Dynamic Scene Graph Generation via Anticipatory Pre-training
IEEE Conference on Computer Vision and Pattern Recognition(2022)
摘要
Humans can not only see the collection of objects in visual scenes, but also identify the relationship between objects. The visual relationship in the scene can be abstracted into the semantic representation of a triple (subject, predicate, object) and thus results in a scene graph, which can convey a lot of information for visual understanding. Due to the motion of objects, the visual relationship between two objects in videos may vary, which makes the task of dynamically generating scene graphs from videos more complicated and challenging than the conventional image-based static scene graph generation. Inspired by the ability of humans to infer the visual relationship, we propose a novel anticipatory pre-training paradigm based on Transformer to explicitly model the temporal correlation of visual relationships in different frames to improve dynamic scene graph generation. In pre-training stage, the model predicts the visual relationships of current frame based on the previous frames by extracting intra-frame spatial information with a spatial encoder and inter-frame temporal correlations with a progressive temporal encoder. In the fine-tuning stage, we reuse the spatial encoder and the progressive temporal encoder while the information of the current frame is combined for predicting the visual relationship. Extensive experiments demonstrate that our method achieves state-of-the-art performance on Action Genome dataset.
更多查看译文
关键词
Video analysis and understanding, Scene analysis and understanding, Visual reasoning
AI 理解论文
溯源树
样例
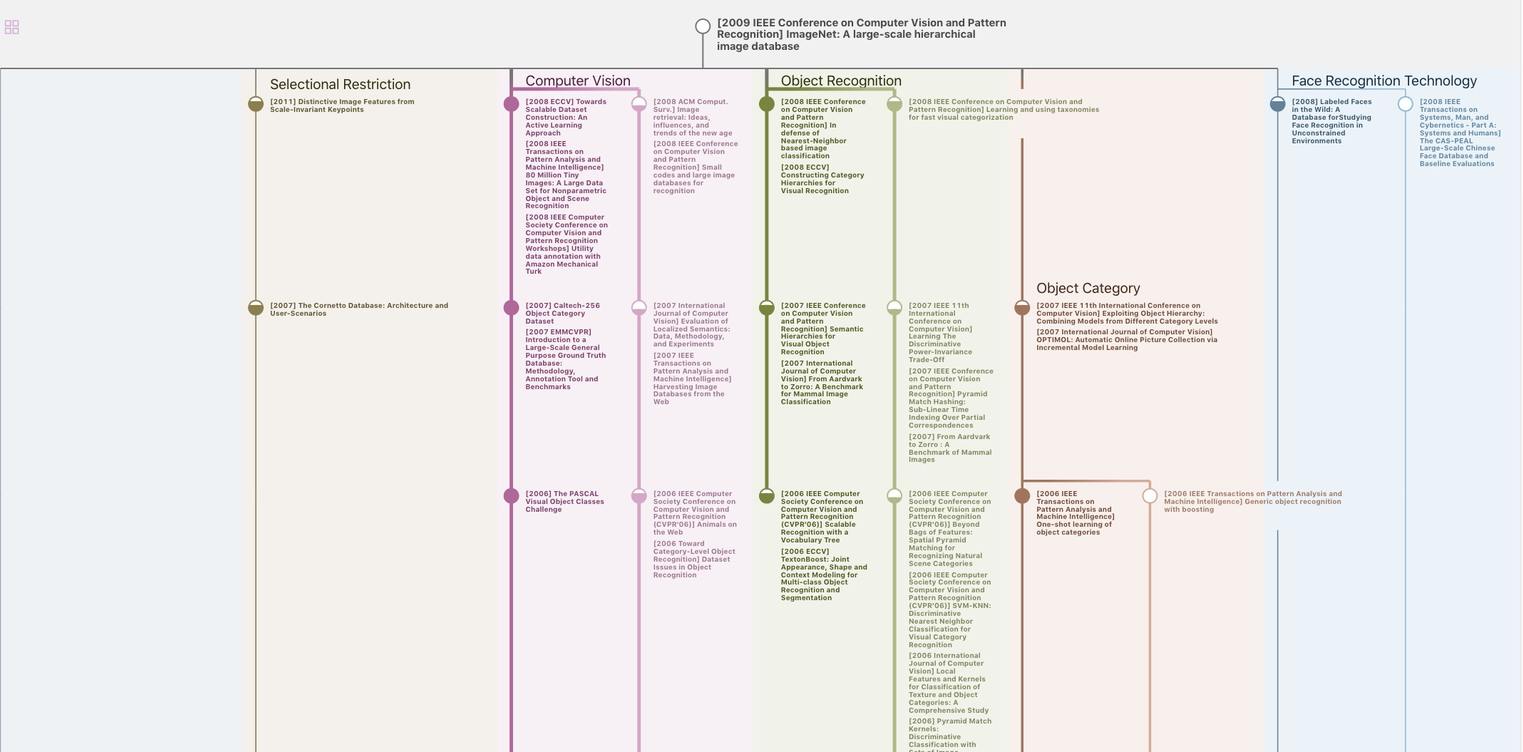
生成溯源树,研究论文发展脉络
Chat Paper
正在生成论文摘要