Sustainable AI Processing at the Edge
arxiv(2023)
摘要
Edge computing is a popular paradigm for accelerating light- to medium-weight machine learning algorithms initiated from mobile devices without requiring the long communication latencies to send them to remote datacenters in the cloud. Edge servers primarily consider traditional concerns, such as size, weight, and power constraints for their installations. However, such metrics are not entirely sufficient to consider environmental impacts from computing given the significant contributions from embodied energy and carbon. In this article we explore the tradeoffs of hardware strategies for convolutional neural network acceleration engines considering inference and online training. In particular, we explore the use of mobile graphics processing unit (GPU) accelerators, recently released edge-class field-programmable gate arrays, and novel processing in memory (PIM) using dynamic random-access memory (DRAM) and emerging Racetrack memory. Given edge servers already employ DRAM and sometimes GPU accelerators, we consider the sustainability implications using breakeven analysis of replacing or augmenting DDR3 with Racetrack memory. We also consider the implications for provisioning edge servers with different accelerators using indifference analysis. While mobile GPUs are typically much more energy efficient, their significant embodied energy can make them less sustainable than PIM solutions in certain scenarios that consider activity time and compute effort.
更多查看译文
关键词
Artificial intelligence,Edge computing,Memory management,Sustainable development,Measurement,Fabrication,Costs
AI 理解论文
溯源树
样例
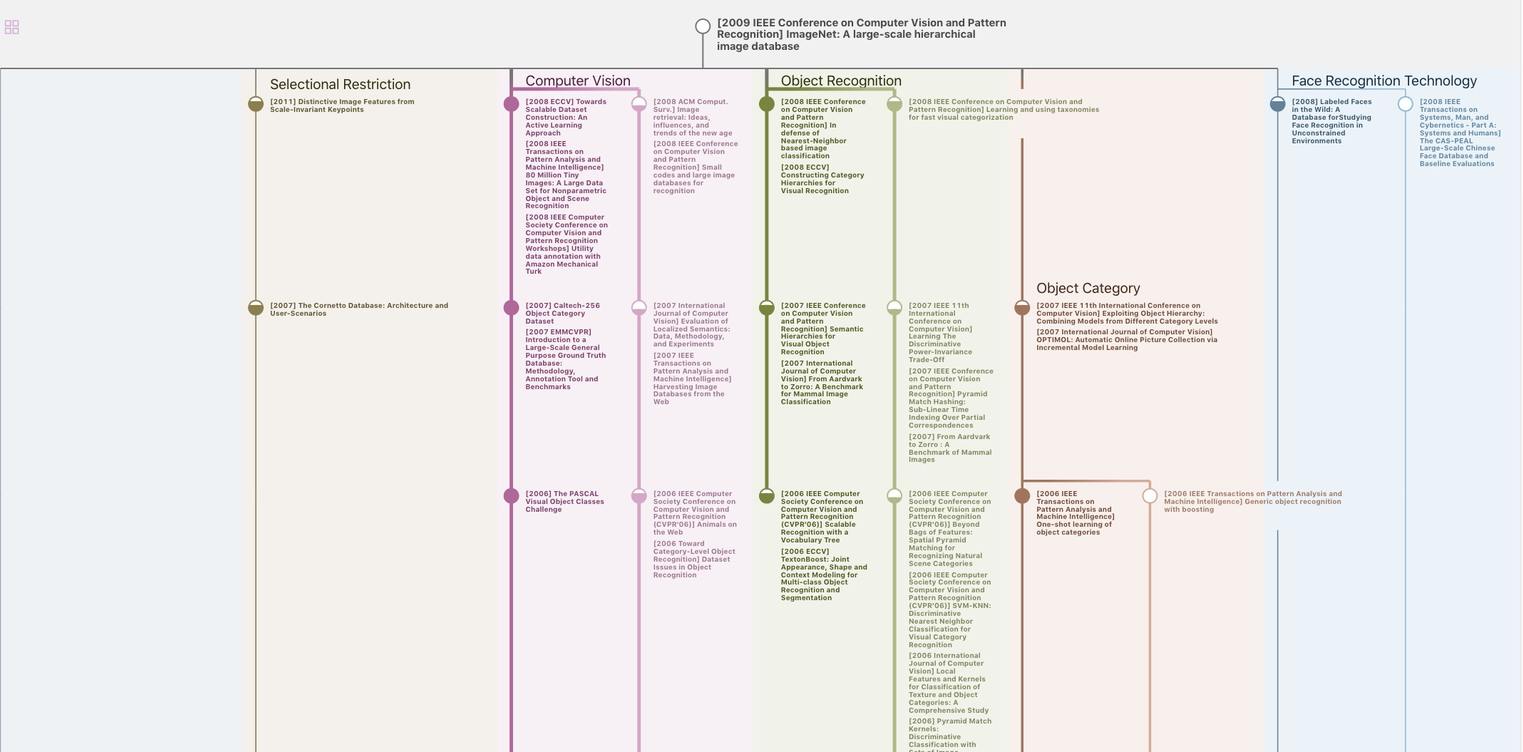
生成溯源树,研究论文发展脉络
Chat Paper
正在生成论文摘要