Classifying tumor brain images using parallel deep learning algorithms
Computers in Biology and Medicine(2022)
摘要
One of the most important resources used in today's world is image. Medical images can play an essential role in helping diagnose diseases. Doctors and specialists use medical images to diagnose brain diseases. Convolution neural networks are among the most important deep learning methods in which several layers are trained powerfully. The proposed method is a deep parallel convolution neural network model consisting of AlexNet and VGGNet networks. The structure of the layers is different for the two types of networks. The features are combined at one point and then categorized using the softmax function. To test the network, we used different criteria with images in the database. In clinical diagnosis, generalizability means prediction for people from whom we have no observation. On this account, we did not include individuals' observations in training set in the test set. Compared to the existing models, the proposed model results show that our network has achieved better results. The best result for the database was FIGSHARE, which achieved 99.14% accuracy on binary class and 98.78% on multi-class, which was much better than other SVM models. We tested the best available result with the existing database and compared the results with the proposed model, which was the best model answer for all evaluation criteria. These results suggest that the proposed model can be used as an effective decision support tool for radiologists in medical diagnosis.
更多查看译文
关键词
Medical image,Magnetic resonance imaging,Brain tumor classification,Parallel convolutional neural network
AI 理解论文
溯源树
样例
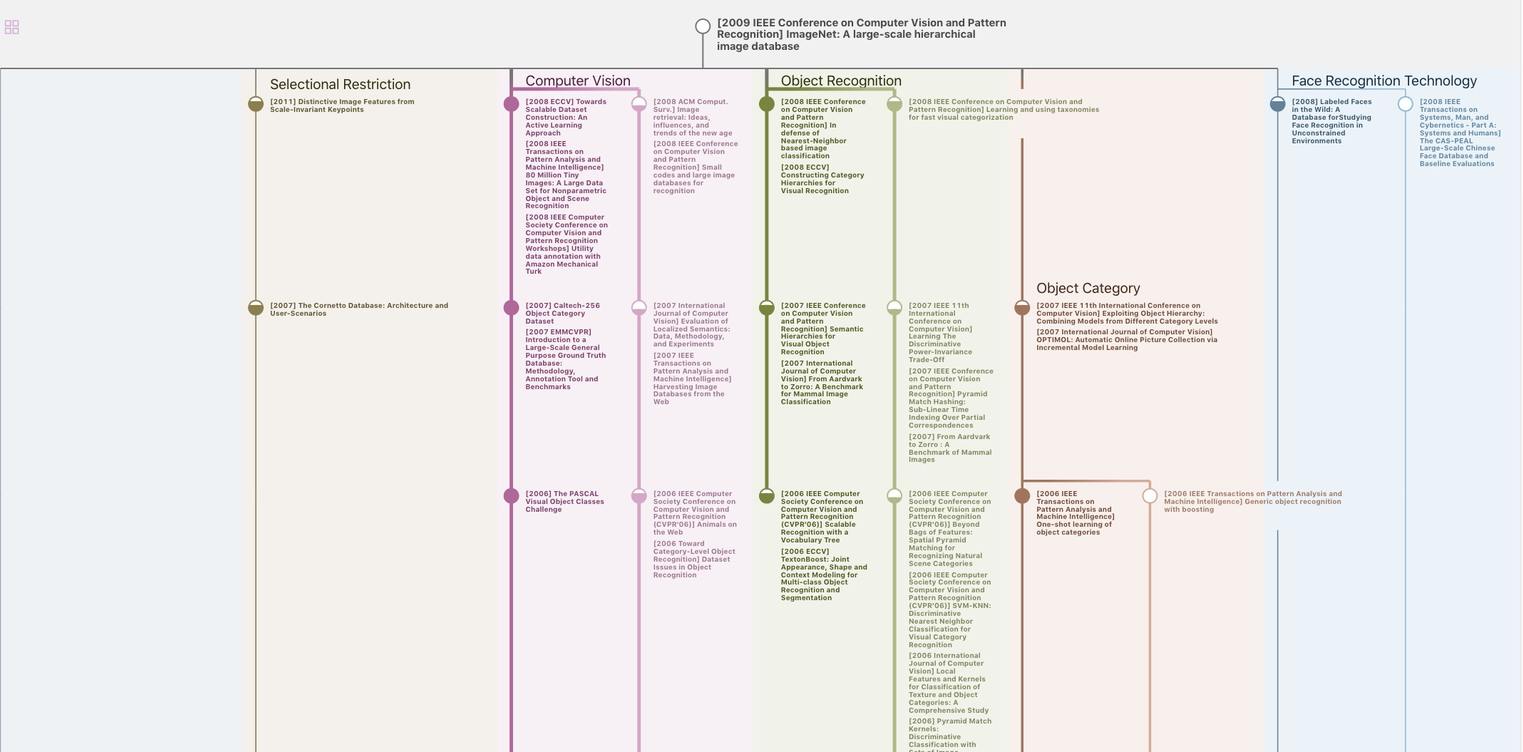
生成溯源树,研究论文发展脉络
Chat Paper
正在生成论文摘要