FSE Compensated Motion Correction for MRI Using Data Driven Methods
MEDICAL IMAGE COMPUTING AND COMPUTER ASSISTED INTERVENTION, MICCAI 2022, PT VI(2022)
摘要
Magnetic Resonance Imaging (MRI) is a widely used medical imaging modality boasting great soft tissue contrast without ionizing radiation, but unfortunately suffers from long acquisition times. Long scan times can lead to motion artifacts, for example due to bulk patient motion such as head movement and periodic motion produced by the heart or lungs. Motion artifacts can degrade image quality and in some cases render the scans nondiagnostic. To combat this problem, prospective and retrospective motion correction techniques have been introduced. More recently, data driven methods using deep neural networks have been proposed. As a large number of publicly available MRI datasets are based on Fast Spin Echo (FSE) sequences, methods that use them for training should incorporate the correct FSE acquisition dynamics. Unfortunately, when simulating training data, many approaches fail to generate accurate motion-corrupt images by neglecting the effects of the temporal ordering of the k-space lines as well as neglecting the signal decay throughout the FSE echo train. In this work, we highlight this consequence and demonstrate a training method which correctly simulates the data acquisition process of FSE sequences with higher fidelity by including sample ordering and signal decay dynamics. Through numerical experiments, we show that accounting for the FSE acquisition leads to better motion correction performance during inference.
更多查看译文
关键词
Motion correction, Deep learning, Magnetic resonance imaging, Fast spin echo
AI 理解论文
溯源树
样例
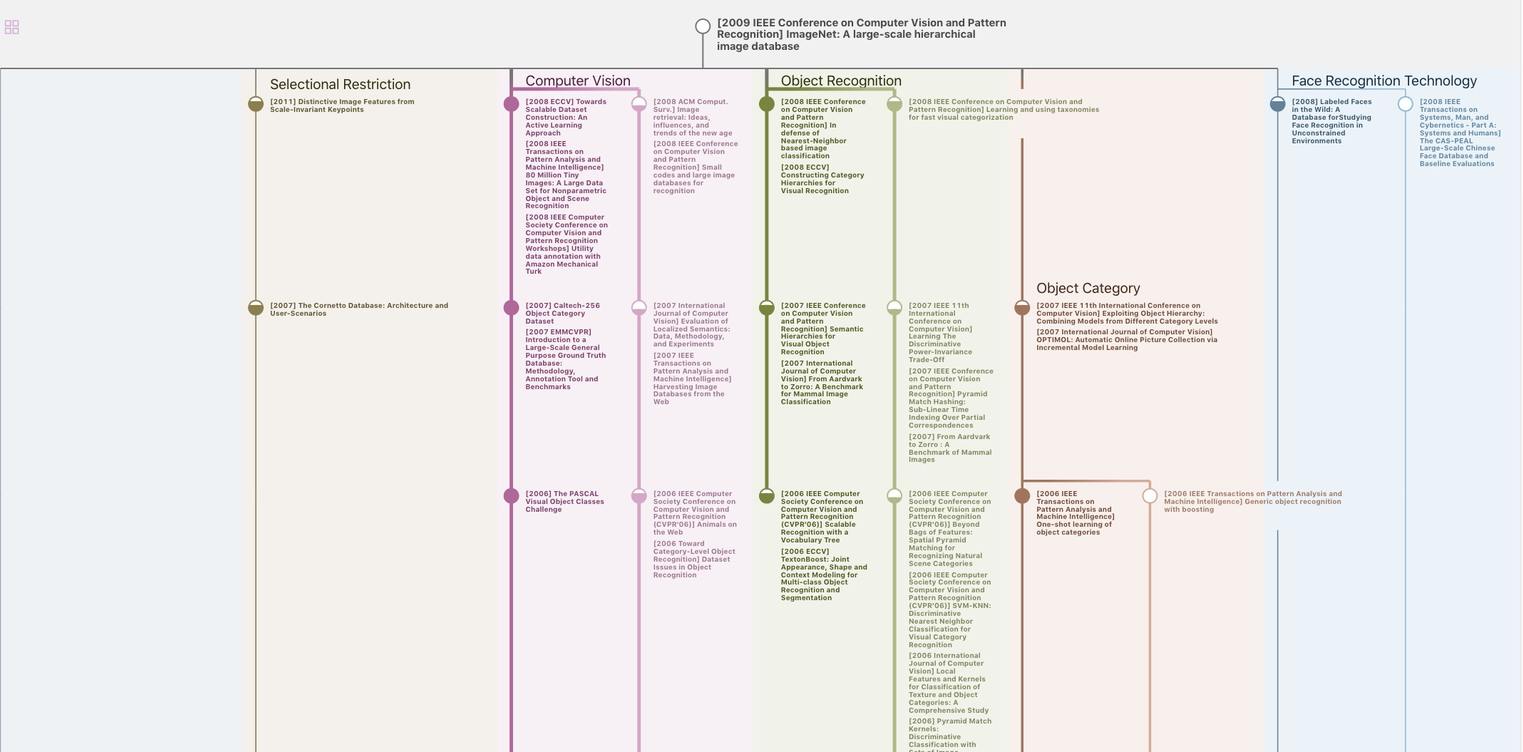
生成溯源树,研究论文发展脉络
Chat Paper
正在生成论文摘要