Revisiting AP Loss for Dense Object Detection: Adaptive Ranking Pair Selection
IEEE Conference on Computer Vision and Pattern Recognition(2022)
摘要
Average precision (AP) loss has recently shown promising performance on the dense object detection task. However, a deep understanding of how AP loss affects the detector from a pairwise ranking perspective has not yet been developed. In this work, we revisit the average precision (AP) loss and reveal that the crucial element is that of selecting the ranking pairs between positive and negative samples. Based on this observation, we propose two strategies to improve the AP loss. The first of these is a novel Adaptive Pairwise Error (APE) loss that focusing on ranking pairs in both positive and negative samples. Moreover, we select more accurate ranking pairs by exploiting the normalized ranking scores and localization scores with a clustering algorithm. Experiments conducted on the MSCOCO dataset support our analysis and demonstrate the superiority of our proposed method compared with current classification and ranking loss. The code is available at https://github.com/Xudangliatiger/APE-Loss.
更多查看译文
关键词
Recognition: detection,categorization,retrieval, Vision applications and systems
AI 理解论文
溯源树
样例
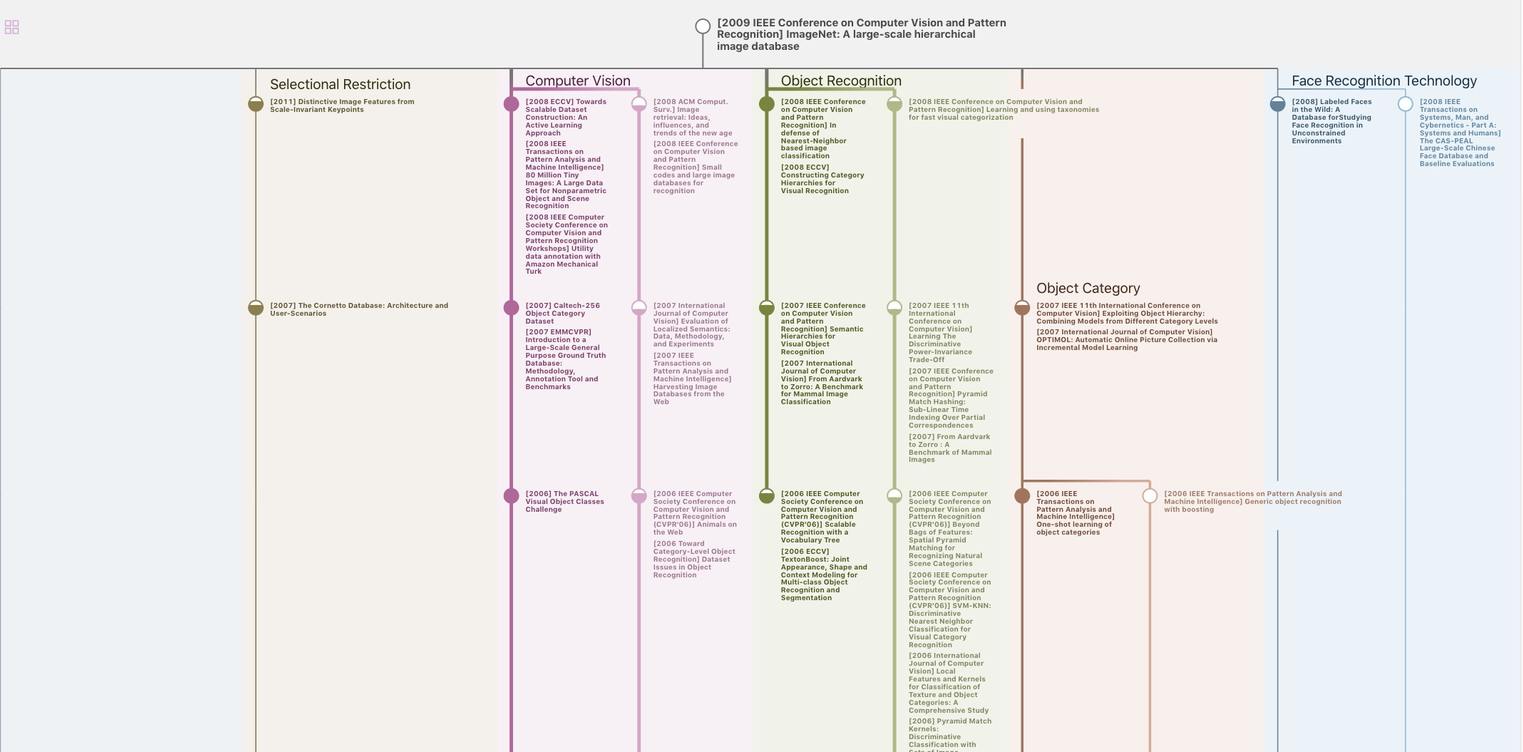
生成溯源树,研究论文发展脉络
Chat Paper
正在生成论文摘要