Usable Region Estimate for Assessing Practical Usability of Medical Image Segmentation Models
MEDICAL IMAGE COMPUTING AND COMPUTER ASSISTED INTERVENTION, MICCAI 2022, PT V(2022)
摘要
We aim to quantitatively measure the practical usability of medical image segmentation models: to what extent, how often, and on which samples a model's predictions can be used/trusted. We first propose a measure, Correctness-Confidence Rank Correlation (CCRC), to capture how predictions' confidence estimates correlate with their correctness scores in rank. A model with a high value of CCRC means its prediction confidences reliably suggest which samples' predictions are more likely to be correct. Since CCRC does not capture the actual prediction correctness, it alone is insufficient to indicate whether a prediction model is both accurate and reliable to use in practice. Therefore, we further propose another method, Usable Region Estimate (URE), which simultaneously quantifies predictions' correctness and reliability of confidence assessments in one estimate. URE provides concrete information on to what extent a model's predictions are usable. In addition, the sizes of usable regions (UR) can be utilized to compare models: A model with a larger UR can be taken as a more usable and hence better model. Experiments on six datasets validate that the proposed evaluation methods perform well, providing a concrete and concise measure for the practical usability of medical image segmentation models.
更多查看译文
关键词
Medical AI models, Model evaluation, Practical usability, Unified measure, Medical image segmentation
AI 理解论文
溯源树
样例
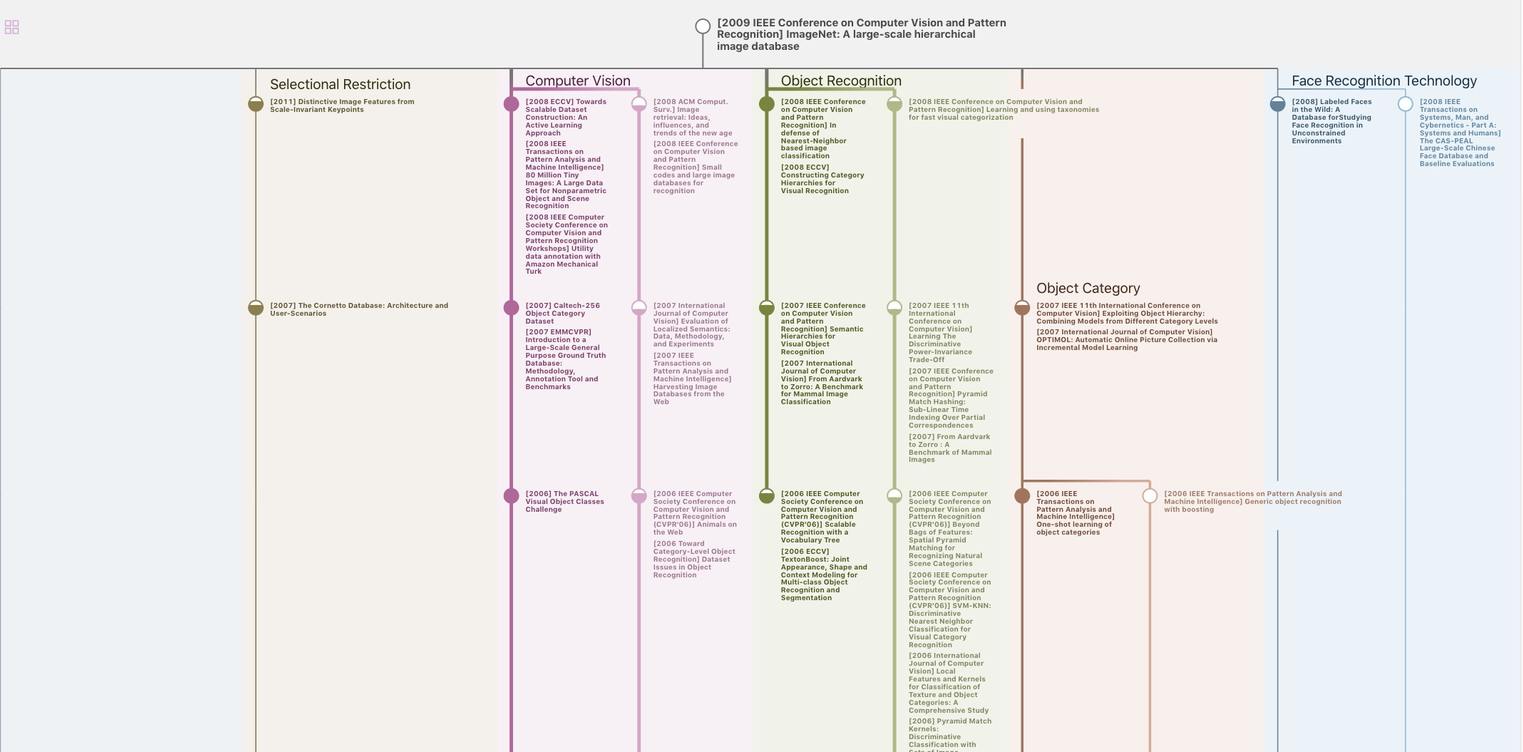
生成溯源树,研究论文发展脉络
Chat Paper
正在生成论文摘要