FAM: Visual Explanations for the Feature Representations from Deep Convolutional Networks.
IEEE Conference on Computer Vision and Pattern Recognition(2022)
摘要
In recent years, increasing attention has been drawn to the internal mechanisms of representation models. Traditional methods are inapplicable to fully explain the feature representations, especially if the images do not fit into any category. In this case, employing an existing class or the similarity with other image is unable to provide a complete and reliable visual explanation. To handle this task, we propose a novel visual explanation paradigm called Feature Activation Mapping (FAM) in this paper. Under this paradigm, Grad-FAM and Score-FAM are designed for visualizing feature representations. Unlike the previous approaches, FAM locates the regions of images that contribute most to the feature vector itself. Extensive experiments and evaluations, both subjective and objective, showed that Score-FAM provided most promising interpretable visual explanations for feature representations in Person Re-Identification. Furthermore, FAM also can be employed to analyze other vision tasks, such as self-supervised representation learning.
更多查看译文
关键词
privacy and ethics in vision,accountability,fairness,retrieval, Representation learning, Transparency,categorization,Explainable computer vision, Recognition: detection
AI 理解论文
溯源树
样例
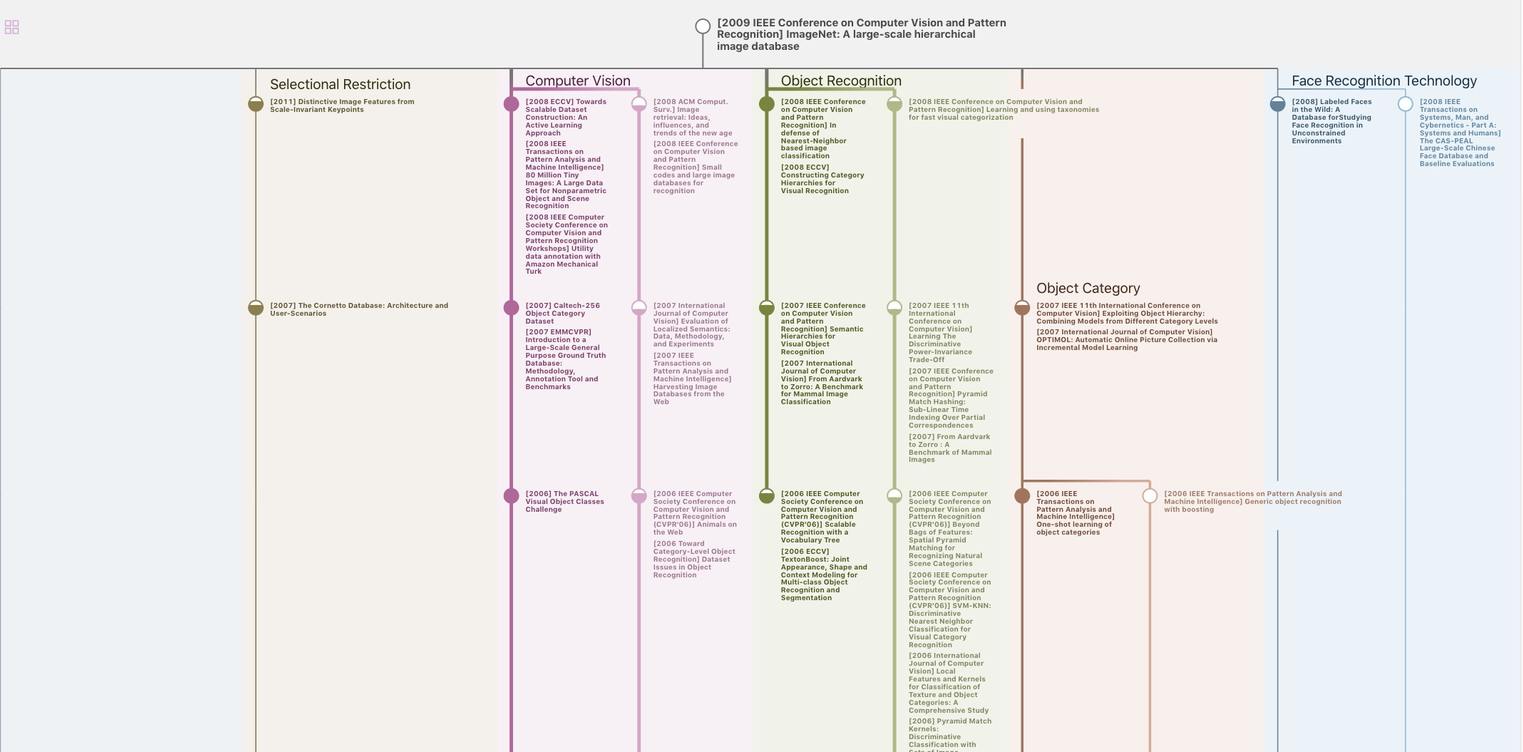
生成溯源树,研究论文发展脉络
Chat Paper
正在生成论文摘要