Multi-scored sleep databases: How to exploit the multiple-labels in automated sleep staging
arxiv(2022)
摘要
Study Objectives: Inter-scorer variability in scoring polysomnograms is a well-known problem. Most of the existing automated sleep scoring systems are trained using labels annotated by a single scorer, whose subjective evaluation is transferred to the model. When annotations from two or more scorers are available, the scoring models are usually trained on the scorer consensus. The averaged scorer's subjectivity is transferred into the model, losing information about the internal variability among different scorers. In this study, we aim to insert the multiple-knowledge of the different physicians into the training procedure. The goal is to optimize a model training, exploiting the full information that can be extracted from the consensus of a group of scorers. Methods: We train two lightweight deep learning based models on three different multi-scored databases. We exploit the label smoothing technique together with a soft-consensus (LSSC) distribution to insert the multiple-knowledge in the training procedure of the model. We introduce the averaged cosine similarity metric (ACS) to quantify the similarity between the hypnodensity-graph generated by the models with-LSSC and the hypnodensity-graph generated by the scorer consensus. Results: The performance of the models improves on all the databases when we train the models with our LSSC. We found an increase in ACS (up to 6.4%) between the hypnodensity-graph generated by the models trained with-LSSC and the hypnodensity-graph generated by the consensus. Conclusion: Our approach definitely enables a model to better adapt to the consensus of the group of scorers. Future work will focus on further investigations on different scoring architectures and hopefully large-scale-heterogeneous multi-scored datasets.
更多查看译文
关键词
automatic sleep stage classification,machine learning,deep learning,multi-scored sleep databases
AI 理解论文
溯源树
样例
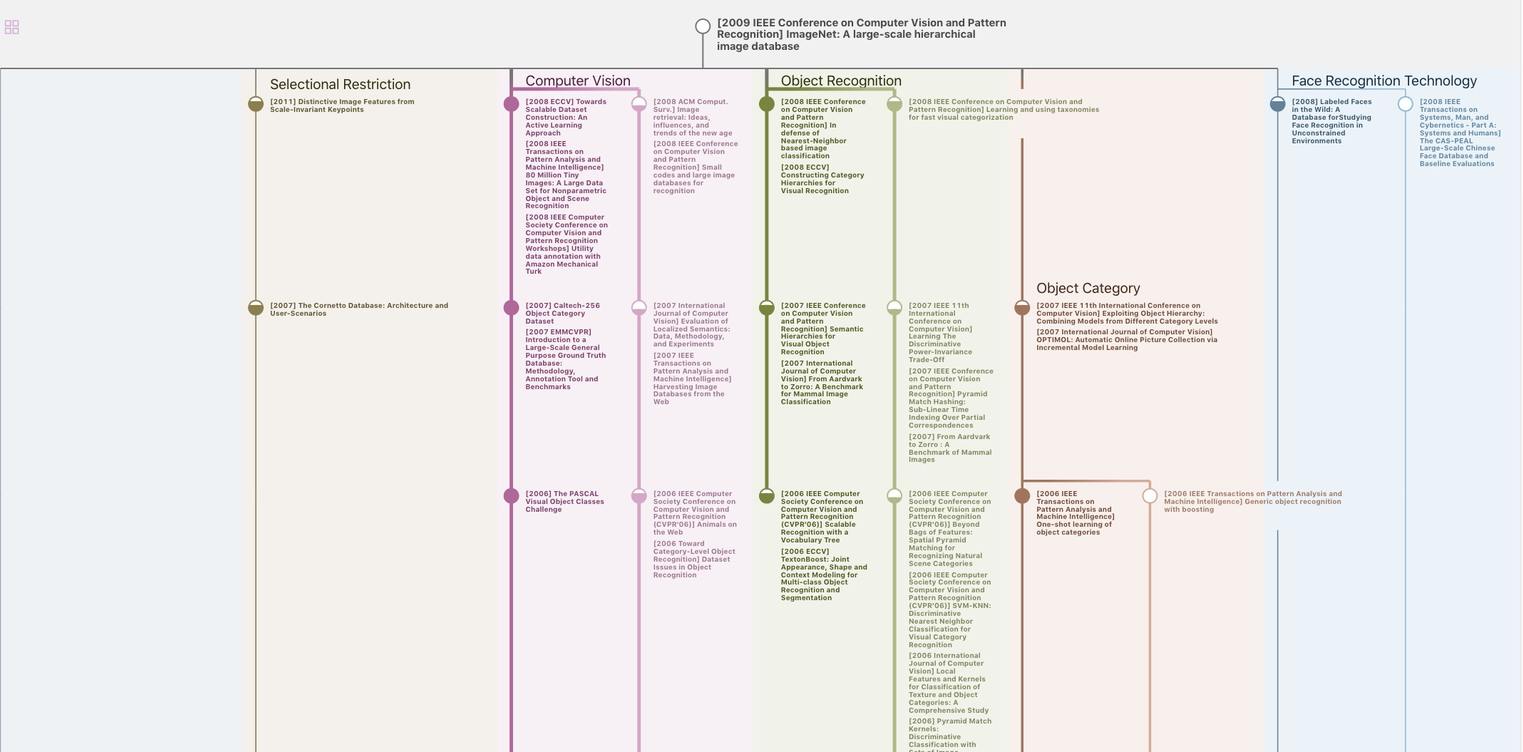
生成溯源树,研究论文发展脉络
Chat Paper
正在生成论文摘要