SNR-Aware Low-light Image Enhancement
IEEE Conference on Computer Vision and Pattern Recognition(2022)
摘要
This paper presents a new solution for low-light image enhancement by collectively exploiting Signal-to-Noise-Ratio-aware transformers and convolutional models to dynamically enhance pixels with spatial-varying operations. They are long-range operations for image regions of extremely low Signal-to-Noise-Ratio (SNR) and short-range operations for other regions. We propose to take an SNR prior to guide the feature fusion and formulate the SNR-aware transformer with a new self-attention model to avoid tokens from noisy image regions of very low SNR. Extensive experiments show that our framework consistently achieves better performance than SOTA approaches on seven representative benchmarks with the same structure. Also, we conducted a large-scale user study with 100 participants to verify the superior perceptual quality of our results. The code is available at https://github.com/dvlab-research/SNR-Aware-Low-Light-Enhance.
更多查看译文
关键词
Computational photography, Low-level vision
AI 理解论文
溯源树
样例
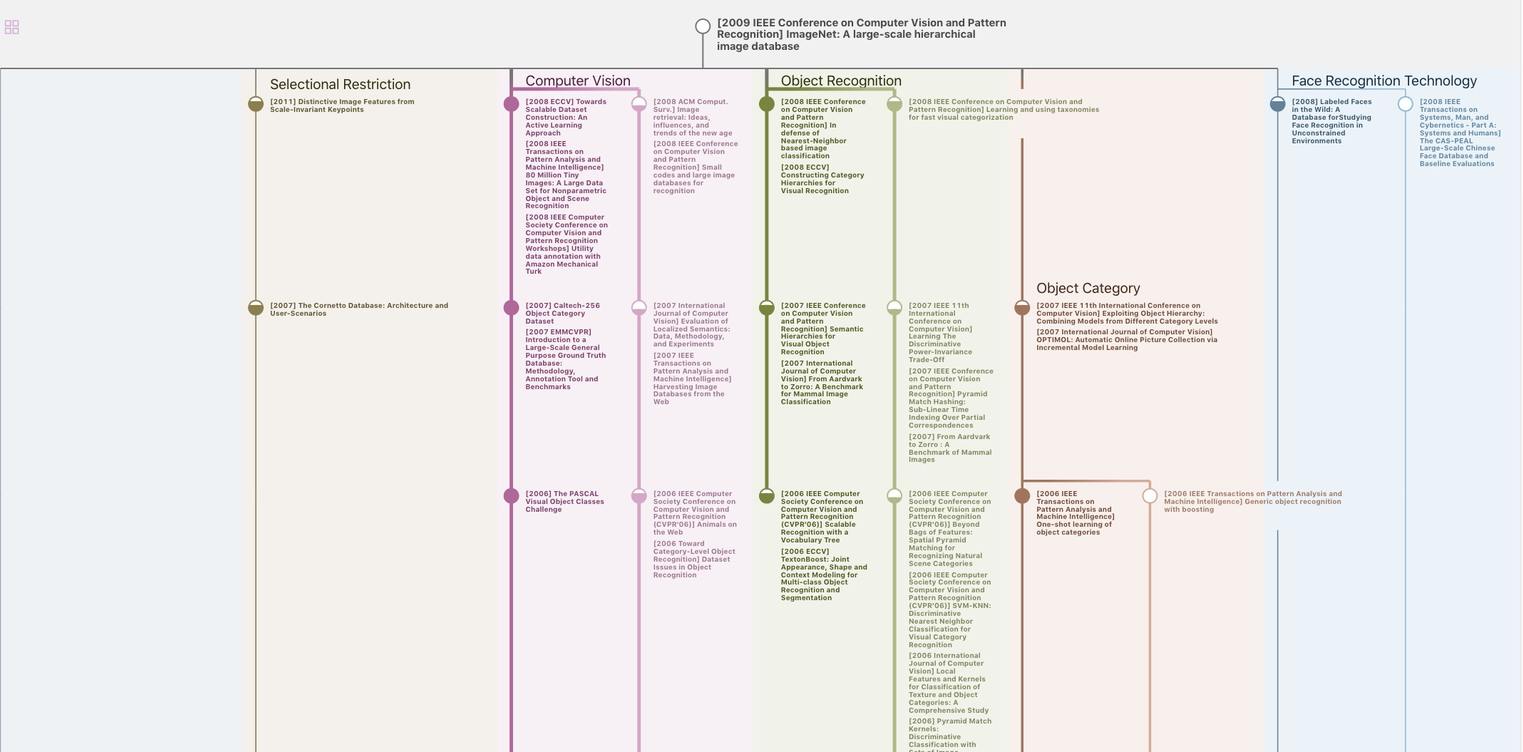
生成溯源树,研究论文发展脉络
Chat Paper
正在生成论文摘要