Abstract 1159: Predicting clinical drug responses using a few-shot learning-based interpretable AI
Cancer Research(2022)
摘要
Abstract High-throughput screens have generated large amounts of data characterizing how thousands of cell lines respond to hundreds of anti-cancer therapies. However, predictive drug response models trained using data from cell lines often fail to translate to clinical applications. Here, we focus on two key issues to improve clinical performance: 1) Transferability: the ability of predictive models to quickly adapt to clinical contexts even with a limited number of samples from patients, and 2) Interpretability: the ability to explain how drug-response predictions are being made given an individual patient’s genotype. Notably, an interpretable AI model can also help to identify biomarkers of treatment response in individual patients. By leveraging new developments in meta-learning and interpretable AI, we have developed an interpretable drug response prediction model that is trained on large amounts of data from experiments using cell lines and then transferred to clinical applications. We assessed our model’s clinical utility using AACR Project GENIE data, which contains mutational profiles from tumors and the patient’s therapeutic responses. We have demonstrated the feasibility of applying our AI-driven predictive model to clinical settings and shown how this model can support clinical decision making for tumor boards. Citation Format: Sungjoon Park, Akshat Singhal, Erica Silva, Jason F. Kreisberg, Trey Ideker. Predicting clinical drug responses using a few-shot learning-based interpretable AI [abstract]. In: Proceedings of the American Association for Cancer Research Annual Meeting 2022; 2022 Apr 8-13. Philadelphia (PA): AACR; Cancer Res 2022;82(12_Suppl):Abstract nr 1159.
更多查看译文
关键词
clinical drug responses,ai,few-shot,learning-based
AI 理解论文
溯源树
样例
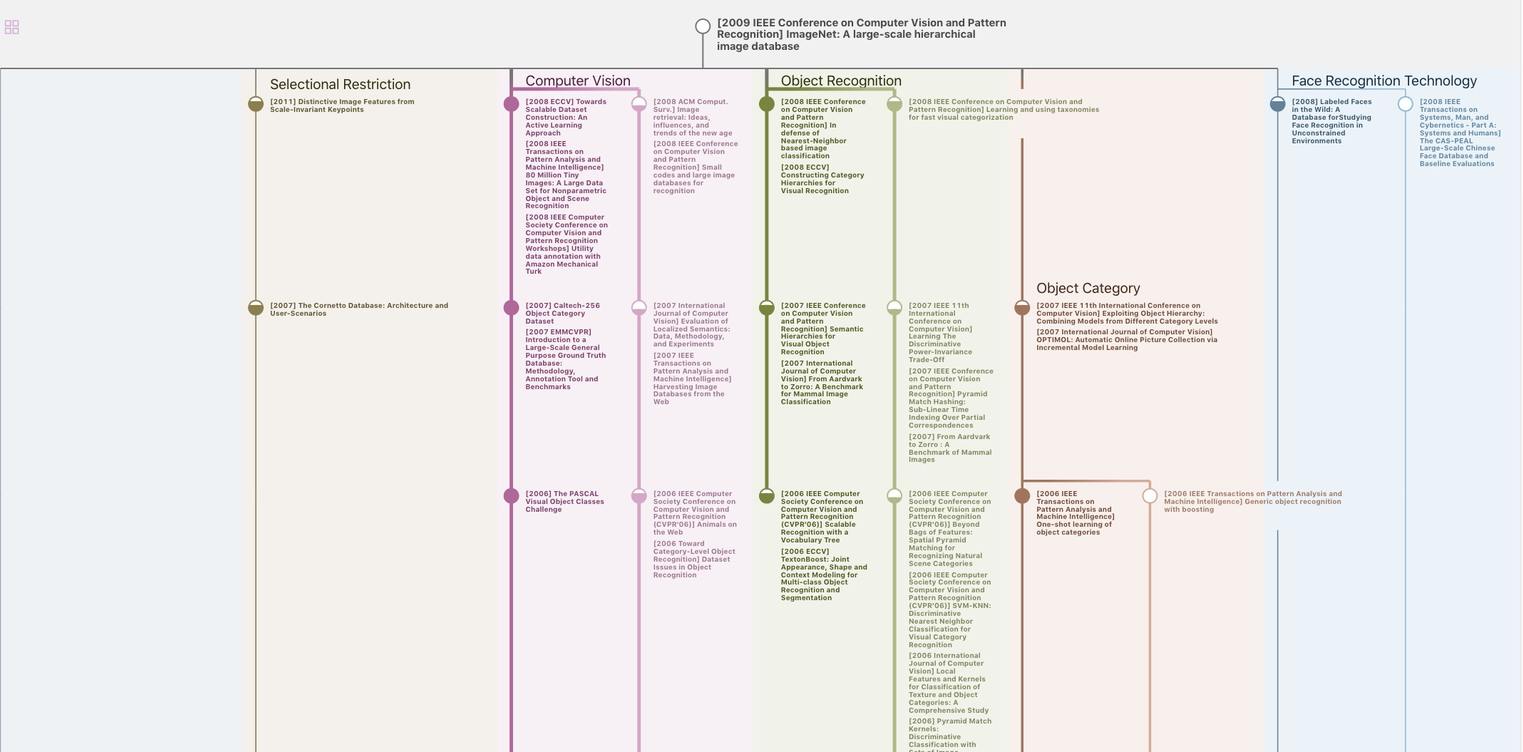
生成溯源树,研究论文发展脉络
Chat Paper
正在生成论文摘要