Shape encoding for semantic healing of design models and knowledge transfer to Scan-to-BIM
Proceedings of the Institution of Civil Engineers - Smart Infrastructure and Construction(2022)
摘要
Automated parsing of design data will increasingly be a prerequisite for efficient data- and analytics-driven management of building portfolios. The high complexity and low rigidity of building information modelling (BIM) model exchange standards such as Industry Foundation Classes result in considerable differences in data quality and impede direct data availability for analytics-based decision support. Mis- or unclassified building elements are a common issue and can lead to tedious manual reworks. At the same time, scan-to-BIM processes still require considerable manual effort to identify subclass element geometry. This work leverages the benefits of a three-dimensional lightweight, geometric algorithm to generate meaningful geometric features autonomously that assist shape classification in erroneous design models and pre-segmented point clouds. Geometric deep learning is introduced in two steps; a discussion about the benefits of graph convolutional networks (GCNs) is given before a set of experiments on BIM element data sets is conducted. Utilising explainable artificial intelligence methods, the GCN performance is made suitable for human–algorithm interaction. Leveraging element geometry solely, the classification reaches a promising average performance of above 83% for the model-healing task with a reduced computation time. The encoded geometric knowledge from the design models is shown to be helpful in showcasing examples of segment classification in point clouds.
更多查看译文
关键词
shape encoding,design models,semantic healing,knowledge transfer,scan-to-bim
AI 理解论文
溯源树
样例
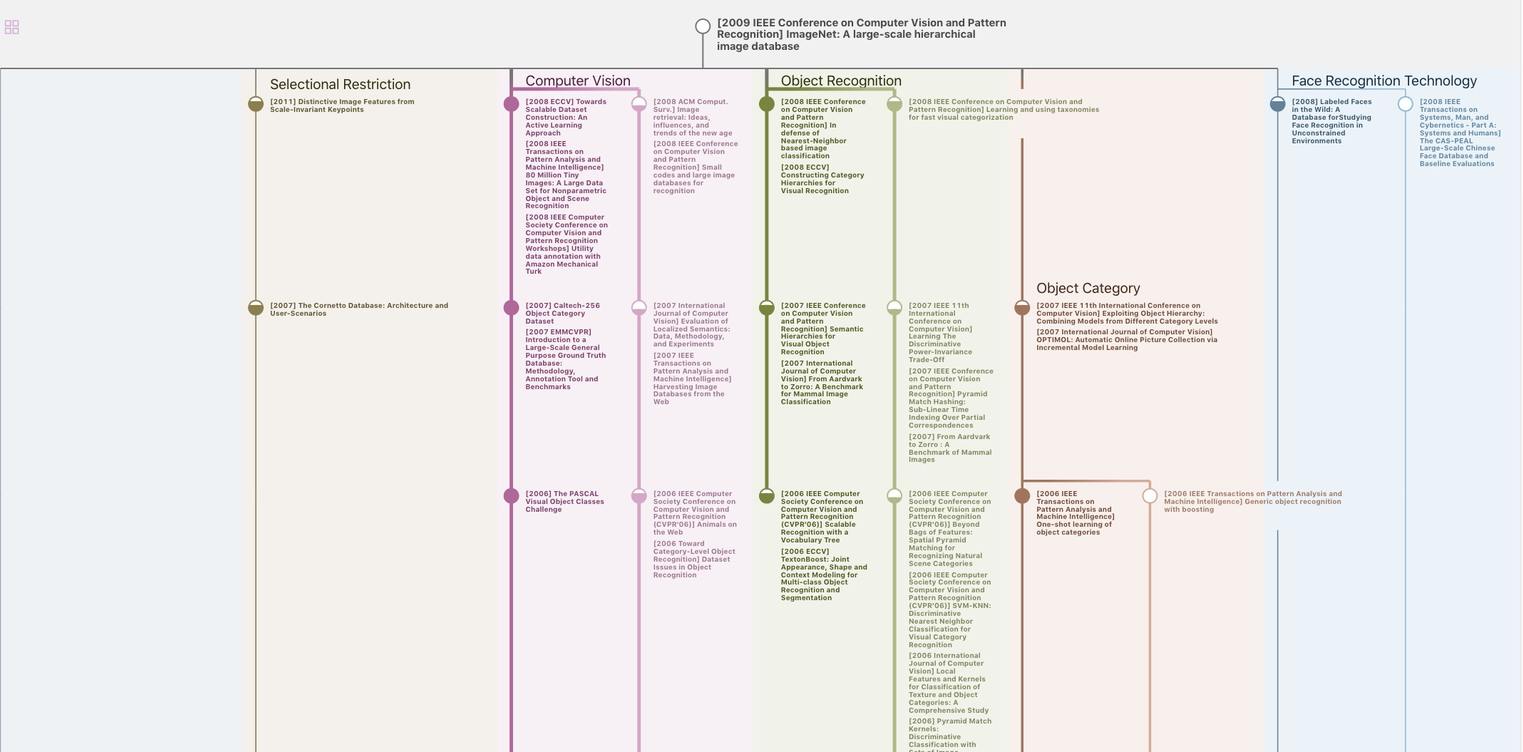
生成溯源树,研究论文发展脉络
Chat Paper
正在生成论文摘要