Hessian-Free Second-Order Adversarial Examples for Adversarial Learning
arxiv(2022)
摘要
Recent studies show deep neural networks (DNNs) are extremely vulnerable to the elaborately designed adversarial examples. Adversarial learning with those adversarial examples has been proved as one of the most effective methods to defend against such an attack. At present, most existing adversarial examples generation methods are based on first-order gradients, which can hardly further improve models' robustness, especially when facing second-order adversarial attacks. Compared with first-order gradients, second-order gradients provide a more accurate approximation of the loss landscape with respect to natural examples. Inspired by this, our work crafts second-order adversarial examples and uses them to train DNNs. Nevertheless, second-order optimization involves time-consuming calculation for Hessian-inverse. We propose an approximation method through transforming the problem into an optimization in the Krylov subspace, which remarkably reduce the computational complexity to speed up the training procedure. Extensive experiments conducted on the MINIST and CIFAR-10 datasets show that our adversarial learning with second-order adversarial examples outperforms other fisrt-order methods, which can improve the model robustness against a wide range of attacks.
更多查看译文
关键词
examples,learning,hessian-free,second-order
AI 理解论文
溯源树
样例
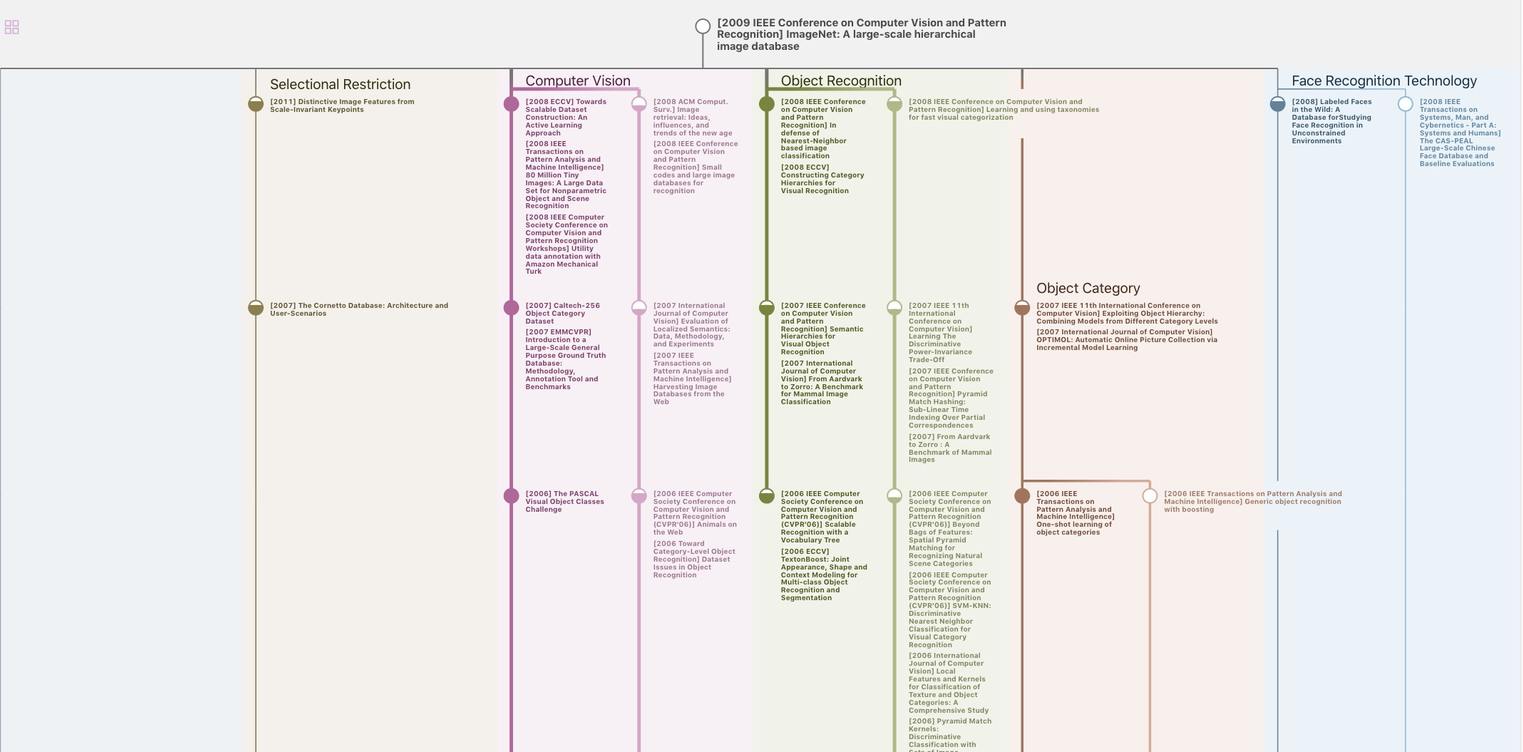
生成溯源树,研究论文发展脉络
Chat Paper
正在生成论文摘要