Reinforcement Learning Approach
IEEE Transactions on Power Systems(2022)
摘要
The rapidly increasing penetration of power electronic equipment has complicated the dynamic characteristics of modern power systems, which has promoted the development of simulation analysis methods for online dynamic security analysis (DSA) systems. Accurate models lay the foundation for online DSA, while the high complexity and low observability of power electronic equipment models hinder the online implementation of conventional model identification approaches. In this paper, we propose an online parameter identification (PI) framework based on deep reinforcement learning (DRL), suitable for power electronic equipment models. The DRL-based online PI formulation is proposed by integrating a model-based online PI and an improved DRL-based PI approach. On this basis, the framework for DRL-based online PI is constructed through tailored design of interfaces and training mechanisms. The DRL agent is able to learn the PI policy offline by constructing a training scenario database and the trained policy can be deployed to realize accurate online identification. Case studies showcase the application of the proposed method on a high-voltage direct current (HVDC) system. Simulation results demonstrate that the proposed method exhibits superior computational advantage, while achieving a similar level of accuracy compared to conventional methods.
更多查看译文
关键词
Data-driven method,deep reinforcement learning,HVDC,parameter identification,power electronic equipment
AI 理解论文
溯源树
样例
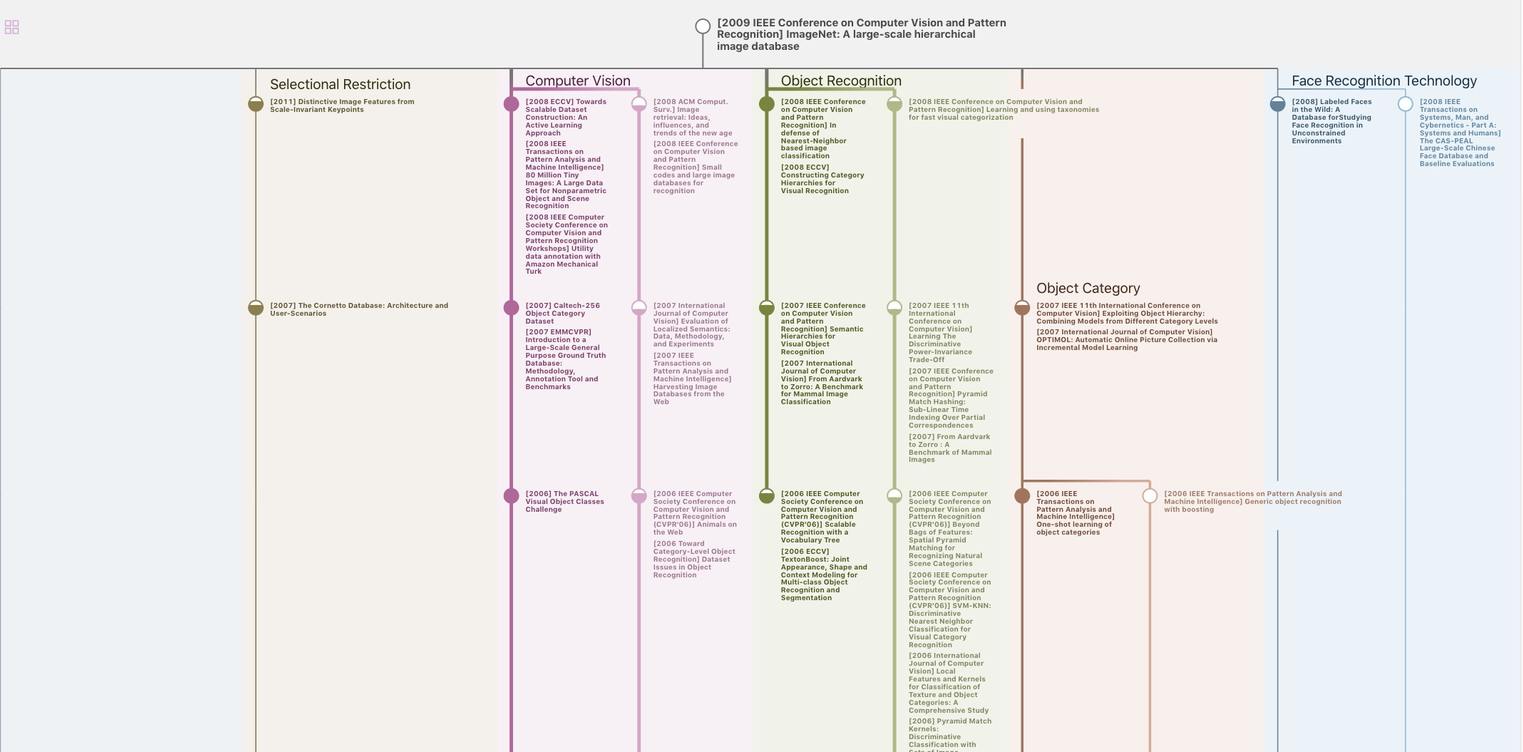
生成溯源树,研究论文发展脉络
Chat Paper
正在生成论文摘要