Optimizing cellulase production from Aspergillus flavus using response surface methodology and machine learning models
ENVIRONMENTAL TECHNOLOGY & INNOVATION(2022)
摘要
The study aims to optimize cellulase (CMCase) production by Aspergillus flavus using wheat straw, an abundantly available lignocellulosic waste, as a substrate. Three pa-rameters, i.e., nitrogen content (0.25 to 1%), fungal inoculum (0.25 to 1%), and duration (3 to 12 days), were optimized for maximum CMCase production using Response surface methodology-Box Behnken design (RSM-BBD). The quadratic response surface was suitable, and the model was significant. However, higher-order machine learning (ML) models were applied as the RSM-BBD model had a low R2 value (0.85) and negative predicted R2 value (-0.82). The supervised ML regression models, i.e., Artificial neural network (ANN) with Bayesian Regularization Neural Network (BRNN) and Radial Basis function Neural Network (RBFNN), Support vector machine (SVM) with Polynomial kernel (SPK), and Gaussian kernel (SGK), and Gaussian process learner (GPL) with the exponential kernel (GEK) and squared exponential kernel (GSEK) were applied. The RBFNN was the best performing model with a mean squared error (MSE) value of 0.0025 and an R2 value of 0.98. The maximum CMCase production of 13.89 U/gds was at yeast extract 0.25%, fungal inoculum 0.625%, and duration of 12 days. There was almost a threefold increase in CMCase production after optimization compared to the screening experiments (4.7 U/gds). (c)(c) 2022 Published by Elsevier B.V. This is an open access article under the CC BY-NC-ND license (http://creativecommons.org/licenses/by-nc-nd/4.0/).
更多查看译文
关键词
Artificial neural network,Cellulases,Gaussian process learner,Optimization,Response surface methodology,Support vector machine
AI 理解论文
溯源树
样例
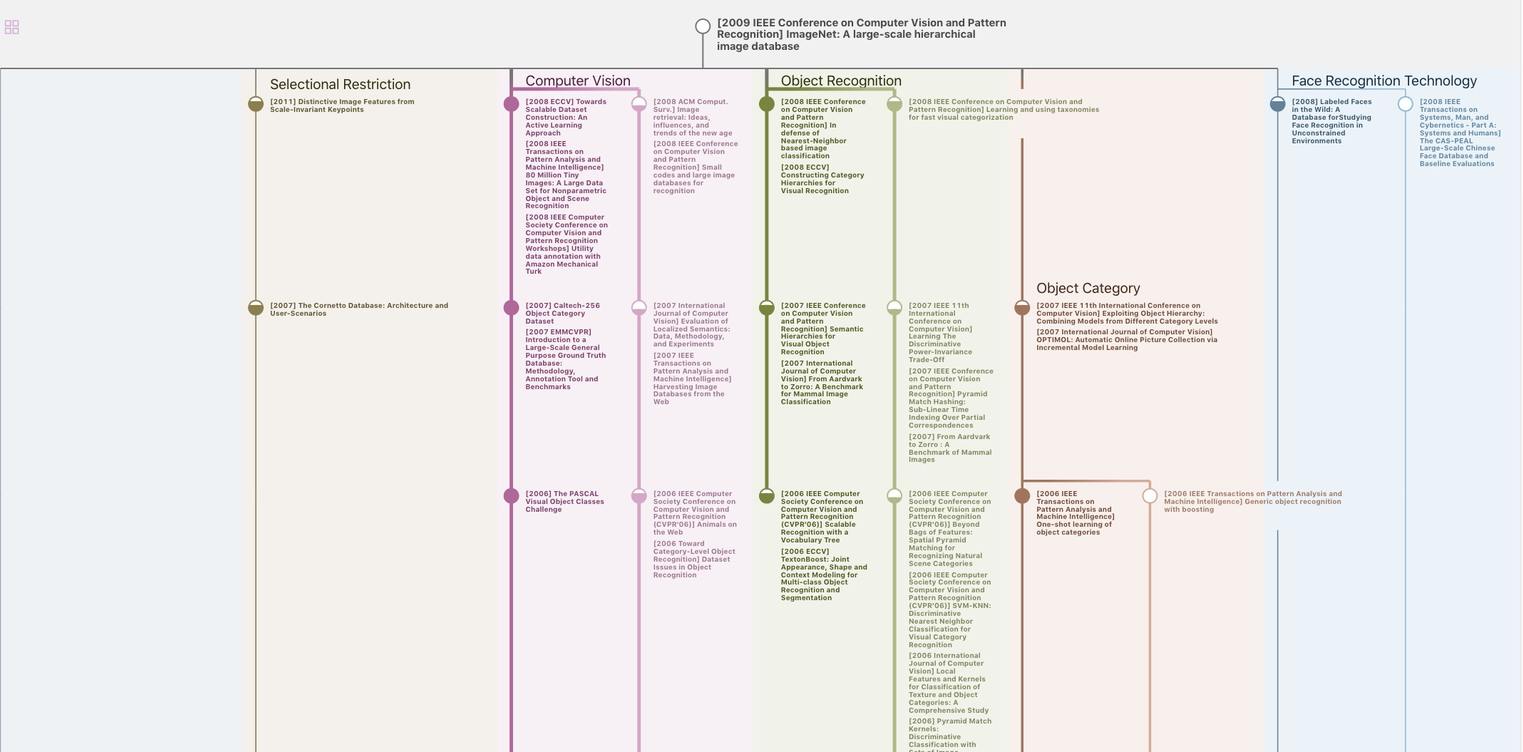
生成溯源树,研究论文发展脉络
Chat Paper
正在生成论文摘要