Unsupervised Cross-Domain Feature Extraction for Single Blood Cell Image Classification
Medical Image Computing and Computer Assisted Intervention – MICCAI 2022(2022)
摘要
Diagnosing hematological malignancies requires identification and classification of white blood cells in peripheral blood smears. Domain shifts caused by different lab procedures, staining, illumination, and microscope settings hamper the re-usability of recently developed machine learning methods on data collected from different sites. Here, we propose a cross-domain adapted autoencoder to extract features in an unsupervised manner on three different datasets of single white blood cells scanned from peripheral blood smears. The autoencoder is based on an R-CNN architecture allowing it to focus on the relevant white blood cell and eliminate artifacts in the image. To evaluate the quality of the extracted features we use a simple random forest to classify single cells. We show that thanks to the rich features extracted by the autoencoder trained on only one of the datasets, the random forest classifier performs satisfactorily on the unseen datasets, and outperforms published oracle networks in the cross-domain task. Our results suggest the possibility of employing this unsupervised approach in more complicated diagnosis and prognosis tasks without the need to add expensive expert labels to unseen data.
更多查看译文
关键词
Unsupervised learning, Feature extraction, Autoencoders, Single cell classification, Microscopy, Domain adaptation
AI 理解论文
溯源树
样例
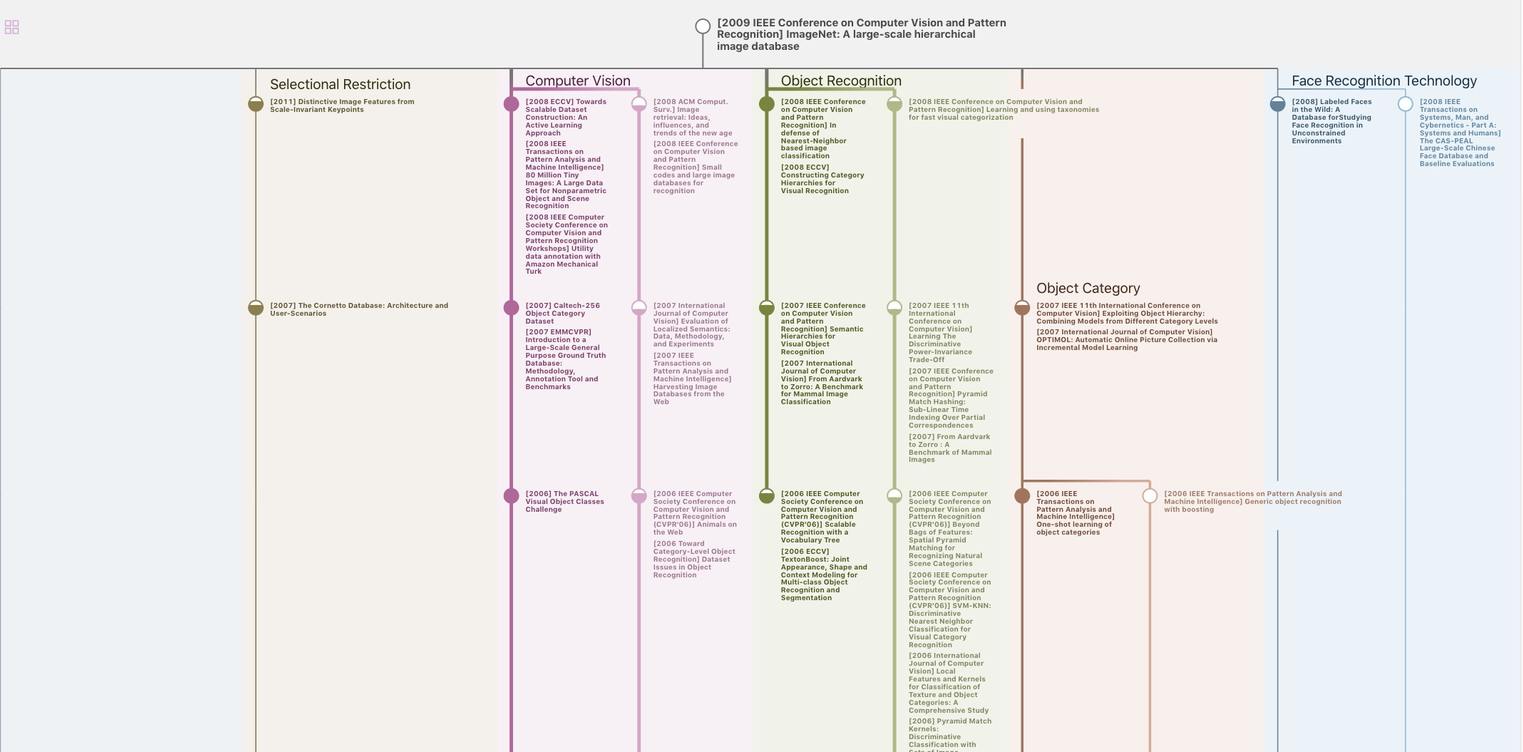
生成溯源树,研究论文发展脉络
Chat Paper
正在生成论文摘要