Facial Image Reconstruction from Functional Magnetic Resonance Imaging via GAN Inversion with Improved Attribute Consistency
2022 International Joint Conference on Neural Networks (IJCNN)(2022)
摘要
Neuroscience studies have revealed that the brain encodes visual content and embeds information in neural activity. Recently, deep learning techniques have facilitated attempts to address visual reconstructions by mapping brain activity to image stimuli using generative adversarial networks (GANs). However, none of these studies have considered the semantic meaning of latent code in image space. Omitting semantic information could potentially limit the performance. In this study, we propose a new framework to reconstruct facial images from functional Magnetic Resonance Imaging (fMRI) data. With this framework, the GAN inversion is first applied to train an image encoder to extract latent codes in image space, which are then bridged to fMRI data using linear transformation. Following the attributes identified from fMRI data using an attribute classifier, the direction in which to manipulate attributes is decided and the attribute manipulator adjusts the latent code to improve the consistency between the seen image and the reconstructed image. Our experimental results suggest that the proposed framework accomplishes two goals: (1) reconstructing clear facial images from fMRI data and (2) maintaining the consistency of semantic characteristics.
更多查看译文
关键词
fMRI,facial image reconstruction,GAN inversion,attribute manipulation
AI 理解论文
溯源树
样例
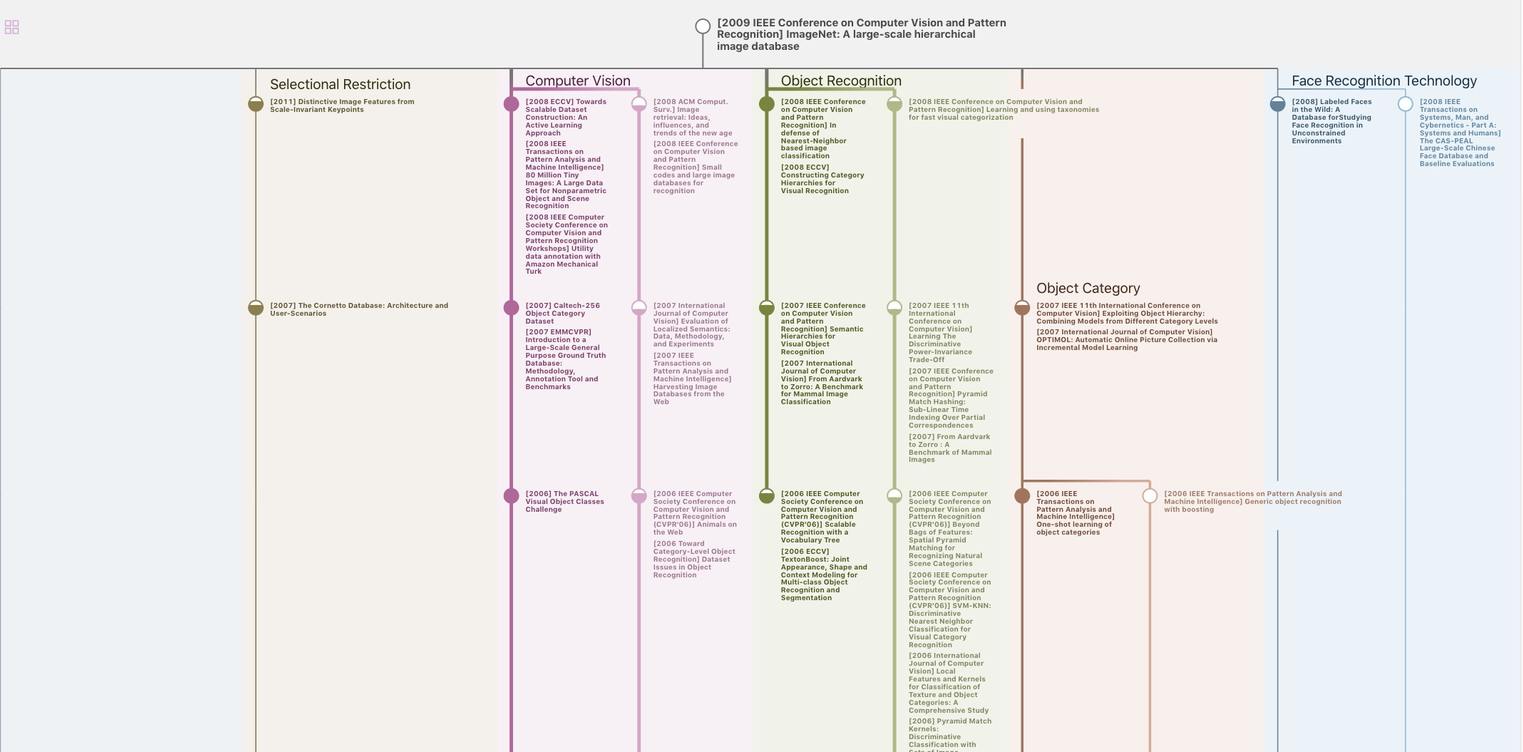
生成溯源树,研究论文发展脉络
Chat Paper
正在生成论文摘要