Back to MLP: A Simple Baseline for Human Motion Prediction
WACV(2023)
摘要
This paper tackles the problem of human motion prediction, consisting in forecasting future body poses from historically observed sequences. State-of-the-art approaches provide good results, however, they rely on deep learning architectures of arbitrary complexity, such as Recurrent Neural Networks (RNN), Transformers or Graph Convolutional Networks (GCN), typically requiring multiple training stages and more than 2 million parameters. In this paper, we show that, after combining with a series of standard practices, such as applying Discrete Cosine Transform (DCT), predicting residual displacement of joints and optimizing velocity as an auxiliary loss, a light-weight network based on multi-layer perceptrons (MLPs) with only 0.14 million parameters can surpass the state-of-the-art performance. An exhaustive evaluation on the Human3.6M, AMASS, and 3DPW datasets shows that our method, named SIMLPE, consistently outperforms all other approaches. We hope that our simple method could serve as a strong baseline for the community and allow re-thinking of the human motion prediction problem. The code is publicly available at https://github.com/dulucas/siMLPe.
更多查看译文
关键词
Algorithms: Biometrics,face,gesture,body pose,3D computer vision
AI 理解论文
溯源树
样例
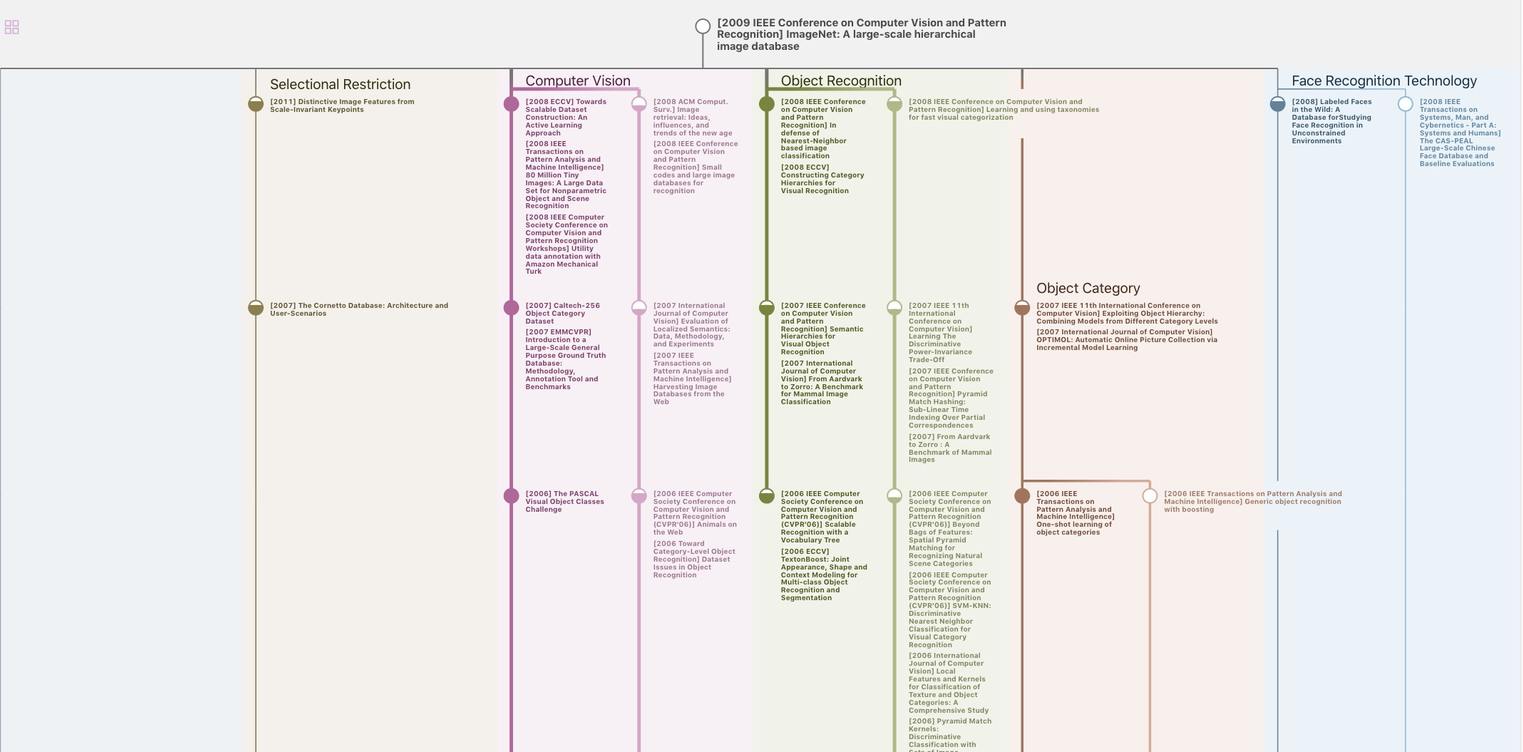
生成溯源树,研究论文发展脉络
Chat Paper
正在生成论文摘要