DIFNet: Boosting Visual Information Flow for Image Captioning
IEEE Conference on Computer Vision and Pattern Recognition(2022)
摘要
Current Image Captioning (IC) methods predict textual words sequentially based on the input visual information from the visual feature extractor and the partially generated sentence information. However, for most cases, the partially generated sentence may dominate the target word prediction due to the insufficiency of visual information, making the generated descriptions irrelevant to the content of the given image. In this paper, we propose a Dual Information Flow Network (DIFNet 1 1 Source code is available at: https://github.com/mrwu-mac/DIFNet) to address this issue, which takes segmentation feature as another visual information source to enhance the contribution of visual information for prediction. To maximize the use of two information flows, we also propose an effective feature fusion module termed Iterative Independent Layer Normalization (IILN) which can condense the most relevant inputs while retraining modality-specific information in each flow. Experiments show that our method is able to enhance the dependence of prediction on visual information, making word prediction more focused on the visual content, and thus achieves new state-of-the-art performance on the MSCOCO dataset, e.g., 136.2 CIDEr on COCO Karpathy test split.
更多查看译文
关键词
Vision + language
AI 理解论文
溯源树
样例
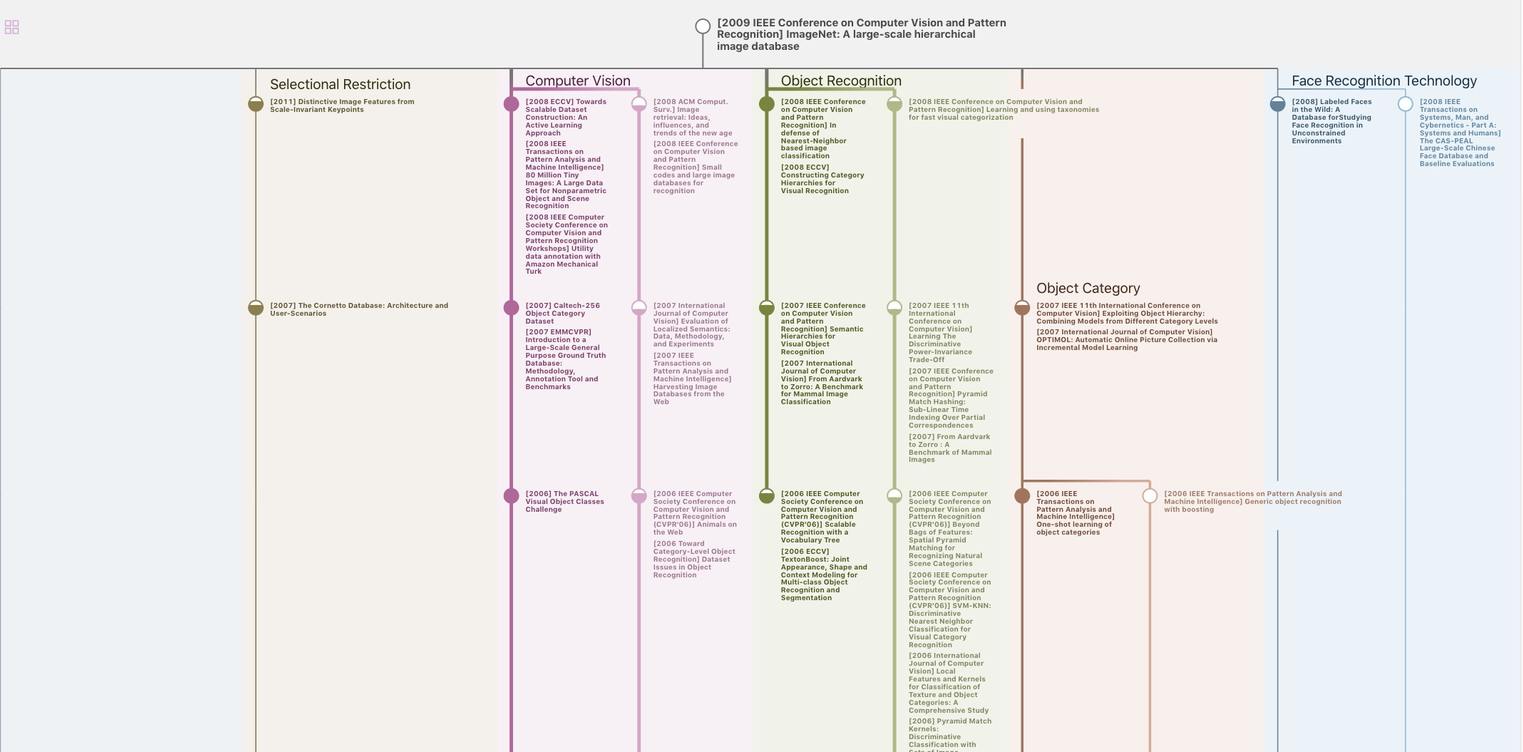
生成溯源树,研究论文发展脉络
Chat Paper
正在生成论文摘要