Rethinking Deep Face Restoration
IEEE Conference on Computer Vision and Pattern Recognition(2022)
摘要
A model that can authentically restore a low-quality face image to a high-quality one can benefit many applications. While existing approaches for face restoration make significant progress in generating high-quality faces, they often fail to preserve facial features that compromise the authenticity of reconstructed faces. Because the human visual system is very sensitive to faces, even minor changes may significantly degrade the perceptual quality. In this work, we argue that the problems of existing models can be traced down to the two sub-tasks of the face restoration problem, i.e. face generation and face reconstruction, and the fragile balance between them. Based on the observation, we propose a new face restoration model that improves both generation and reconstruction. Besides the model improvement, we also introduce a new evaluation metric for measuring models' ability to preserve the identity in the restored faces. Extensive experiments demonstrate that our model achieves state-of-the-art performance on multiple face restoration benchmarks, and the proposed metric has a higher correlation with user preference. The user study shows that our model produces higher quality faces while better preserving the identity 86.4% of the time compared with state-of-the-art methods.
更多查看译文
关键词
Image and video synthesis and generation, Face and gestures
AI 理解论文
溯源树
样例
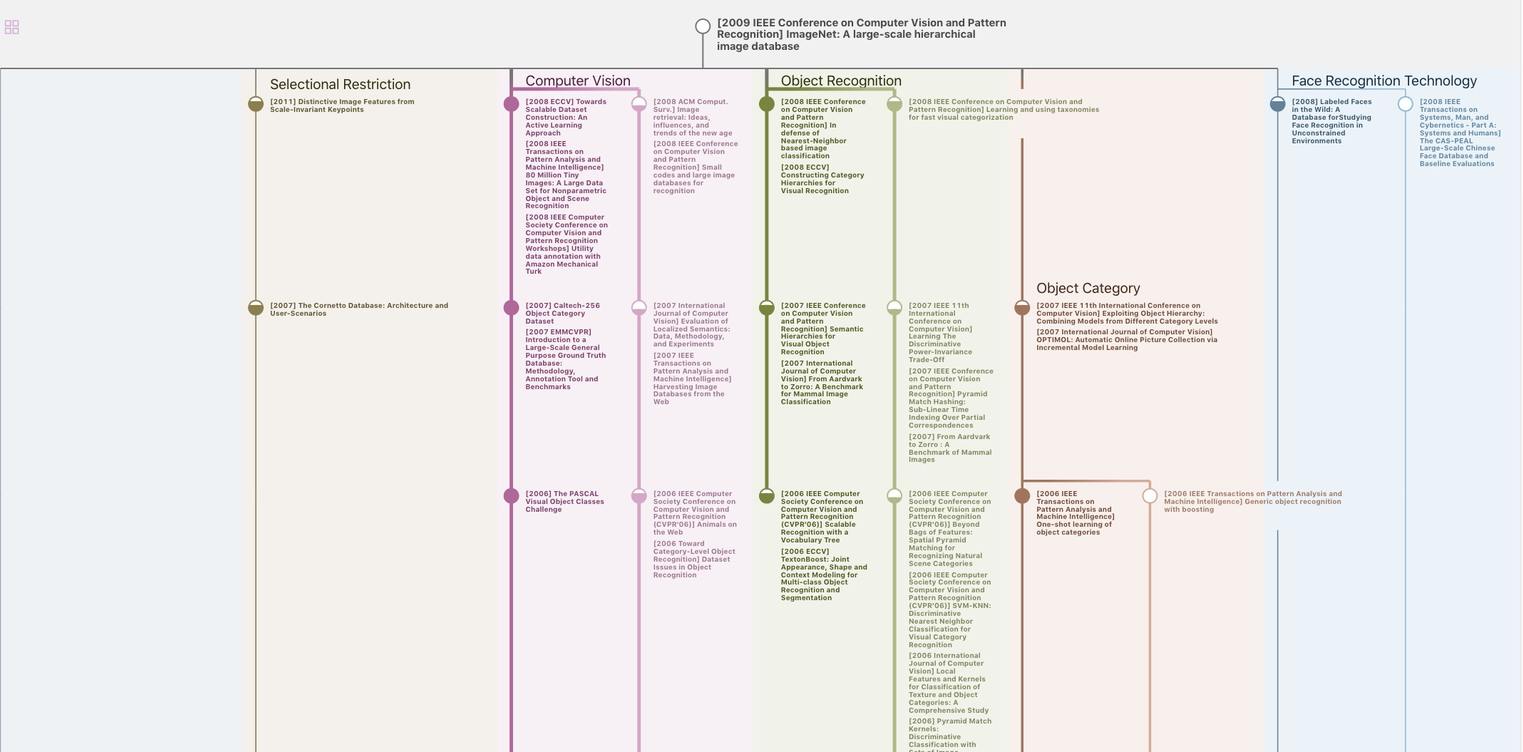
生成溯源树,研究论文发展脉络
Chat Paper
正在生成论文摘要