CE-Fed: Communication efficient multi-party computation enabled federated learning
Array(2022)
摘要
Federated learning (FL) allows a number of parties collectively train models without revealing private datasets. There is a possibility of extracting personal or confidential data from the shared models even-though sharing of raw data is prevented by federated learning. Secure Multi Party Computation (MPC) is leveraged to aggregate the locally-trained models in a privacy preserving manner. However, it results in high communication cost and poor scalability in a decentralized environment. We design a novel communication-efficient MPC enabled federated learning called CE-Fed. In particular, the proposed CE-Fed is a hierarchical mechanism which forms model aggregation committee with a small number of members and aggregates the global model only among committee members, instead of all participants. We develop a prototype and demonstrate the effectiveness of our mechanism with different datasets. Our proposed CE-Fed achieves high accuracy, communication efficiency and scalability without compromising privacy.
更多查看译文
关键词
Federated learning,Edge computing,Multi-party computation,Committee selection
AI 理解论文
溯源树
样例
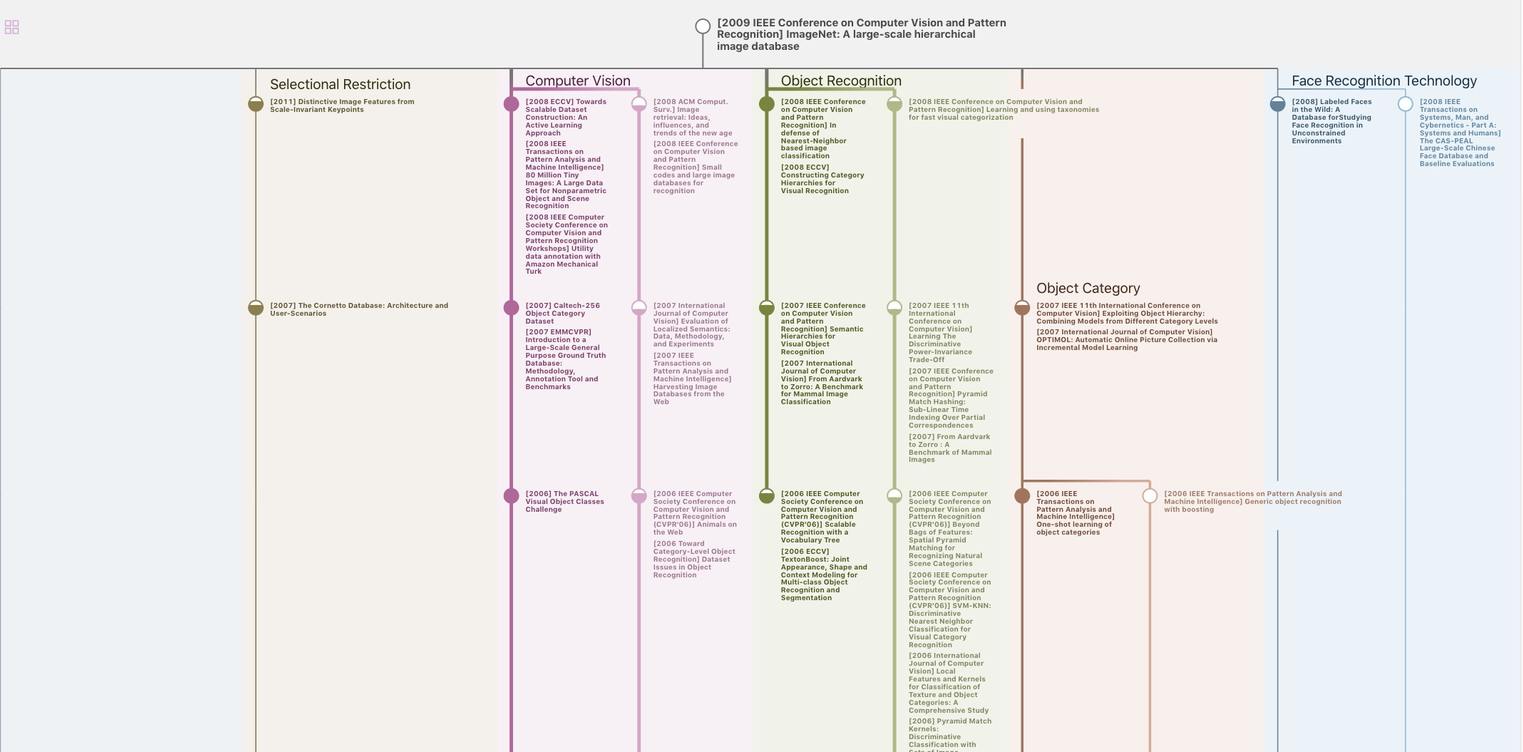
生成溯源树,研究论文发展脉络
Chat Paper
正在生成论文摘要