Abstract 4090: Creating research quality cancer genomic data from electronic health records
Cancer Research(2022)
摘要
Abstract Background: Understanding the impact of precision medicine on medical practice, patient care, and clinical outcomes is a priority for advancing cancer care. With the recent dramatic increase in the use of tumor genomic testing (TGT), records within EHRs are a rich data source for evaluating the impact of TGT results in real-world clinical practice of care and on patient outcomes. However, extracting TGT results from electronic health records (EHR) is challenging due to a lack of standards to communicate genomic information and an inability to store such information in commonly available EHR systems. Moreover, TGT results are delivered to clinicians in unstructured formats and image-based files (PDFs). We initiated a pilot study to assess the ability of natural language processing (NLP) algorithms to convert EHR unstructured clinical text and PDF-formatted TGT results into research-quality data. Methods: One author (RY) drew a sample of approximately 800 clinical text records from 21 breast cancer patients treated at University of Washington. Sources used for data extraction included medical record notes and PDF reports for two breast cancer gene expression tests: 21-gene Recurrence Score (RS, OncotypeDx) and/or the 70-gene signature (MMP, Mammaprint). A team redacted all PHI and provided records to a commercial collaborator (Pangaeadata.AI, UK), along with definitions of variables to be extracted, but without annotated target answers. Existing NLP algorithms that leverage pre-training, fine-tuning and rules were adapted to extract 26 variables specified by the research team (e.g., age at diagnosis, histology, and RS or MMP dates and scores). The output placed variables into relevant, standardized formats and produced a research quality data set. The extraction strategy depended on the feature and variable characteristics. For example, cancer stage, an ordinal numerical variable, was determined with a rule-based extraction method from outpatient clinic notes and pathology reports, whereas the RS score, a continuous variable, came from OncotypeDx PDF and OCR semi-structured retrieval produced the output. Results/Conclusions: The Pangaea tool obtained an average accuracy of 97.3% with a standard deviation of 3.5% across all 26 variables. The approach is developed based on rules designed and validated by clinical experts, using a model that does not require training, making overfitting likely minimal. Qualitative analysis showed that: 1] algorithms used to electronically extract TGT results provided the same data as manual abstraction by physicians, and 2] context matters, namely, the capability of preliminary semantic understanding in the Pangaea model using contextual words and phrases contributed to high accuracy and can be generalized further with larger datasets. Expansion to other health care data systems is needed to assess scalability of these technologies to create research-quality data fit for use. Citation Format: Rachel Yung, Kari A. Stephens, Meliha Yetisgen, Andrea Burnett-Hartman, Ashwani Tanwar, Guilherme Freire, Atri Sharma, Jingqing Zhang, Vibhor Gupta, Yike Guo, VK Gadi, Larry Kessler. Creating research quality cancer genomic data from electronic health records [abstract]. In: Proceedings of the American Association for Cancer Research Annual Meeting 2022; 2022 Apr 8-13. Philadelphia (PA): AACR; Cancer Res 2022;82(12_Suppl):Abstract nr 4090.
更多查看译文
关键词
genomic data,electronic health records,cancer
AI 理解论文
溯源树
样例
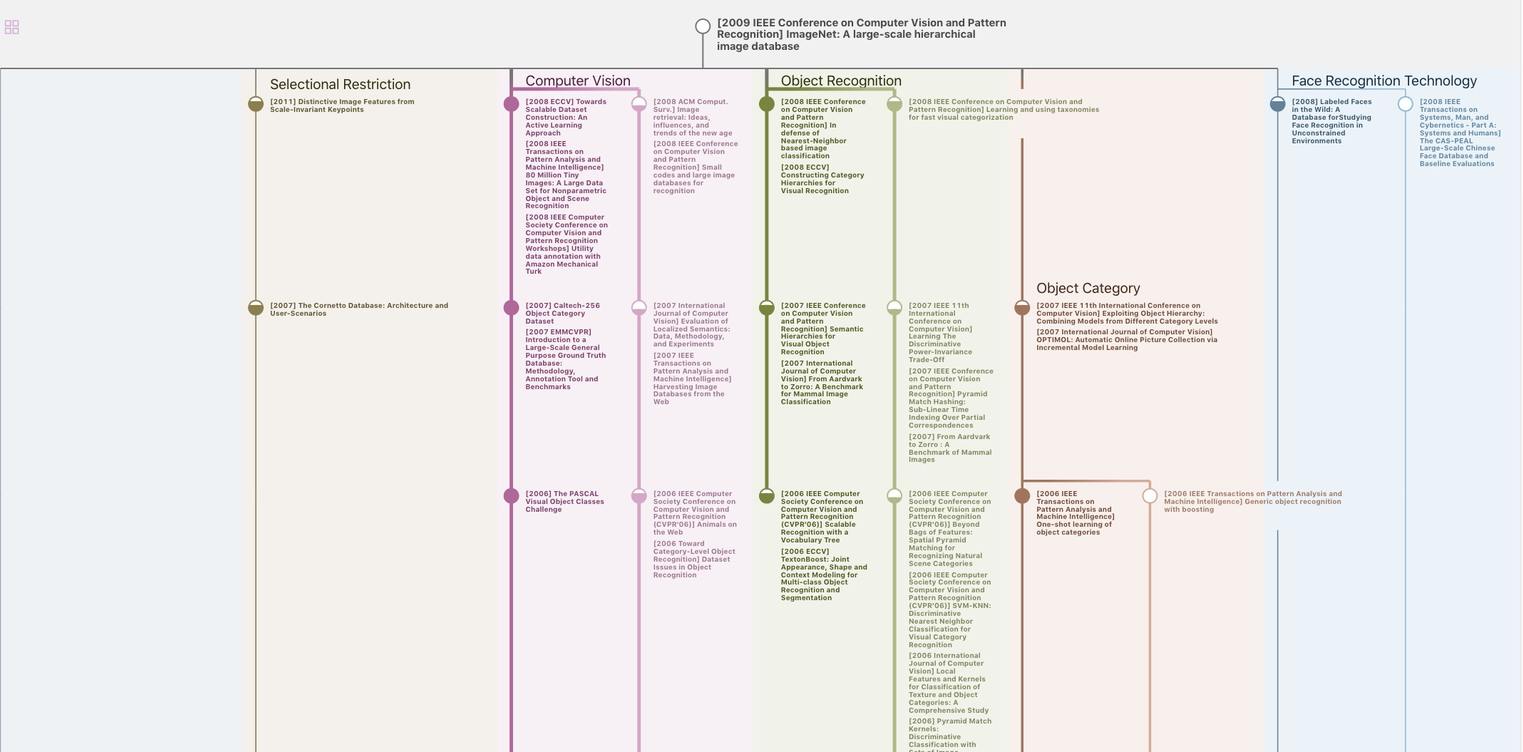
生成溯源树,研究论文发展脉络
Chat Paper
正在生成论文摘要