Few-shot incremental learning in the context of solar cell quality inspection
arXiv (Cornell University)(2022)
摘要
In industry, Deep Neural Networks have shown high defect detection rates surpassing other more traditional manual feature engineering based proposals. This has been achieved mainly through supervised training where a great amount of data is required in order to learn good classification models. However, such amount of data is sometimes hard to obtain in industrial scenarios, as few defective pieces are produced normally. In addition, certain kinds of defects are very rare and usually just appear from time to time, which makes the generation of a proper dataset for training a classification model even harder. Moreover, the lack of available data limits the adaptation of inspection models to new defect types that appear in production as it might require a model retraining in order to incorporate the detects and detect them. In this work, we have explored the technique of weight imprinting in the context of solar cell quality inspection where we have trained a network on three base defect classes, and then we have incorporated new defect classes using few samples. The results have shown that this technique allows the network to extend its knowledge with regard to defect classes with few samples, which can be interesting for industrial practitioners.
更多查看译文
关键词
solar cell quality
AI 理解论文
溯源树
样例
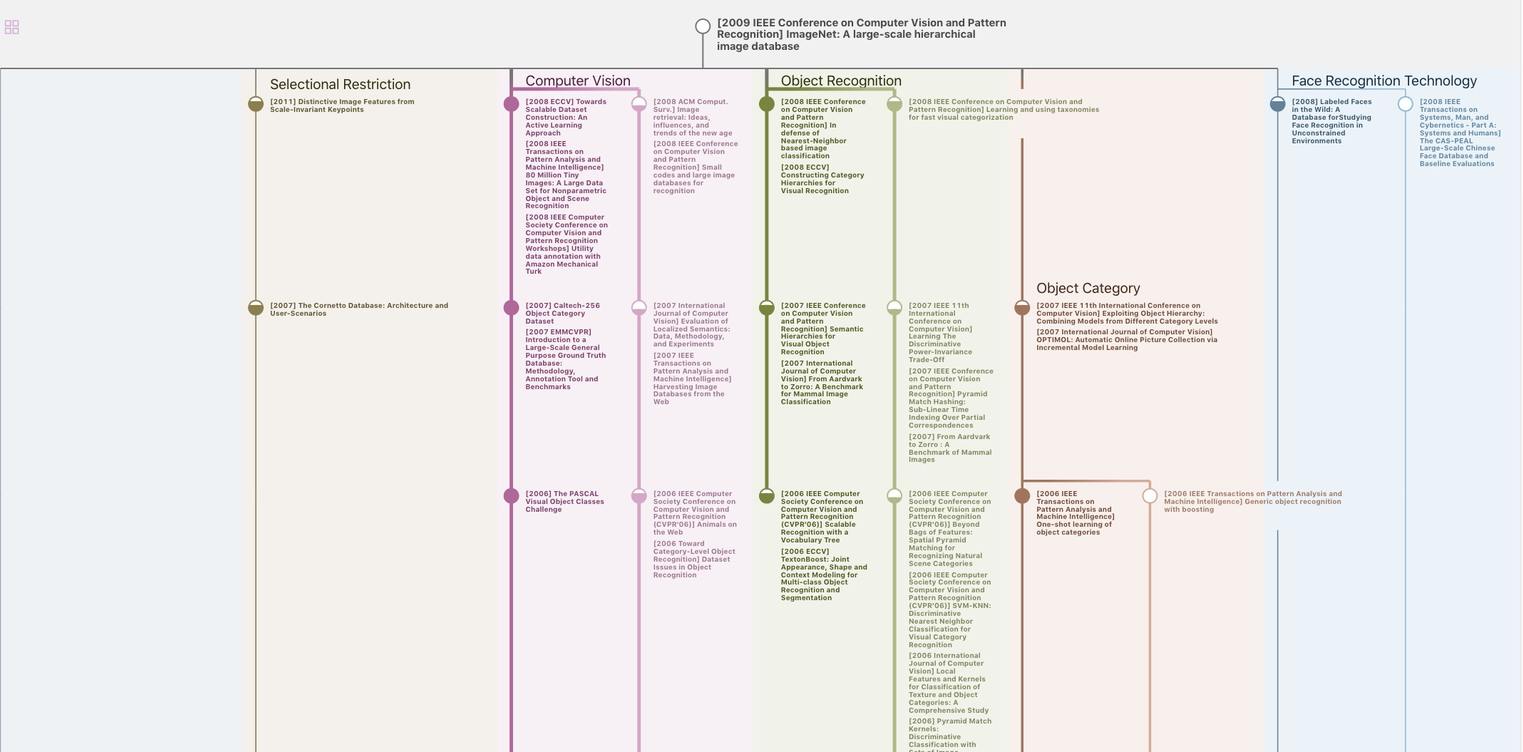
生成溯源树,研究论文发展脉络
Chat Paper
正在生成论文摘要