Robust artificial intelligence-powered imaging biomarker based on mammography for risk prediction of breast cancer.
Journal of Clinical Oncology(2022)
摘要
10533 Background: Accurate risk assessment allows the precise personalized screening of breast cancer. Conventional statistical risk models have been used that estimate the probability of breast cancer incidence with patient demographics, detailed personal and family history. The purpose of this study was to investigate the feasibility of the AI-powered Imaging Biomarker in mammography (IBM) which was developed to predict the risk of future breast cancer beyond the mammographic breast density. Methods: We trained and developed the deep learning risk model, the AI-powered IBM, using a total of 36,955 examinations from 21,438 patients, who underwent at least one mammogram using Hologic or Siemens machines and pathology-confirmed breast cancer outcomes. To discover the feasibility of the AI-powered IBM, mammograms and various clinical information including pathology-confirmed breast cancer outcomes were collected, which were only used for external validation. C-indices and receiver operating characteristic (ROC) curves for 1- to 5-year outcomes were obtained. We compared 5-year ROC area under the curves (AUCs) of our AI-powered IBM and statistical risk models including the Tyrer-Cuzick model and the Gail model, which were most commonly used and widely known, using DeLong’s test. Results: A total of 16,894 mammograms were collected for external validation, of which 4,002 were followed by a cancer diagnosis within 5 years. Our AI-powered IBM obtained C-index of 0.758, and the model demonstrated the risk of breast cancer with AUC of 0.895 (95% CI: 0.880, 0.909) at 1-year, 0.839 (0.824, 0.852) at 2-year, 0.807 (0.794, 0.819) at 3-year, 0.783 (0.7947, 0.819) at 4-year. The 5-year AUC of our AI-powered IBM was 0.808 (0.792, 0.822). Our AI-powered IBM showed significantly higher 5-year AUC than the Gail model (AUC: 0.572, P< 0.001) and the Tyrer-Cuzick model (0.569, P< 0.001). Conclusions: A deep learning AI-powered IBM using mammograms has a substantial potential to advance toward the robust risk prediction of breast cancer over conventional risk models. This approach for risk stratification of breast cancer might be feasible to improve personalized screening programs.
更多查看译文
AI 理解论文
溯源树
样例
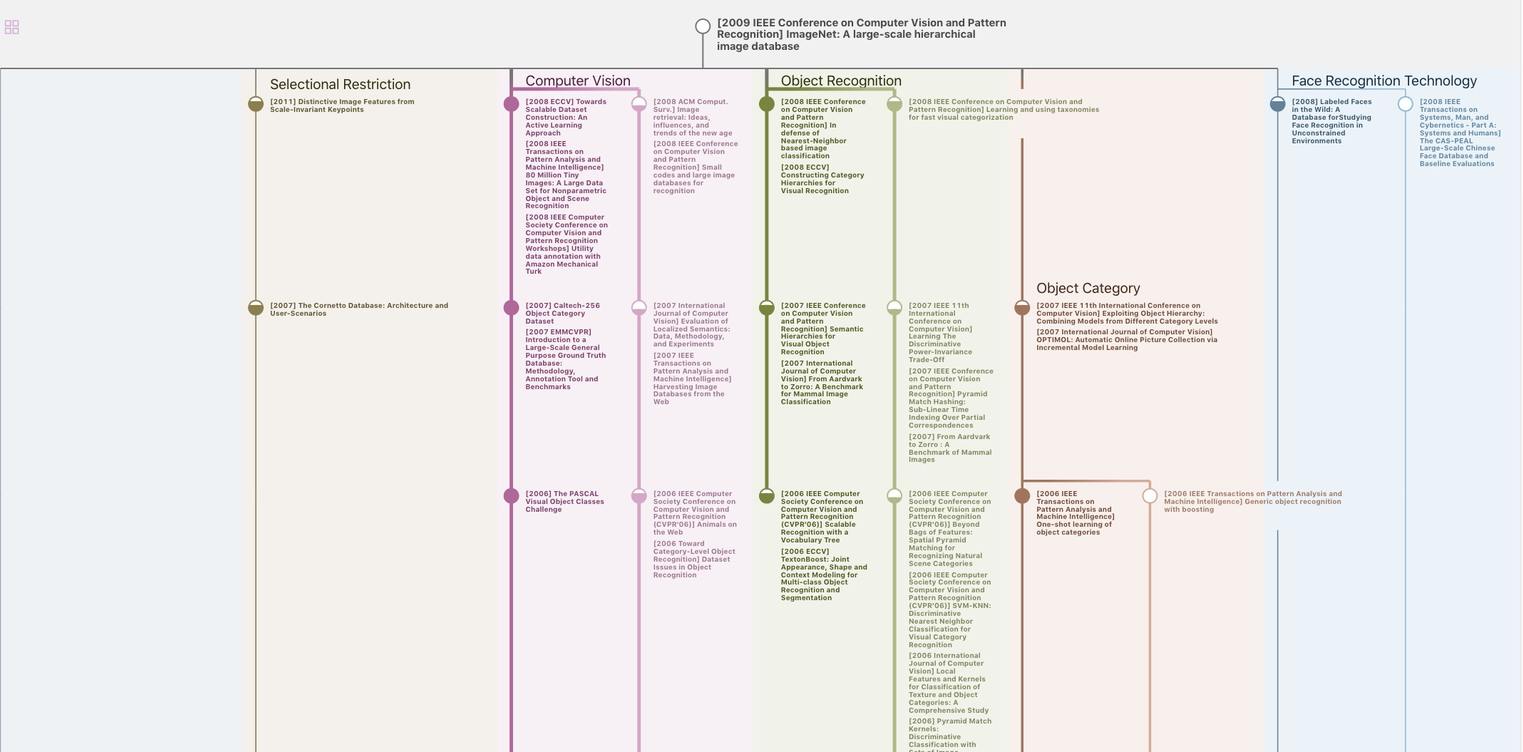
生成溯源树,研究论文发展脉络
Chat Paper
正在生成论文摘要