Bayesian model selection for multilevel models using marginal likelihoods
PloS one(2022)
摘要
Multilevel linear models allow flexible statistical modelling of complex data with different levels of stratification. Identifying the most appropriate model from the large set of possible candidates is a challenging problem. In the Bayesian setting, the standard approach is a comparison of models using the model evidence or the Bayes factor. However, in all but the simplest of cases, direct computation of these quantities is impossible. Markov Chain Monte Carlo approaches are widely used, such as sequential Monte Carlo, but it is not always clear how well such techniques perform in practice. We present an improved method for estimation of the log model evidence, by an intermediate analytic computation of a marginal likelihood, integrated over non-variance parameters. This reduces the dimensionality of the Monte Carlo sampling algorithm, which in turn yields more consistent estimates. We illustrate this method on a popular multilevel dataset containing levels of radon in homes in the US state of Minnesota.
更多查看译文
关键词
multilevel models,likelihoods,selection
AI 理解论文
溯源树
样例
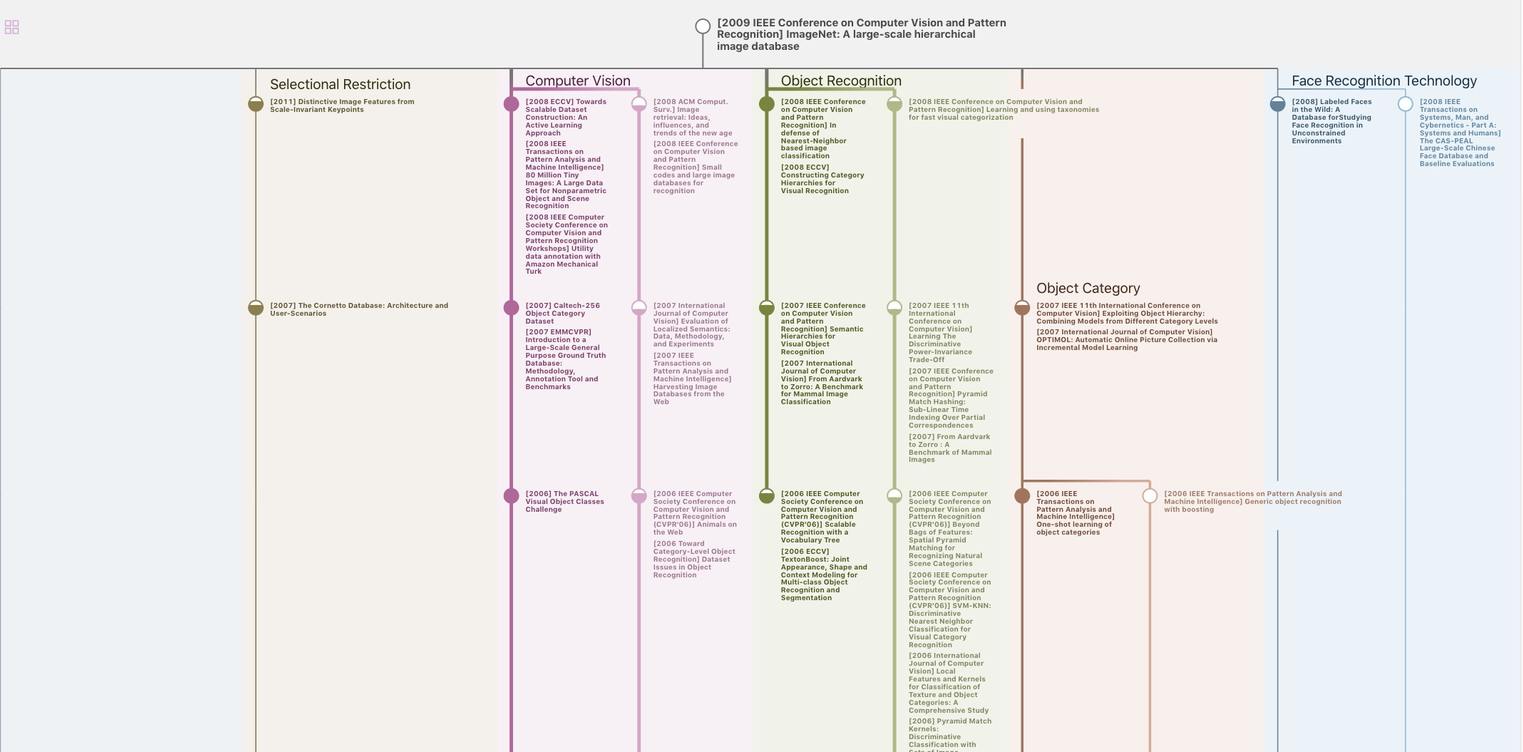
生成溯源树,研究论文发展脉络
Chat Paper
正在生成论文摘要