Predicting the abundance of metal resistance genes in subtropical estuaries using amplicon sequencing and machine learning
ECOTOXICOLOGY AND ENVIRONMENTAL SAFETY(2022)
摘要
Heavy metals are a group of anthropogenic contaminants in estuary ecosystems. Bacteria in estuaries counteract the highly concentrated metal toxicity through metal resistance genes (MRGs). Presently, metagenomic technology is popularly used to study MRGs. However, an easier and less expensive method of acquiring MRG information is needed to deepen our understanding of the fate of MRGs. Thus, this study explores the feasibility of using a machine learning approach-namely, random forests (RF)-to predict MRG abundance based on the 16S rRNA amplicon sequenced datasets from subtropical estuaries in China. Our results showed that the total MRG abundance could be predicted by RF models using bacterial composition at different taxonomic levels. Among them, the relative abundance of bacterial phyla had the highest predicted accuracy (71.7 %). In addition, the RF models constructed by bacterial phyla predicted the abundance of six MRG types and nine MRG subtypes with substantial accuracy (R-2 > 0.600). Five bacterial phyla (Firmicutes, Bacteroidetes, Patescibacteria, Armatimonadetes, and Nitrospirae) substantially determined the variations in MRG abundance. Our findings prove that RF models can predict MRG abundance in South China estuaries during the wet season by using the bacterial composition obtained by 16S rRNA amplicon sequencing.
更多查看译文
关键词
Amplicon sequencing,Estuary,Machine learning,Metal resistance gene,Random forests
AI 理解论文
溯源树
样例
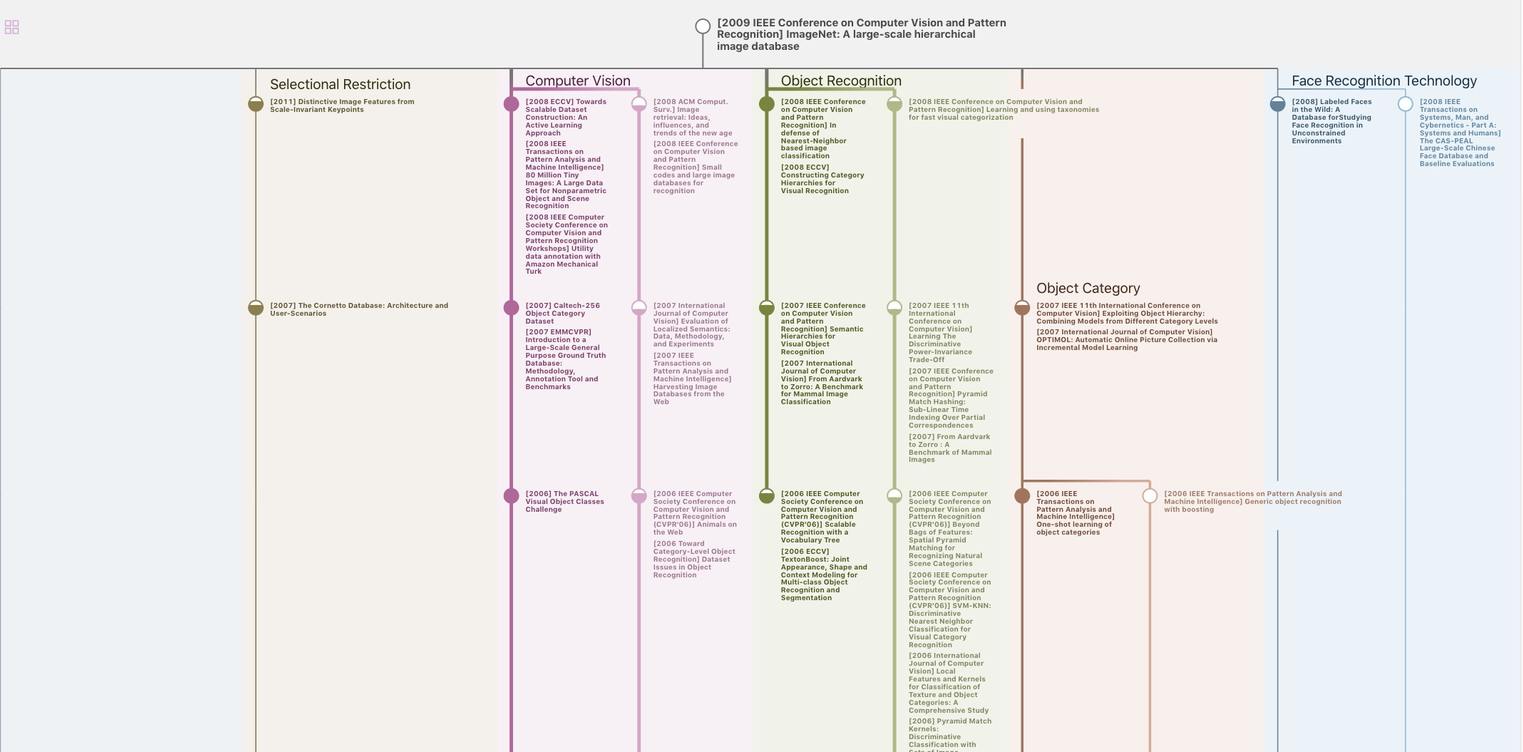
生成溯源树,研究论文发展脉络
Chat Paper
正在生成论文摘要