Interpretable by Design: Learning Predictors by Composing Interpretable Queries.
IEEE transactions on pattern analysis and machine intelligence(2022)
摘要
There is a growing concern about typically opaque decision-making with high-performance machine learning algorithms. Providing an explanation of the reasoning process in domain-specific terms can be crucial for adoption in risk-sensitive domains such as healthcare. We argue that machine learning algorithms should be interpretable by design and that the language in which these interpretations are expressed should be domain- and task-dependent. Consequently, we base our model's prediction on a family of user-defined and task-specific binary functions of the data, each having a clear interpretation to the end-user. We then minimize the expected number of queries needed for accurate prediction on any given input. As the solution is generally intractable, following prior work, we choose the queries sequentially based on information gain. However, in contrast to previous work, we need not assume the queries are conditionally independent. Instead, we leverage a stochastic generative model (VAE) and an MCMC algorithm (Unadjusted Langevin) to select the most informative query about the input based on previous query-answers. This enables the online determination of a query chain of whatever depth is required to resolve prediction ambiguities. Finally, experiments on vision and NLP tasks demonstrate the efficacy of our approach and its superiority over post-hoc explanations.
更多查看译文
关键词
Explainable AI,interpretable ML,computer vision,generative models,information theory
AI 理解论文
溯源树
样例
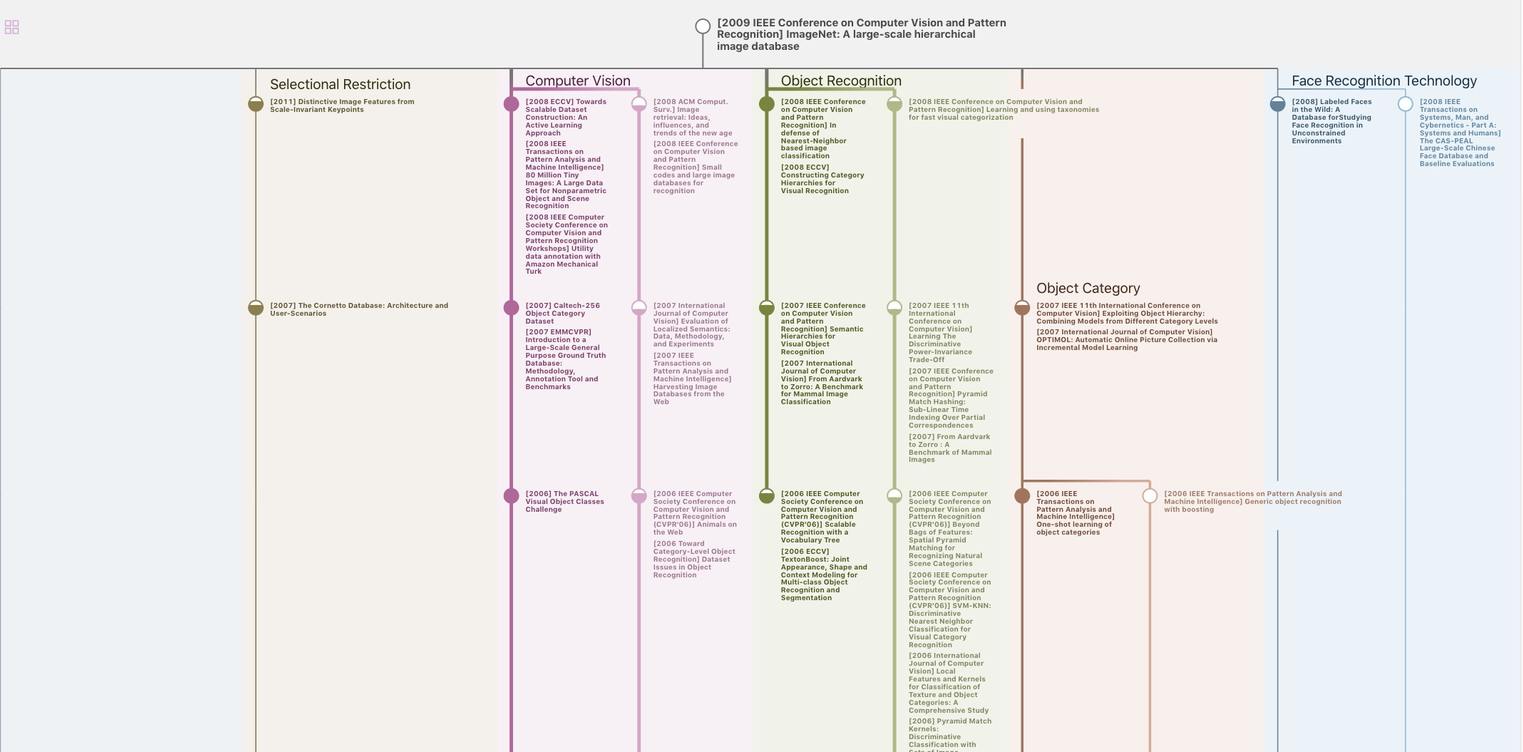
生成溯源树,研究论文发展脉络
Chat Paper
正在生成论文摘要