Comparing Feature Importance and Rule Extraction for Interpretability on Text Data
arxiv(2022)
摘要
Complex machine learning algorithms are used more and more often in critical tasks involving text data, leading to the development of interpretability methods. Among local methods, two families have emerged: those computing importance scores for each feature and those extracting simple logical rules. In this paper we show that using different methods can lead to unexpectedly different explanations, even when applied to simple models for which we would expect qualitative coincidence. To quantify this effect, we propose a new approach to compare explanations produced by different methods.
更多查看译文
关键词
interpretability,rule extraction,feature importance
AI 理解论文
溯源树
样例
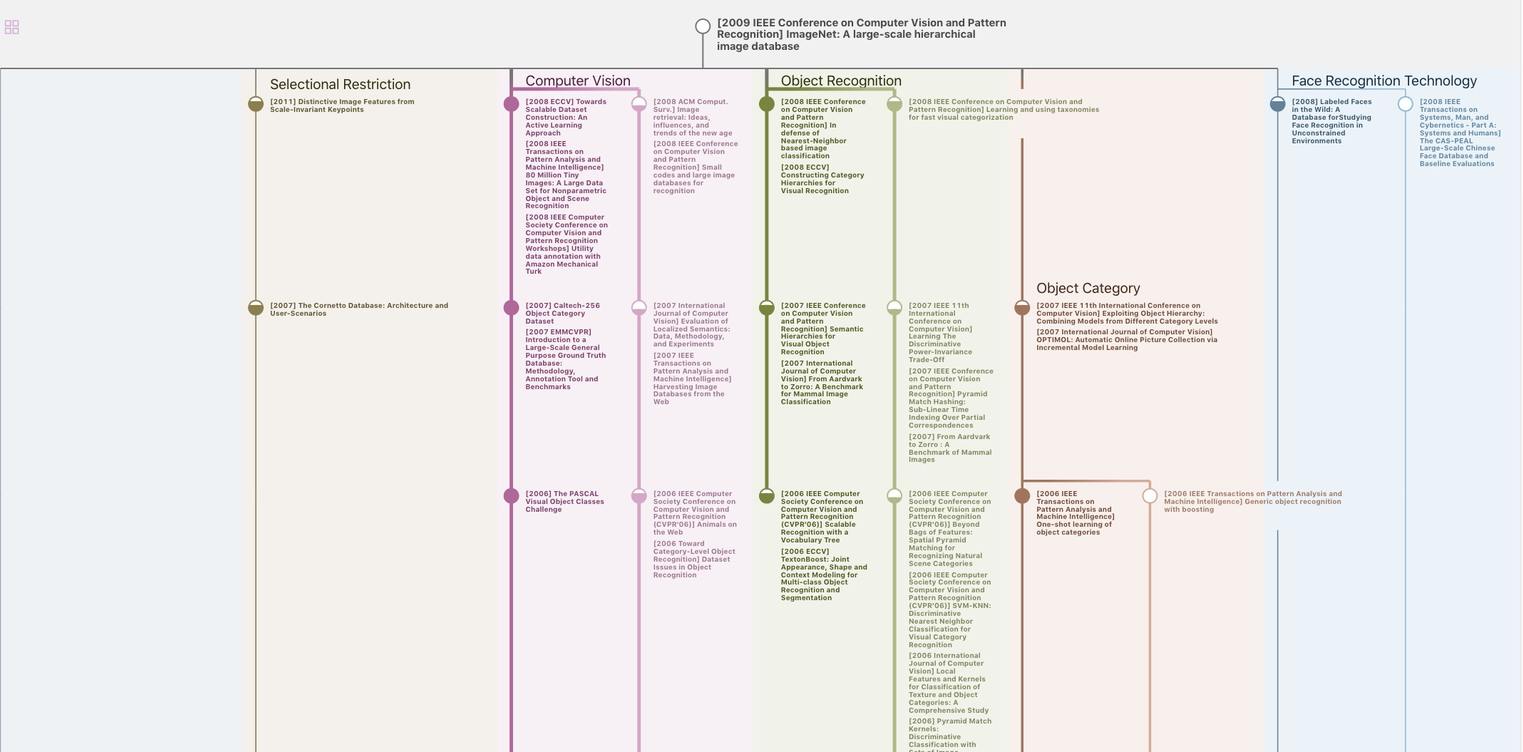
生成溯源树,研究论文发展脉络
Chat Paper
正在生成论文摘要