Registration of Multisensor Images through a Conditional Generative Adversarial Network and a Correlation-Type Similarity Measure
REMOTE SENSING(2022)
摘要
The automatic registration of multisensor remote sensing images is a highly challenging task due to the inherently different physical, statistical, and textural characteristics of the input data. Information-theoretic measures are often used to favor comparing local intensity distributions in the images. In this paper, a novel method based on the combination of a deep learning architecture and a correlation-type area-based functional is proposed for the registration of a multisensor pair of images, including an optical image and a synthetic aperture radar (SAR) image. The method makes use of a conditional generative adversarial network (cGAN) in order to address image-to-image translation across the optical and SAR data sources. Then, once the optical and SAR data are brought to a common domain, an area-based l(2) similarity measure is used together with the COBYLA constrained maximization algorithm for registration purposes. While correlation-type functionals are usually ineffective in the application to multisensor registration, exploiting the image-to-image translation capabilities of cGAN architectures allows moving the complexity of the comparison to the domain adaptation step, thus enabling the use of a simple l(2) similarity measure, favoring high computational efficiency, and opening the possibility to process a large amount of data at runtime. Experiments with multispectral and panchromatic optical data combined with SAR images suggest the effectiveness of this strategy and the capability of the proposed method to achieve more accurate registration as compared to state-of-the-art approaches.
更多查看译文
关键词
multisensor image registration,conditional generative adversarial networks (cGANs),deep learning,image-to-image translation,area-based registration,l(2) similarity,COBYLA
AI 理解论文
溯源树
样例
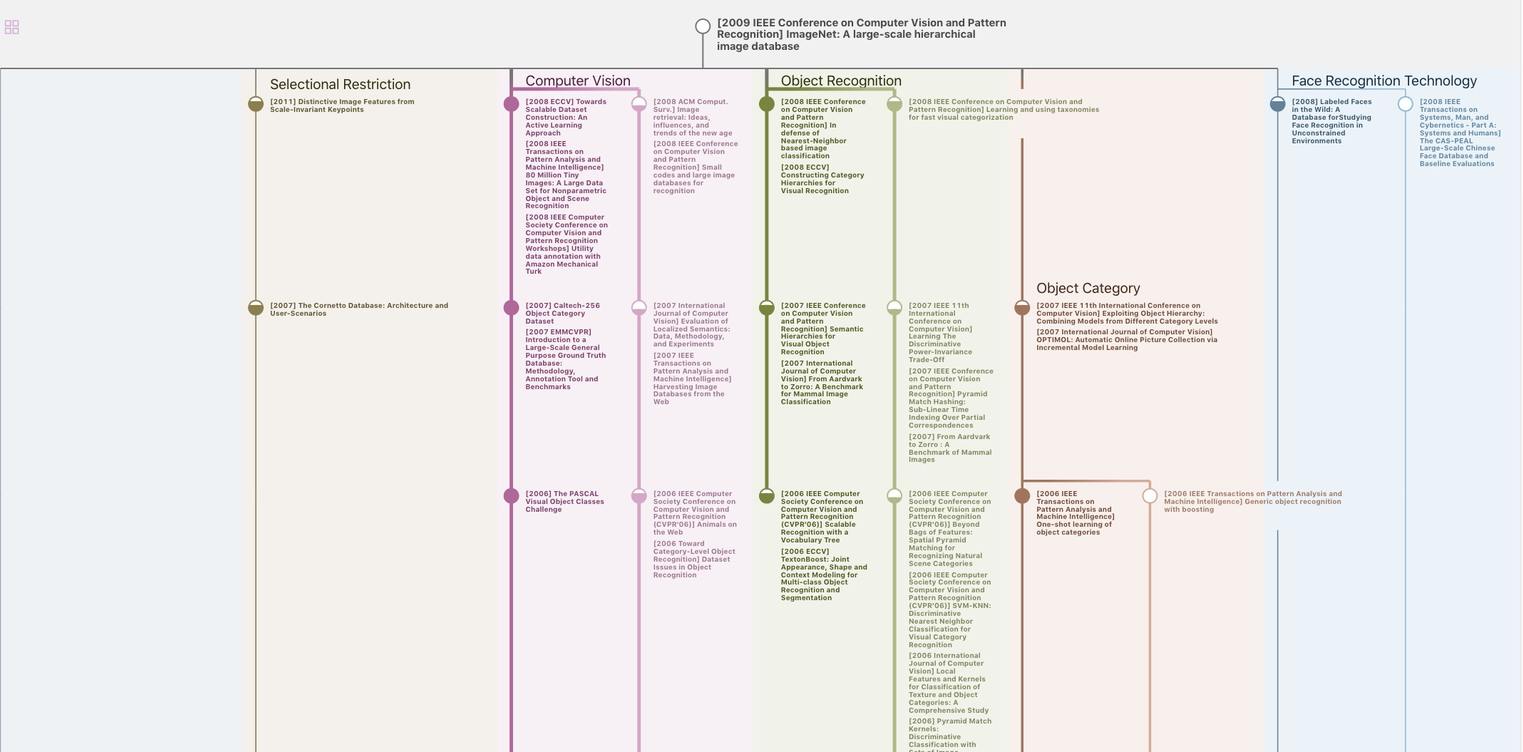
生成溯源树,研究论文发展脉络
Chat Paper
正在生成论文摘要