Assessing the Performance of Automated Prediction and Ranking of Patient Age from Chest X-rays Against Clinicians
MEDICAL IMAGE COMPUTING AND COMPUTER ASSISTED INTERVENTION, MICCAI 2022, PT VII(2022)
摘要
Understanding the internal physiological changes accompanying the aging process is an important aspect of medical image interpretation, with the expected changes acting as a baseline when reporting abnormal findings. Deep learning has recently been demonstrated to allow the accurate estimation of patient age from chest X-rays, and shows potential as a health indicator and mortality predictor. In this paper we present a novel comparative study of the relative performance of radiologists versus state-of-the-art deep learning models on two tasks: (a) patient age estimation from a single chest X-ray, and (b) ranking of two time-separated images of the same patient by age. We train our models with a heterogeneous database of 1.8M chest X-rays with ground truth patient ages and investigate the limitations on model accuracy imposed by limited training data and image resolution, and demonstrate generalisation performance on public data. To explore the large performance gap between the models and humans on these age-prediction tasks compared with other radiological reporting tasks seen in the literature, we incorporate our age prediction model into a conditional Generative Adversarial Network (cGAN) allowing visualisation of the semantic features identified by the prediction model as significant to age prediction, comparing the identified features with those relied on by clinicians.
更多查看译文
关键词
Chest X-rays, Age prediction, GAN, Deep learning
AI 理解论文
溯源树
样例
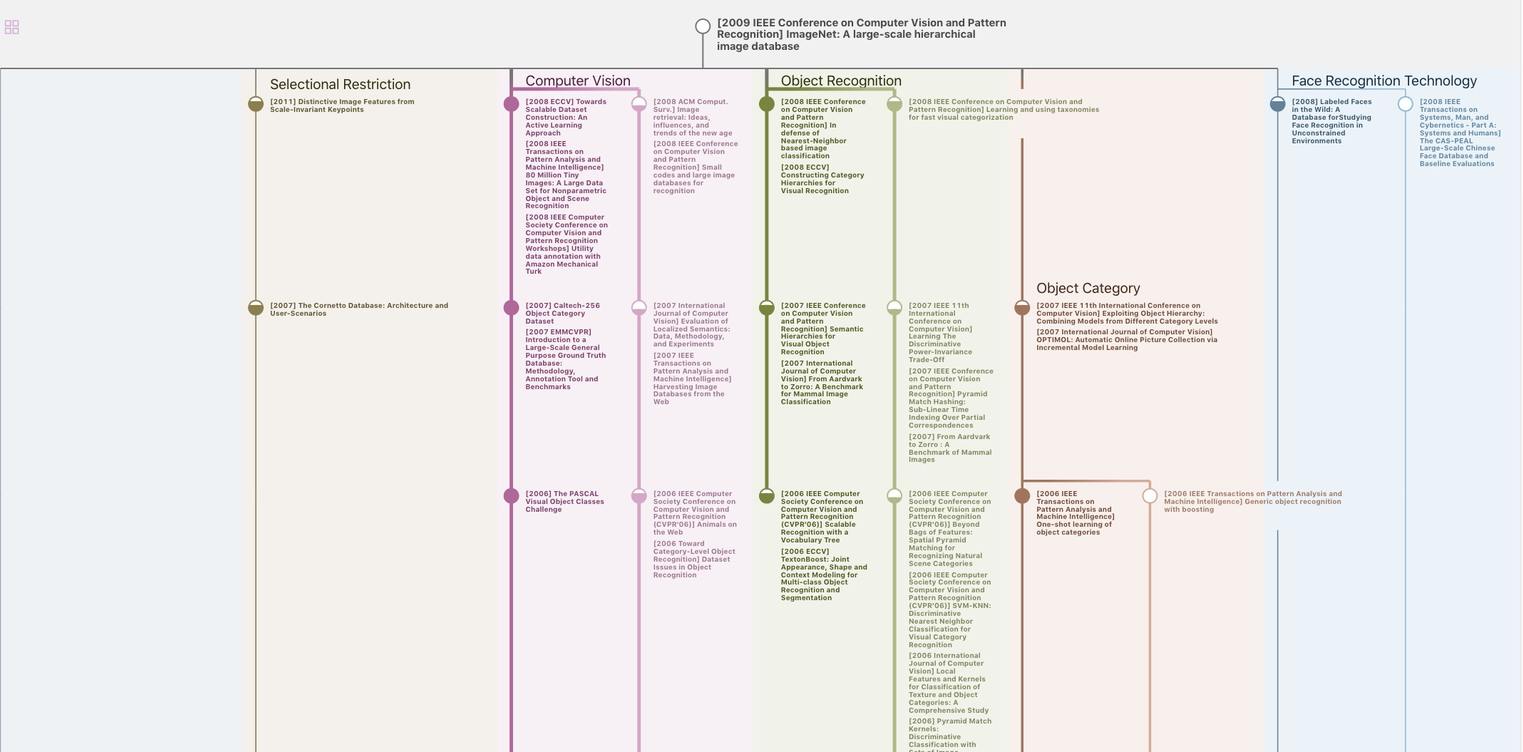
生成溯源树,研究论文发展脉络
Chat Paper
正在生成论文摘要