URetinex-Net: Retinex-based Deep Unfolding Network for Low-light Image Enhancement
IEEE Conference on Computer Vision and Pattern Recognition(2022)
摘要
Retinex model-based methods have shown to be effective in layer-wise manipulation with well-designed priors for low-light image enhancement. However, the commonly used handcrafted priors and optimization-driven solutions lead to the absence of adaptivity and efficiency. To address these issues, in this paper, we propose a Retinex-based deep unfolding network (URetinex-Net), which unfolds an optimization problem into a learnable network to decompose a low-light image into reflectance and illumination layers. By formulating the decomposition problem as an implicit priors regularized model, three learning-based modules are carefully designed, responsible for data-dependent initialization, high-efficient unfolding optimization, and user-specified illumination enhancement, respectively. Particularly, the proposed unfolding optimization module, introducing two networks to adaptively fit implicit priors in data-driven manner, can realize noise suppression and details preservation for the final decomposition results. Extensive experiments on real-world low-light images qualitatively and quantitatively demonstrate the effectiveness and superiority of the proposed method over state-of-the-art methods. The code is available at https://github.com/AndersonYong/URetinex-Net.
更多查看译文
关键词
Low-level vision
AI 理解论文
溯源树
样例
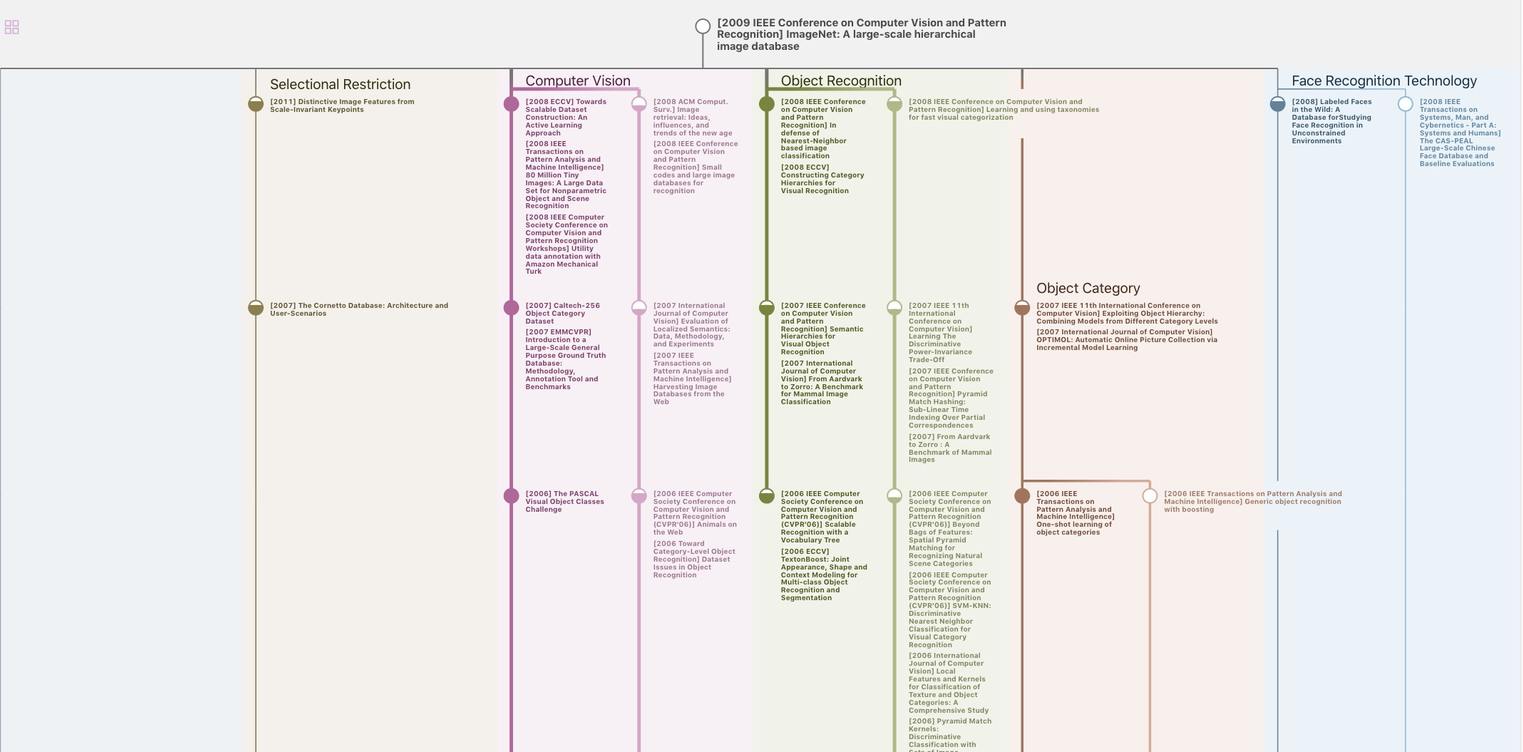
生成溯源树,研究论文发展脉络
Chat Paper
正在生成论文摘要