Deriving Surface Resistivity from Polarimetric SAR Data Using Dual-Input UNet
arxiv(2022)
摘要
Traditional survey methods for finding surface resistivity are time-consuming and labor intensive. Very few studies have focused on finding the resistivity/conductivity using remote sensing data and deep learning techniques. In this line of work, we assessed the correlation between surface resistivity and Synthetic Aperture Radar (SAR) by applying various deep learning methods and tested our hypothesis in the Coso Geothermal Area, USA. For detecting the resistivity, L-band full polarimetric SAR data acquired by UAVSAR were used, and MT (Magnetotellurics) inverted resistivity data of the area were used as the ground truth. We conducted experiments to compare various deep learning architectures and suggest the use of Dual Input UNet (DI-UNet) architecture. DI-UNet uses a deep learning architecture to predict the resistivity using full polarimetric SAR data by promising a quick survey addition to the traditional method. Our proposed approach accomplished improved outcomes for the mapping of MT resistivity from SAR data.
更多查看译文
AI 理解论文
溯源树
样例
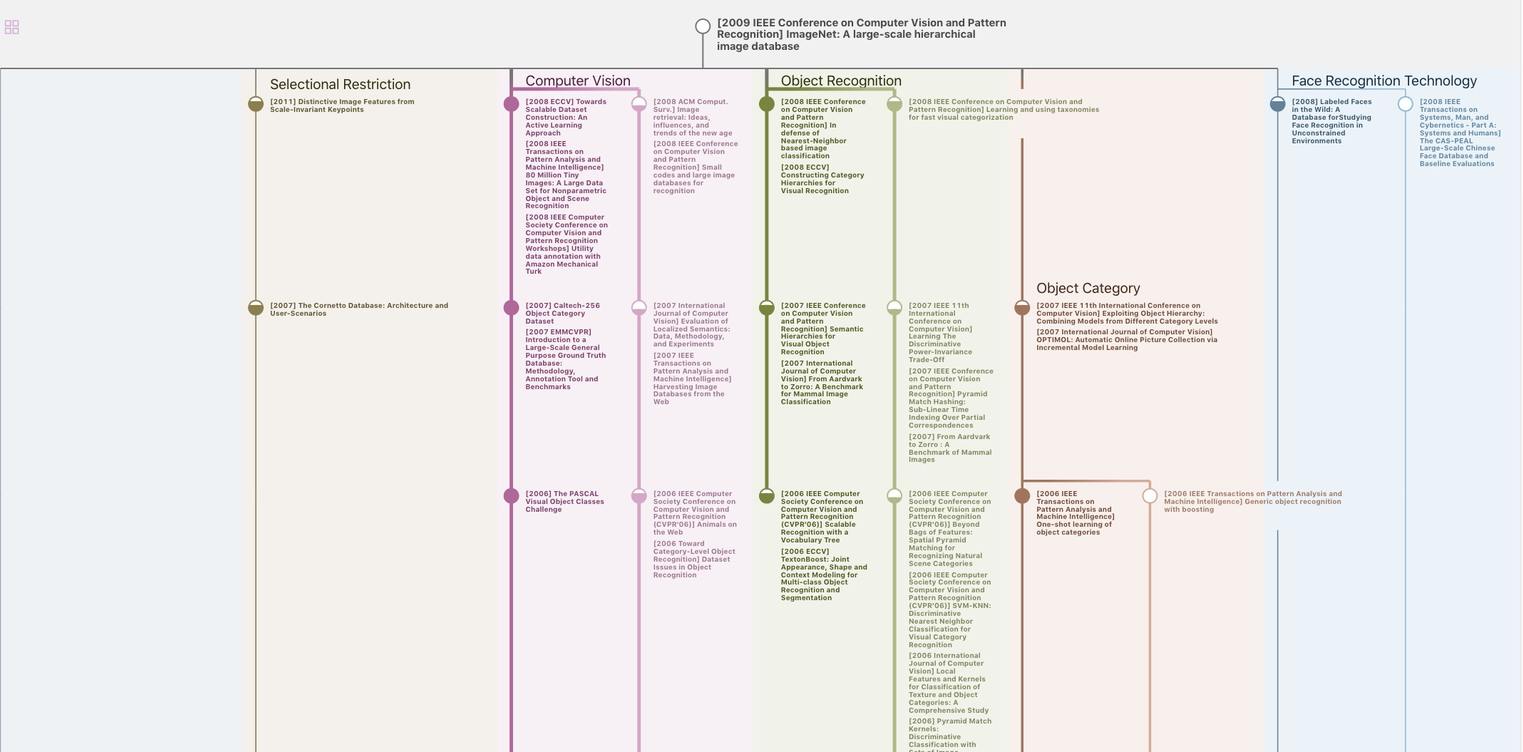
生成溯源树,研究论文发展脉络
Chat Paper
正在生成论文摘要